Clustering multivariate count data via Dirichlet-multinomial network fusion
Computational Statistics & Data Analysis(2023)
摘要
Clustering of multivariate count data has widespread applications in areas such as text analysis and microbiome studies. The need to account for overdispersion generally results in a nonconvex loss function, which does not fit into the existing convex clustering framework. Moreover, prior knowledge of a network over the samples, often available from citation or similarity relationships, is not taken into account. We introduce Dirichlet-multinomial network fusion (DMNet) for clustering multivariate count data, which models the samples via Dirichlet-multinomial distributions with individual parameters and employs a weighted group L1 fusion penalty to pursue homogeneity over a prespecified network. To circumvent the nonconvexity issue, we present two exponential family approximations to the Dirichlet-multinomial distribution, which are amenable to efficient optimization and theoretical analysis. We derive an ADMM algorithm and establish nonasymptotic error bounds for the proposed methods. Our bounds involve a trade-off between the connectivity of the network and its fidelity to the true parameter. The usefulness of our methods is illustrated through simulation studies and two text clustering applications.
更多查看译文
关键词
Convex clustering,Exponential family approximation,Group L1 fusion,Nonasymptotic error bound,Overdispersion,Text analysis
AI 理解论文
溯源树
样例
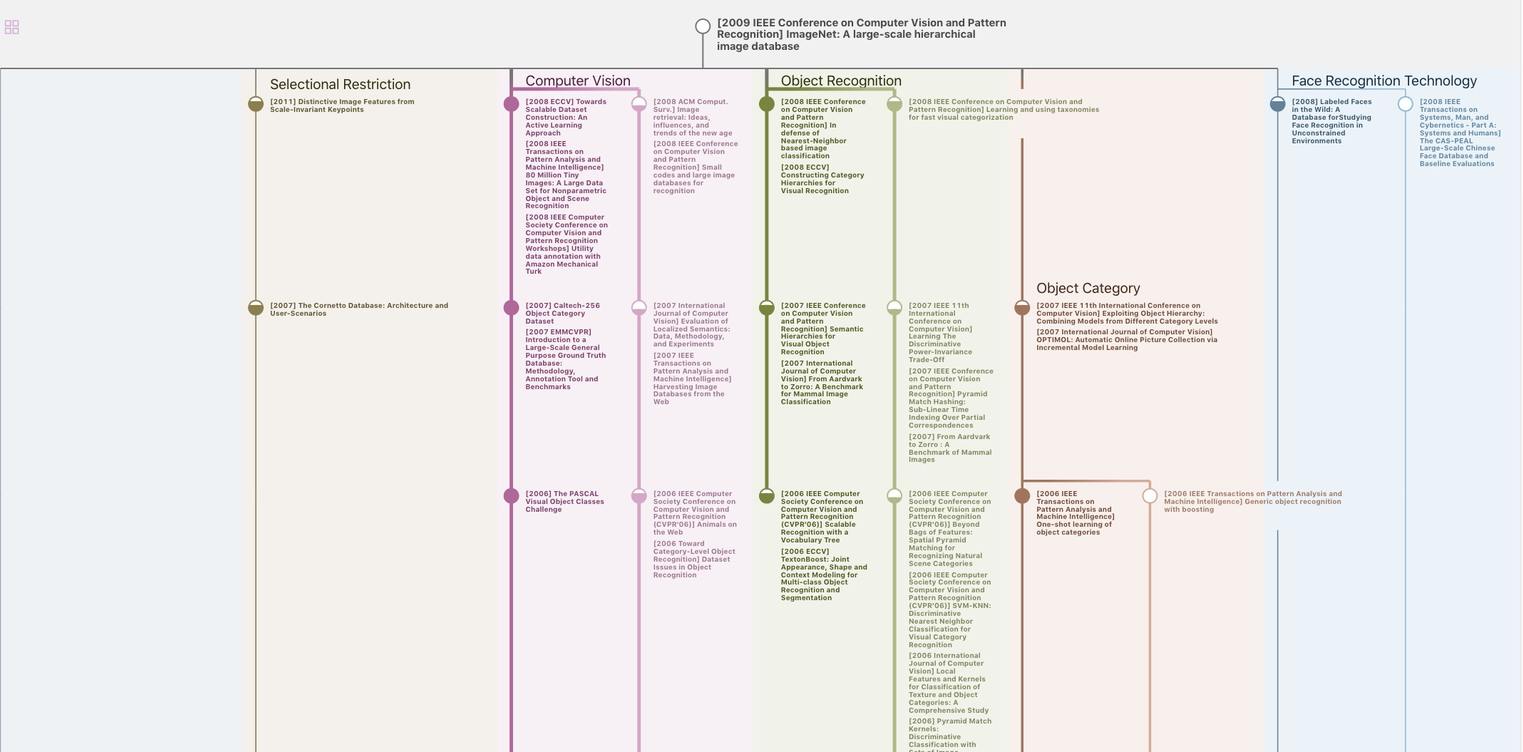
生成溯源树,研究论文发展脉络
Chat Paper
正在生成论文摘要