Hyperparameter Optimization for Convolutional Neural Networks with Genetic Algorithms and Bayesian Optimization
2022 IEEE Latin American Conference on Computational Intelligence (LA-CCI)(2022)
摘要
Deep learning (DL) has emerged as a leading approach to solving complex problems in multiple areas. Its success is mainly due to technological development, large volumes of data, and various algorithms based on artificial neural networks (ANN). However, their functioning and performance are sensitive to the configuration of hyperparameters, which result from trial and error processes based on the knowledge and creativity of experts. In this sense, to reduce subjective associated with human intervention, an approach suggests tackling the tuning of hyper-parameters as an optimization problem. Here, the objective is to find a configuration that maximizes some performance metrics. The present work presents an implementation and comparison of three optimization strategies (Random Search, Bayesian Opti-mization, and Genetic Algorithm) for image classification in the CIFAR-10 dataset using convolutional neural networks, showing that algorithm performance and their solutions are tightly bound with the kind of architecture and search space.
更多查看译文
关键词
hyperparameter optimization,deep learning,genetic algorithms,bayesian optimization,convolutional neural network
AI 理解论文
溯源树
样例
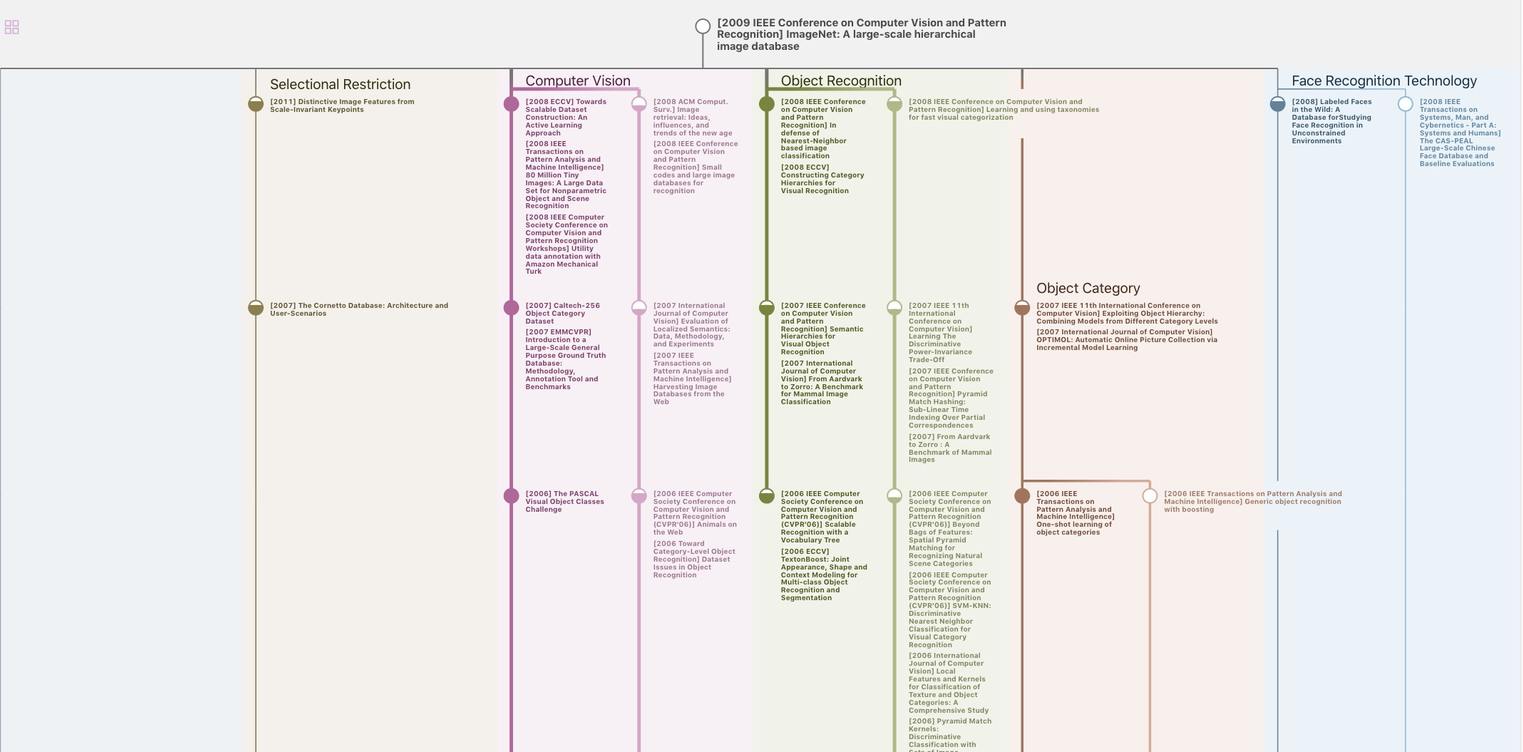
生成溯源树,研究论文发展脉络
Chat Paper
正在生成论文摘要