Sugar Beets and Weed Detection using Semantic Segmentation
2022 International Conference on Fuzzy Theory and Its Applications (iFUZZY)(2022)
摘要
Weeds have a big impact on beet growth. As a result, there is a growing public interest in weed detection and identification during crop growth. This paper describes a UNet++ method with deep supervision for detecting weeds in sugar beet fields trained on the dataset. The UNet++ architecture is an encoder-decoder network that closes the semantic gap between the feature maps of the encoder and decoder sub-networks through redesigned skip paths. When the feature maps of the encoder and decoder networks are semantically similar, the optimizer will handle the learning task more easily. We evaluate the comparison of UNet, UNet++, and UNet++ with deep supervision architectures on the dataset segmentation task. Experiments show that the mIoU values on UNet, UNet++ and UNet++ with deep supervision are about 90.81%, 92.00% and 92.34%. It turns out that UNet++ with deep supervision can detect the location of weeds more effectively.
更多查看译文
关键词
Deep Learning,Weed,Sugar Beet,Detection Classification,Nested UNet
AI 理解论文
溯源树
样例
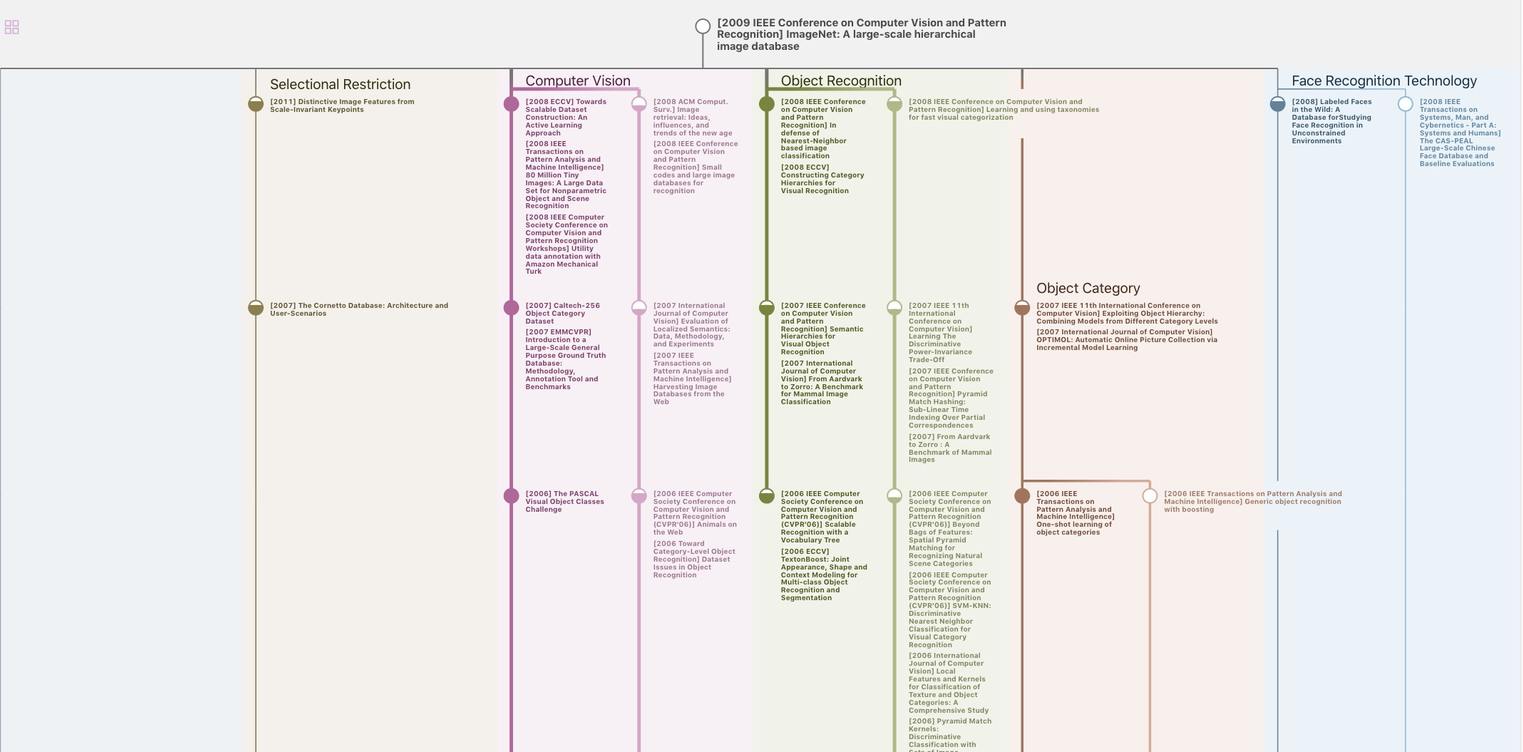
生成溯源树,研究论文发展脉络
Chat Paper
正在生成论文摘要