Extending the Fellegi-Sunter record linkage model for mixed-type data with application to the French national health data system
Computational Statistics & Data Analysis(2023)
摘要
Probabilistic record linkage is a process of combining data from different sources, when such data refer to common entities and identifying information is not available. A probabilistic record linkage framework that takes into account multiple non-identifying information that this is limited to simple binary comparison between matching variables has been previously proposed. An extension of this method is proposed for mixed-type comparison vectors. A mixture model for handling comparison values of low prevalence categorical matching variables, and a mixture of hurdle gamma distribution for handling comparison values of continuous matching variables have been developed. The parameters are estimated by means of the Expectation Conditional Maximization (ECM) algorithm. Through a Monte Carlo simulation study, both the posterior probability estimation for a record pair to be a match and the prediction of matched record pairs are evaluated. The simulation results indicate that the proposed methods outperform existing ones in most considered cases. The proposed methods are applied on a real dataset, to perform linkage between a registry of patients suffering from venous thromboembolism in the Brest district area (GETBO) and the French national health information system (SNDS).
更多查看译文
关键词
Expectation Conditional Maximization (ECM) algorithm,Hurdle gamma distribution,Low prevalence variables,Mixture model,Probabilistic record linkage
AI 理解论文
溯源树
样例
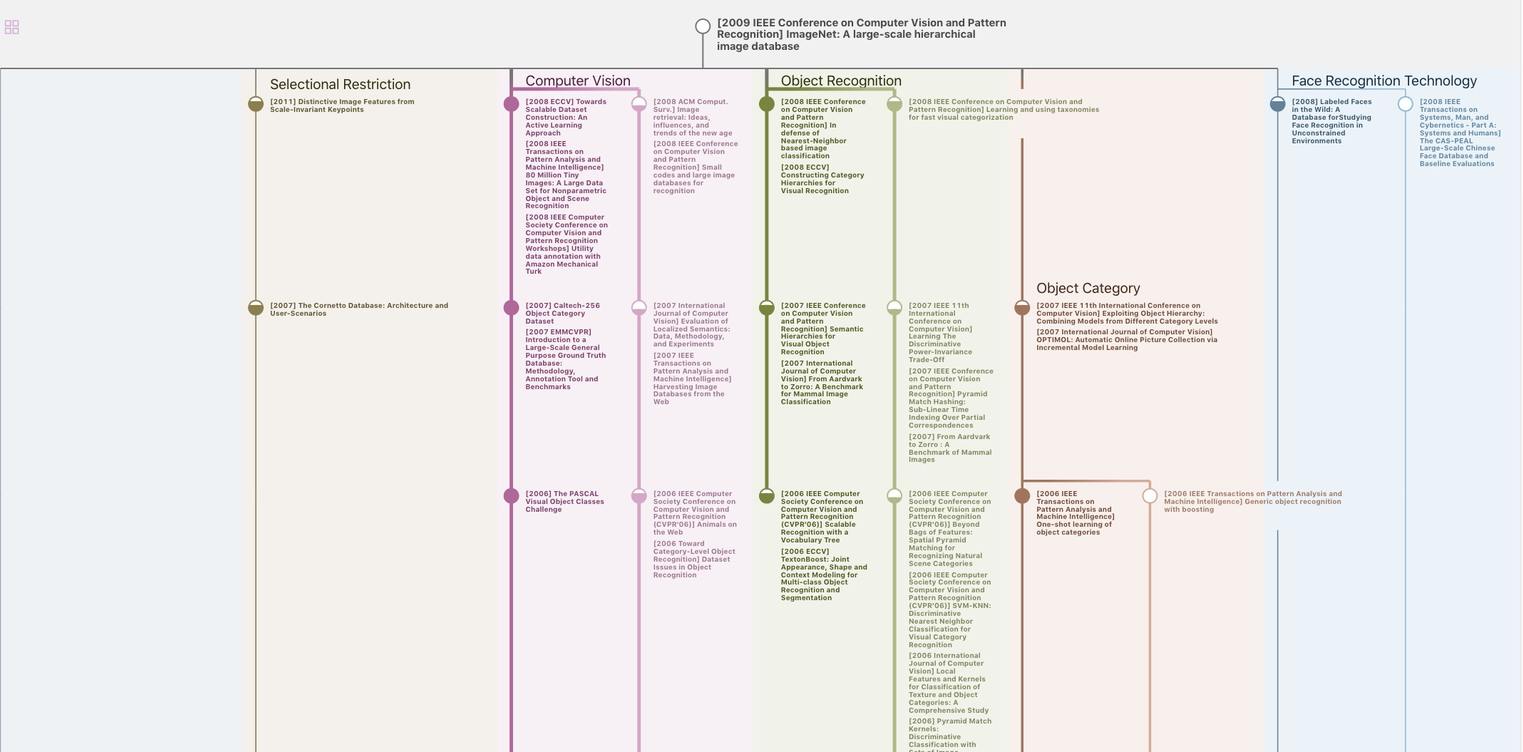
生成溯源树,研究论文发展脉络
Chat Paper
正在生成论文摘要