Reflectance edge guided networks for detail-preserving intrinsic image decomposition
Science China Information Sciences(2023)
摘要
Deep learning-based intrinsic image decomposition methods rely heavily on large-scale training data. However, current real-world datasets only contain sparse annotations, leading to textureless reflectance estimation. Although densely-labeled synthetic datasets are available, the large bias between these two categories easily incurs noticeable artifacts (e.g., shading residuals) on reflectance. To address this issue, we introduce reflectance edges that are predicted by a neural network trained on synthetic data with full supervision. Once trained, this network is able to capture high-frequency details of reflectance while greatly reducing the bias stemming from the discrepancy between different data distributions. We design another neural network to remove shading as much as possible from the input image. As this network is trained solely on real-world datasets, little bias will be introduced but the predicted reflectance will be overly smooth due to limited annotations. To recover texture details of the reflectance while still suppressing bias, we leverage a third neural network to progressively fuse feature maps from both reflectance edge maps and coarse-grained reflectance maps. The well-designed fusion strategy makes the best use of features extracted from the real-world data and helps to generate texture-rich reflectance with fewer artifacts. Extensive experiments on multiple benchmark datasets demonstrate the superiority of the proposed method.
更多查看译文
关键词
intrinsic image decomposition,detail-preserving,reflectance edges
AI 理解论文
溯源树
样例
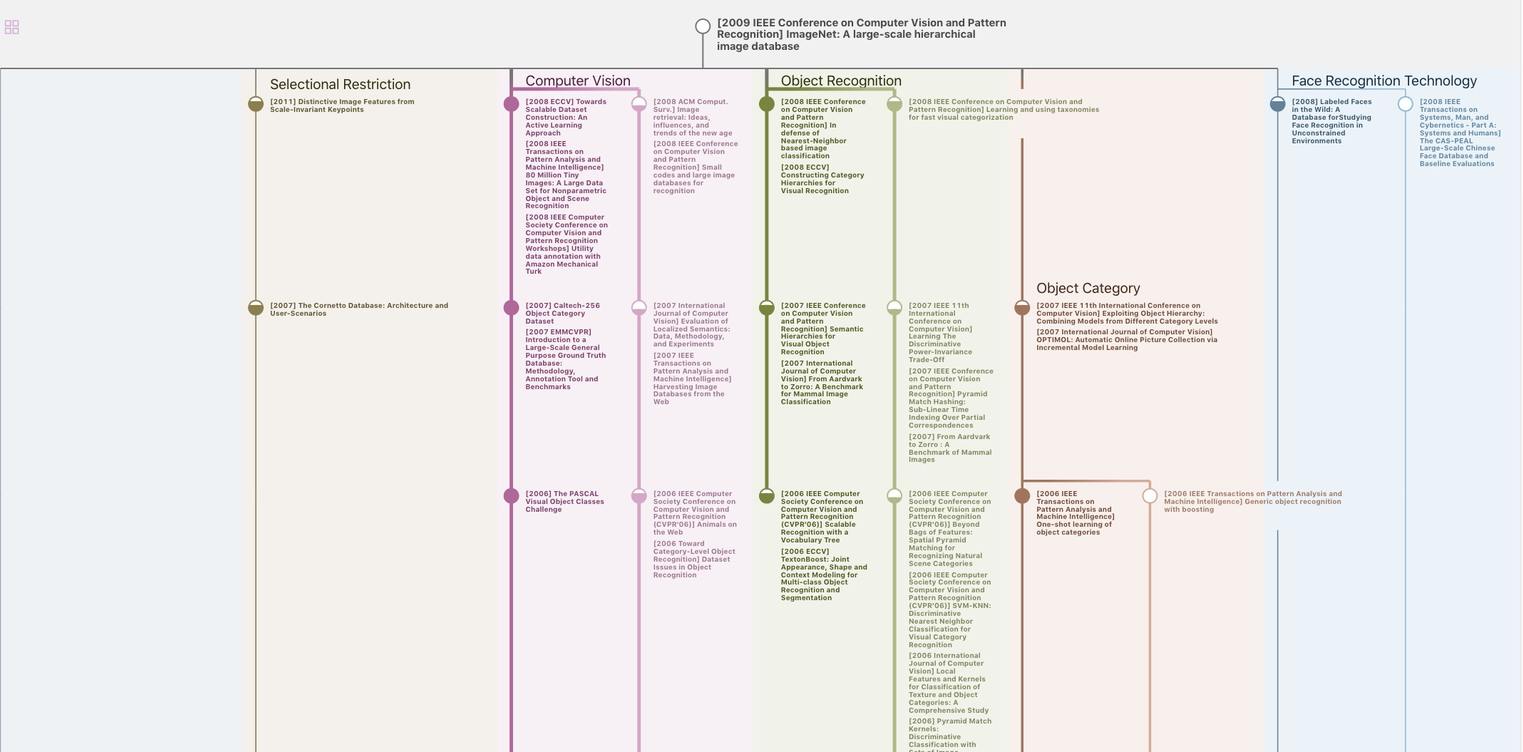
生成溯源树,研究论文发展脉络
Chat Paper
正在生成论文摘要