A probabilistic forecasting approach for air quality spatio-temporal data based on kernel learning method
Applied Soft Computing(2023)
摘要
Reliable air quality index forecasting is an important guarantee for government to take early emergency measures to protect the ecological environment and public health. Nevertheless, providing accurate and reliable forecasting is still a difficult and challenging task, owing to the high nonlinearity and noise in the AQI (air quality index). This study develops a novel framework based on feature selection, the Error Correction Model, and the Residual estimation method with new structure fast kernels, to conveniently improve the prediction performance and efficiently quantify the uncertainty of the point prediction generated by any pre-trained model. This framework takes some representative ML (machine learning) tools as examples, with the significant input variables extracted by the two-stage feature selection method for accurate AQI point prediction, and models their residuals by the multi-output Gaussian process and the multi-output Student -t process, which rely on the new multi-output I/O kernels with chronological memory to embed the relevant information of the point prediction and the residuals as well as of different output for making predictions on residual distributions, thereby enhancing predic-tion performance and enriching uncertainty information. Besides, an acceleration method based on the Orthogonal Random Features method is also proposed to enable the framework to handle large-scale data at an acceptable time cost. The framework is validated by the AQI Spatio-temporal data of four observation points in Shijiazhuang, China, and the result reveals that the proposed framework provides valid prediction intervals and enhances the accuracy of the ML models' point predictions. Specifically, the framework reduces the prediction error of almost all basic models, and provides shorter prediction intervals than other common methods. Especially, the multi-output GP model or multi-output TP model obtains the best improvement in point prediction and the shortest prediction intervals.(c) 2022 Elsevier B.V. All rights reserved.
更多查看译文
关键词
Hierarchical model,Error correction,Interval prediction,Gaussian process,Student,t process,Uncertainty quantification
AI 理解论文
溯源树
样例
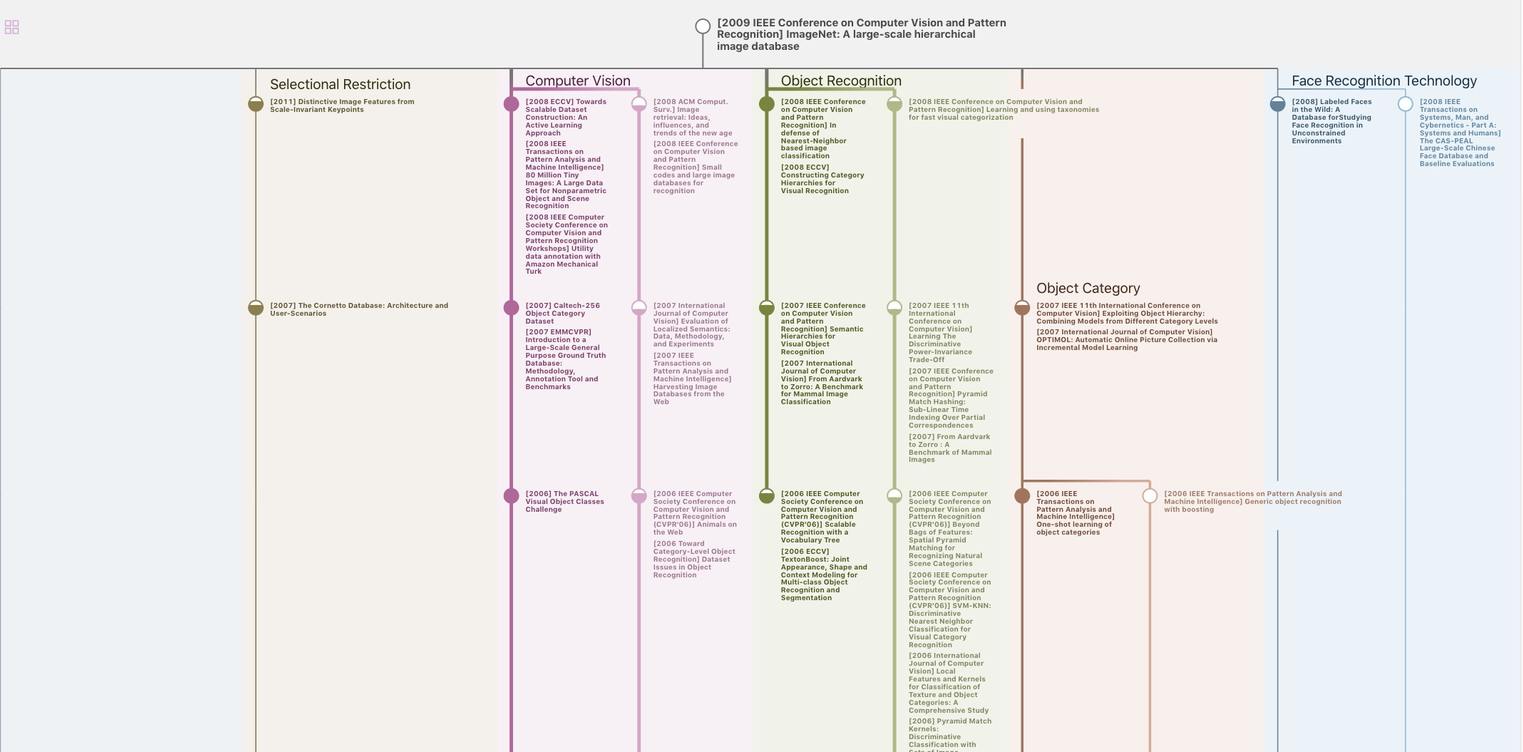
生成溯源树,研究论文发展脉络
Chat Paper
正在生成论文摘要