Context Feature Guided Network for Image Super-resolution
2022 4th International Conference on Data Intelligence and Security (ICDIS)(2022)
摘要
Convolutional neural networks (CNNs) have achieved remarkable advance in single image super-resolution (SISR). While seeking for better performance, most existing SR methods prone to be deeper or wider structure are usually computationally intensive, which thus limits the application of SR networks in resource-limited devices. In this paper, a novel lightweight SR network named Context Feature Guided Network (CFGN) is proposed for a better trade-off between performance and running efficiency. Specifically, to fully exploit semantic features, a novel Context Feature Guided Convolution (CFGC) is designed as the main building block for CFGN. The proposed CFGC consists of multiple stacked two-path fusion convolutions (TFCs), which could significantly enlarge the receptive field of each layer and learn more discriminative feature representations. To further consider rich multi-scale information, we design a context feature guided group (CFGG) as the main building block of CFGN by progressively stacking feature extraction distillation module and CFGCs. Extensive experiments demonstrate the superiority of the proposed method over the state-of-the-art lightweight SR models in terms of both quantitative metrics and visual quality.
更多查看译文
关键词
image super-resolution,convolutional neural networks,feature aggregation
AI 理解论文
溯源树
样例
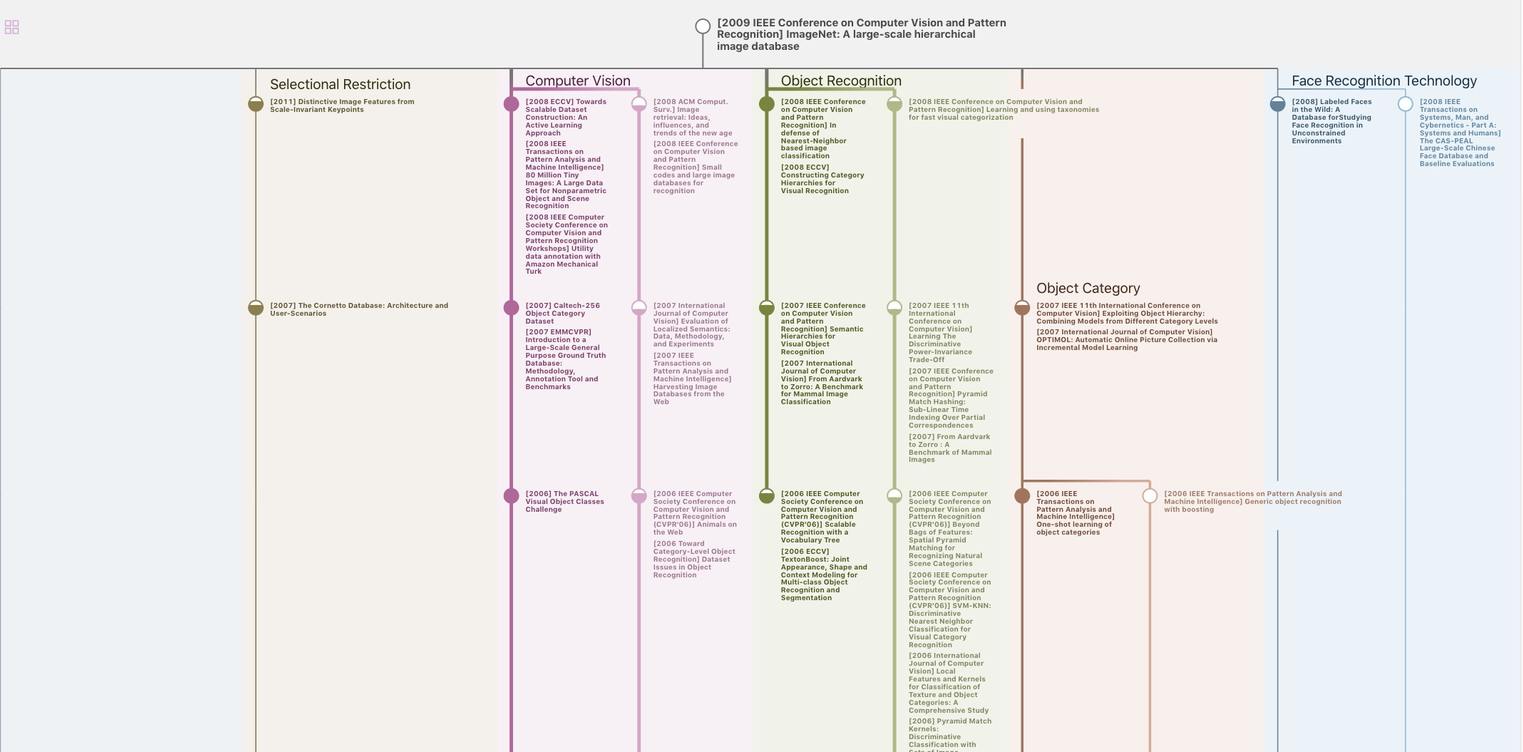
生成溯源树,研究论文发展脉络
Chat Paper
正在生成论文摘要