Invariance measures for neural networks
Applied Soft Computing(2023)
摘要
Invariances in neural networks are useful and necessary for many tasks. However, the representation of the invariance of most neural network models has not been characterized. We propose measures to quantify the invariance of neural networks in terms of their internal representation. The measures are efficient and interpretable, and can be applied to any neural network model. They are also more sensitive to invariance than previously defined measures. We validate the measures and their properties in the domain of affine transformations and the CIFAR10 and MNIST datasets, including their stability and interpretability. Using the measures, we perform a first analysis of CNN models and show that their internal invariance is remarkably stable to random weight initializations, but not to changes in dataset or transformation. We believe the measures will enable new avenues of research in invariance representation.
更多查看译文
关键词
Invariance,Neural networks,Transformations,Convolutional neural networks,Measures
AI 理解论文
溯源树
样例
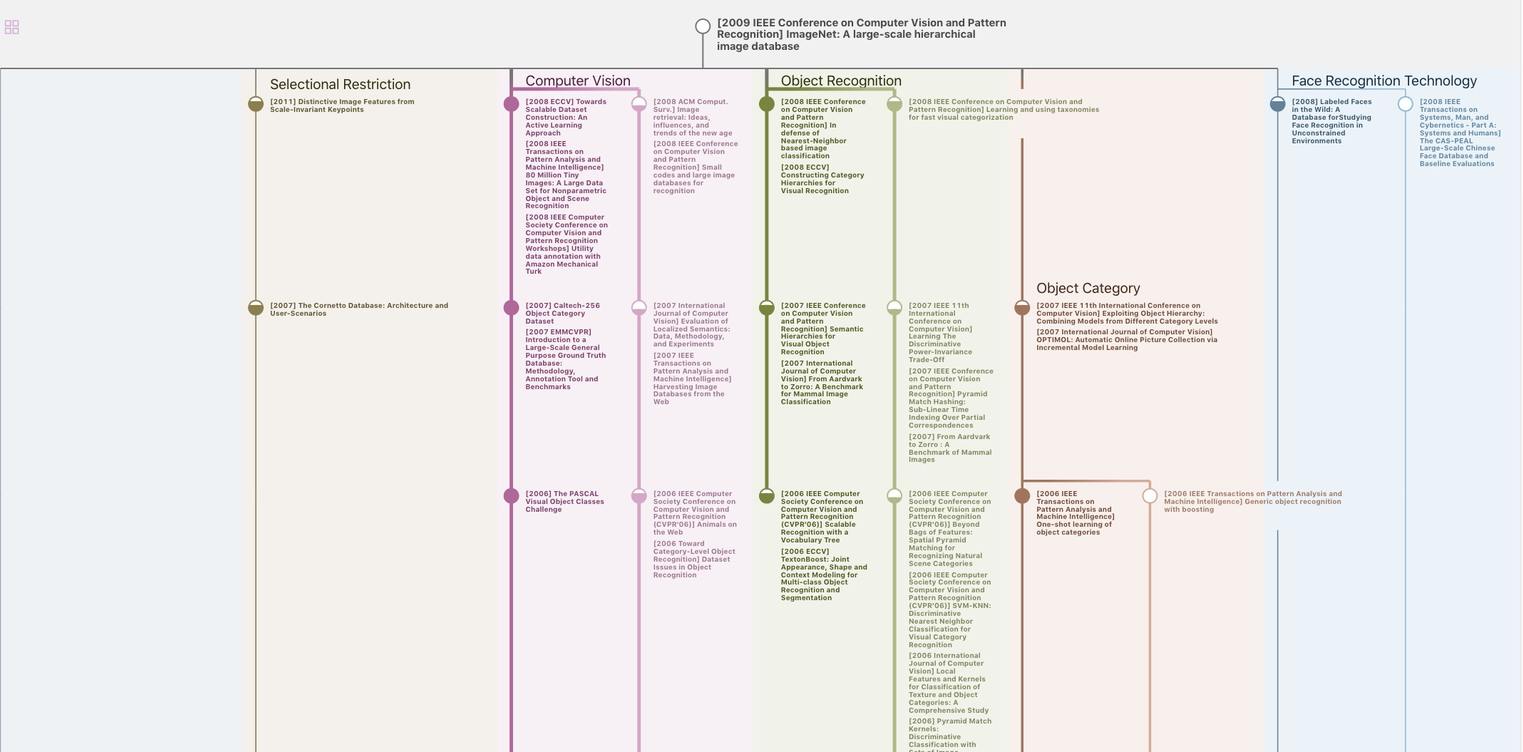
生成溯源树,研究论文发展脉络
Chat Paper
正在生成论文摘要