IronForge: An Open, Secure, Fair, Decentralized Federated Learning
IEEE TRANSACTIONS ON NEURAL NETWORKS AND LEARNING SYSTEMS(2023)
摘要
Federated learning (FL) offers an effective learning architecture to protect data privacy in a distributed manner. However, the inevitable network asynchrony, overdependence on a central coordinator, and lack of an open and fair incentive mechanism collectively hinder FL's further development. We propose IronForge, a new generation of FL framework, that features a directed acyclic graph (DAG)-based structure, where nodes represent uploaded models, and referencing relationships between models form the DAG that guides the aggregation process. This design eliminates the need for central coordinators to achieve fully decentralized operations. IronForge runs in a public and open network and launches a fair incentive mechanism by enabling state consistency in the DAG. Hence, the system fits in networks where training resources are unevenly distributed. In addition, dedicated defense strategies against prevalent FL attacks on incentive fairness and data privacy are presented to ensure the security of IronForge. Experimental results based on a newly developed test bed FLSim highlight the superiority of IronForge to the existing prevalent FL frameworks under various specifications in performance, fairness, and security. To the best of our knowledge, IronForge is the first secure and fully decentralized FL (DFL) framework that can be applied in open networks with realistic network and training settings.
更多查看译文
关键词
Task analysis,Training,Data models,Peer-to-peer computing,Adaptation models,Security,Scalability,Blockchain,decentralization,directed acyclic graph (DAG),federated learning (FL),incentive
AI 理解论文
溯源树
样例
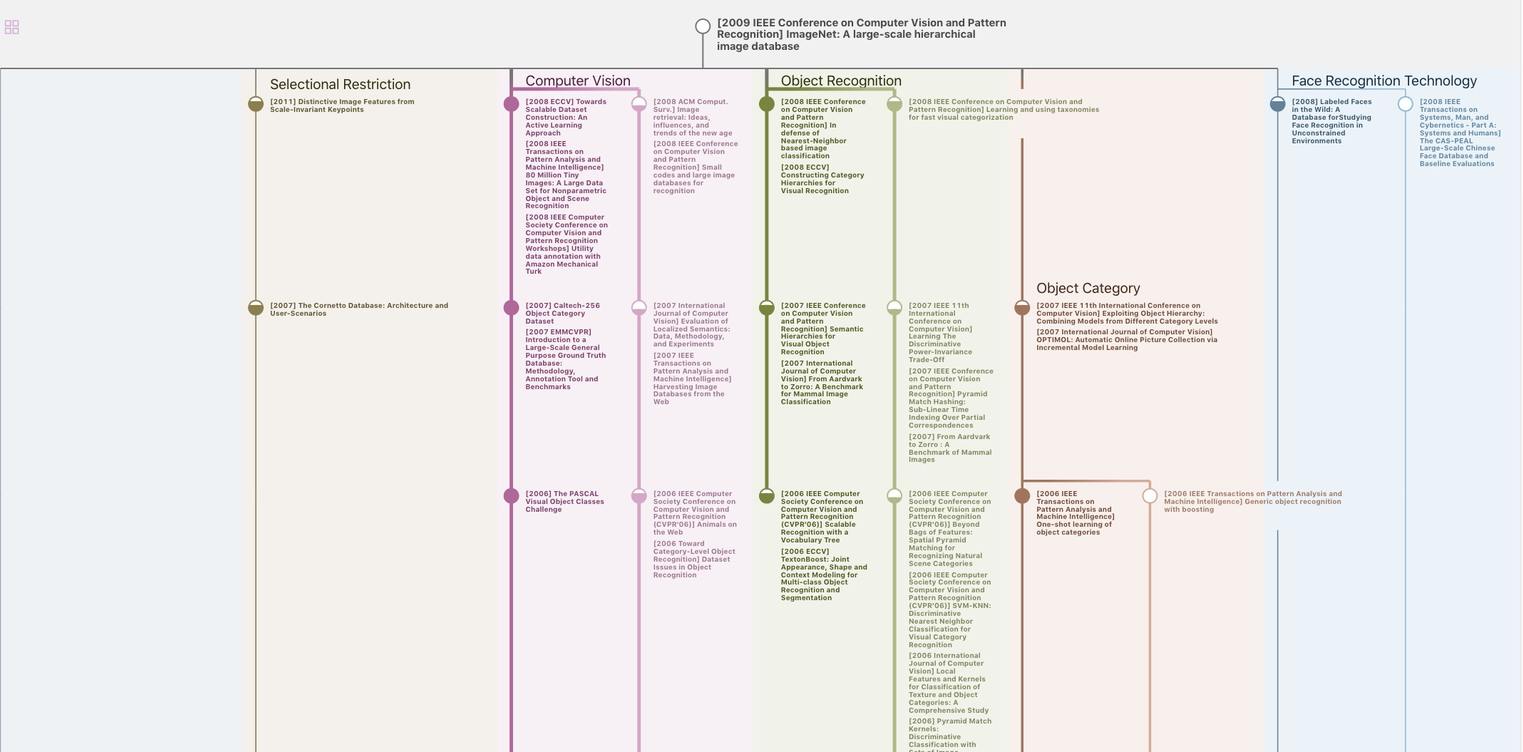
生成溯源树,研究论文发展脉络
Chat Paper
正在生成论文摘要