Enabling smart curb management with spatiotemporal deep learning
Computers, Environment and Urban Systems(2023)
摘要
Curb spaces are important assets to cities. They are often used by travelers to switch transportation means, visitors to access curbside properties, and municipalities to place roadside infrastructure. The promotion of multi-modal transportation and the emergence of new mobility services have complicated both curb environments and their management. Consequently, some cities have started to explore new strategies for curb management, but lacked the anticipation on how different curb regulations and built-environment features may collectively influence curb-use patterns across user groups. We make a step toward smart curb environment by proposing a graph-based deep learning approach, i.e., MultiGCN-LSTM, to predict diverse curb uses across time and space. We used two graph convolution layers and an LSTM layer to capture the spatial, temporal, and semantic dependencies between curb regulations, built-environment semantics, and diverse curb uses. Two place-specific models were developed separately for a medium-sized college town and a metropolitan in the U.S. The effectiveness of the proposed models was validated with ablation studies and demonstrated in three scenario experiments. The research contributes to smart curb management in the face of more diversified and intensified curb uses with new mobility services and emerging vehicular technologies.
更多查看译文
关键词
Built environment,Curb management,Graph-based deep learning,Smart cities,Scenario planning
AI 理解论文
溯源树
样例
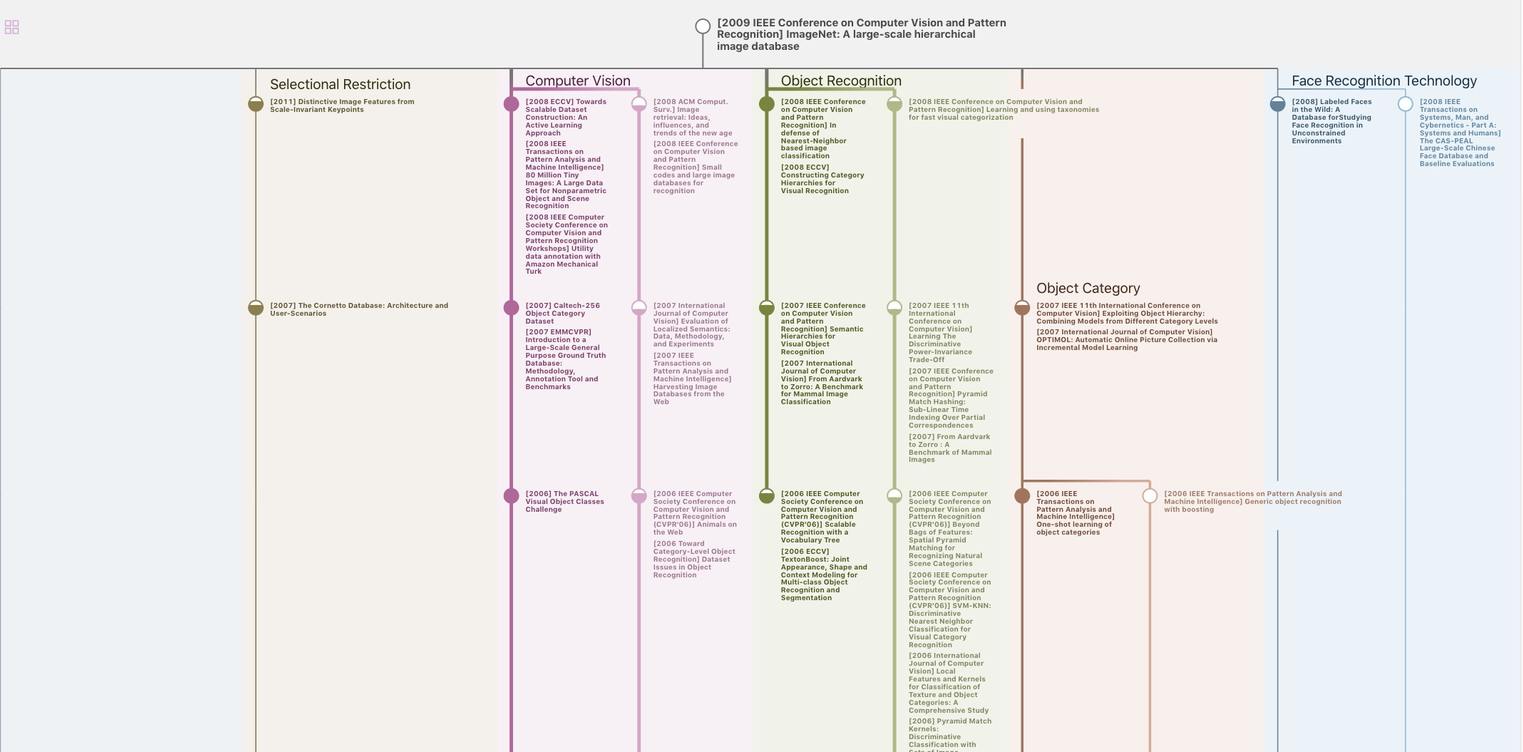
生成溯源树,研究论文发展脉络
Chat Paper
正在生成论文摘要