PPSL: Privacy-Preserving Text Classification for Split Learning
2022 4th International Conference on Data Intelligence and Security (ICDIS)(2022)
摘要
With the blooming of machine learning, Distributed Collaborative Machine Learning (DCML) approaches have been used in various applications to scale up the training process. However, they may have privacy issues that need to be addressed. Split learning is one of the latest DCML approaches that enable the training of the machine learning models without sharing the raw data. In this paper, we study the novel problem of building a privacy-preserving text classifier in the split learning setting. This task, however, is challenging due to the need to maintain the utility of the text data for downstream tasks while protecting privacy by preventing the leakage of private attributes. We address this dilemma of privacy and utility in this work. We propose a text classification for split learning, PPSL, which utilizes the adversarial learning to minimize the private attribute leakage. We study the impacts of increasing the training time and the number of hidden layers on the privacy of split learning. Our experimental results demonstrate the effectiveness of the proposed framework that retains the sentiment meaning and preserves the private attributes while minimizing the leakage.
更多查看译文
关键词
split learning,privacy,text,adversarial learning
AI 理解论文
溯源树
样例
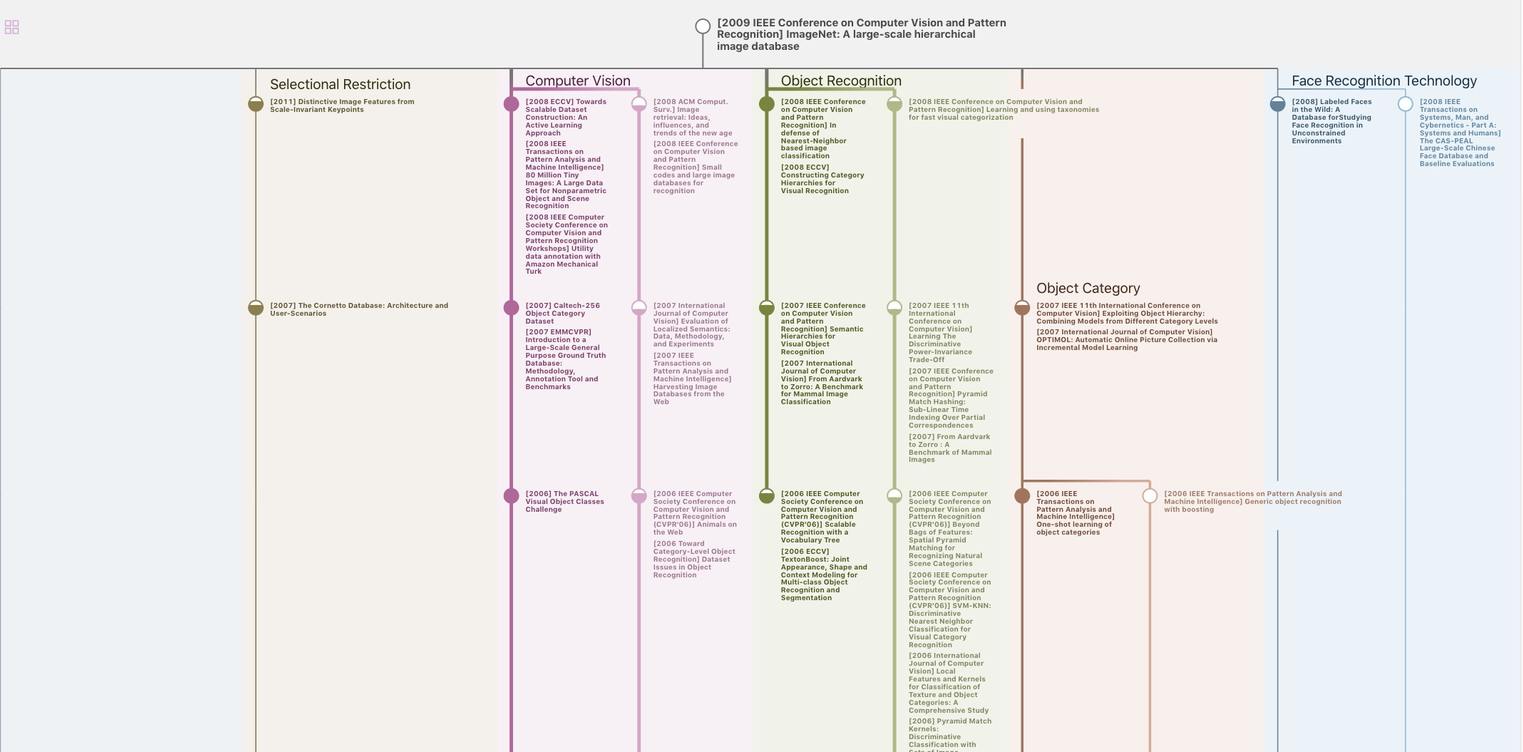
生成溯源树,研究论文发展脉络
Chat Paper
正在生成论文摘要