Feature selection and cascade dimensionality reduction for self-supervised visual representation learning
Computers and Electrical Engineering(2023)
摘要
Self-supervised visual representation learning focuses on capturing comprehensive features via exploiting the unlabeled datasets. However, existing contrastive learning based SSL frameworks are subjected to higher computational consumption and unsatisfactory performance. To handle these issues, we present a novel single-branch SSL method that incorporates an adaptive feature selection and activation module and a progressive cascade dimensionality reduction module, called APNet. Specifically, our method first fully exploits the unlabeled datasets and extracts intra- and inter-image information via introducing montage image. In addition, a novel adaptive feature selection and activation module is designed to generate the most comprehensive features. Besides, a progressive cascade dimensionality reduction module is proposed to capture the most representative features from latent vectors through cascade dimensionality increasing–decreasing operations. Extensive experiments have demonstrated the robustness and effectiveness of APNet. Specifically, APNet exceeds MoCo-v3 by 3.1% on the ImageNet-100 dataset, and consumes only half of the calculation. Code is available at https://github.com/AI-TYQ/APNet.
更多查看译文
关键词
Unsupervised learning,Feature selection and activation,Self-supervised learning,Cascade dimensionality reduction
AI 理解论文
溯源树
样例
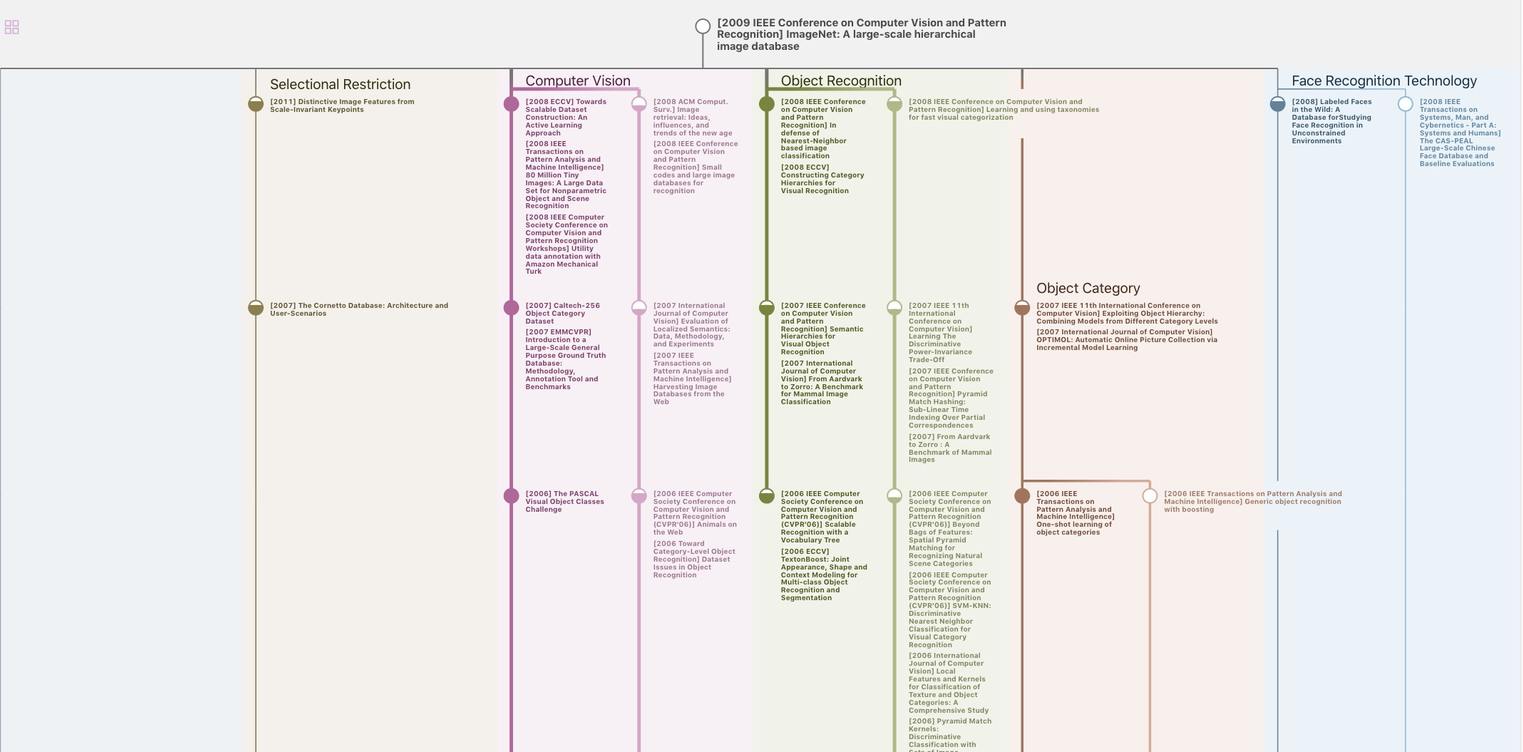
生成溯源树,研究论文发展脉络
Chat Paper
正在生成论文摘要