Understanding Geometry for Point Cloud Segmentation via Covariance
2022 15th International Congress on Image and Signal Processing, BioMedical Engineering and Informatics (CISP-BMEI)(2022)
摘要
Geometry plays a vital role in 3D point cloud semantic segmentation since each category of object exhibits a specific geometric pattern. However, popular point cloud semantic segmentation methods ignore this property during feature aggregation. In this paper, we propose a novel Covariance-based Geometry Encoder (CGE) to learn latent geometry representation in point clouds and break this limitation. Specifically, we find that the classic covariance matrix can represent geometry implicitly in a point neighborhood, and we can learn geometry representation through simple multi-layer perceptrons to enhance the point features in a deep network. The proposed CGE module is generally applicable to any point-based network, while only requiring a little extra computing. Through extensive experiments, our method shows competitive performance on both indoor and outdoor benchmark datasets. Code will be publicly available.
更多查看译文
关键词
point cloud segmentation,geometry,understanding
AI 理解论文
溯源树
样例
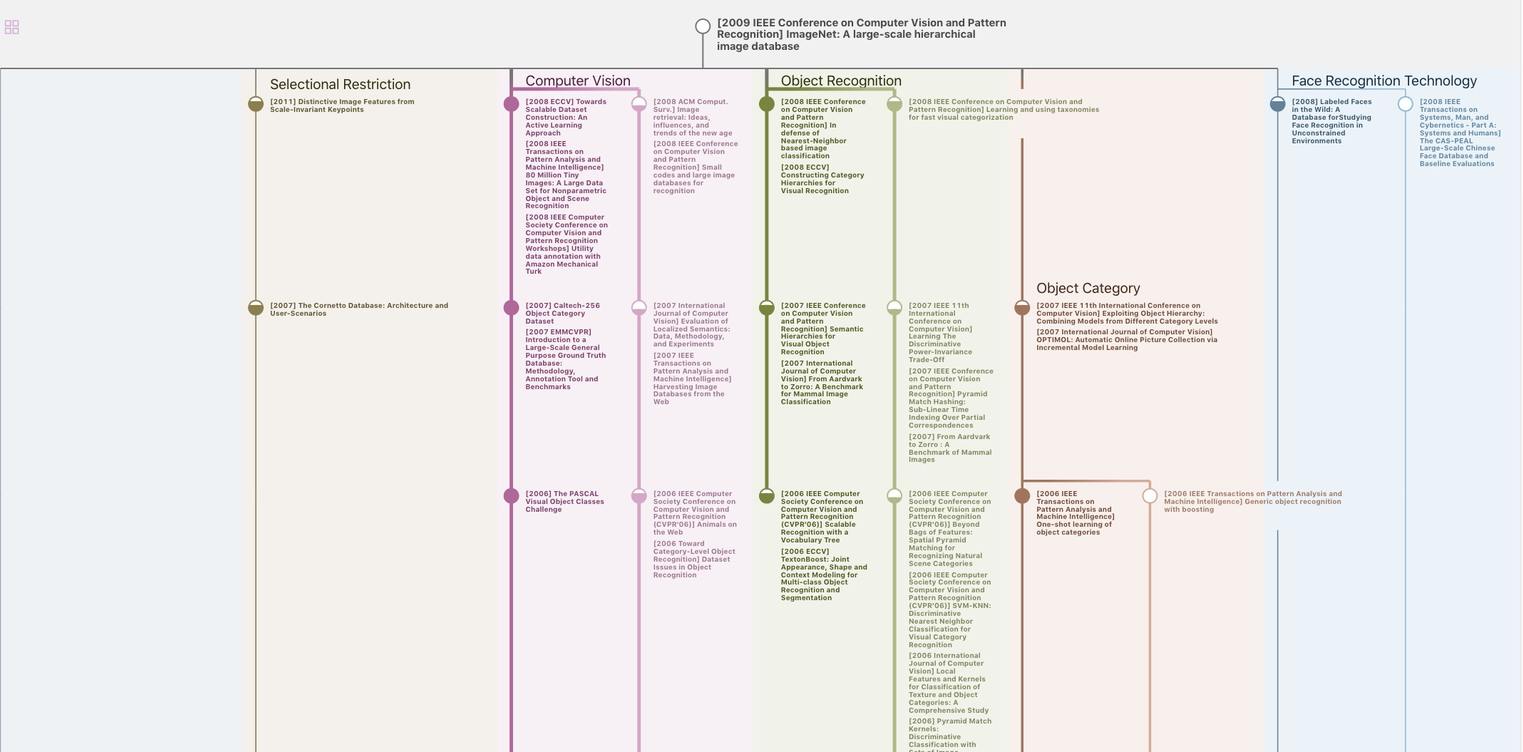
生成溯源树,研究论文发展脉络
Chat Paper
正在生成论文摘要