Contingency Space: A Semimetric Space for Classification Evaluation
IEEE Transactions on Pattern Analysis and Machine Intelligence(2023)
摘要
In Machine Learning, a supervised model’s performance is measured using the evaluation metrics. In this study, we first present our motivation by revisiting the major limitations of these metrics, namely one-dimensionality, lack of context, lack of intuitiveness, uncomparability, binary restriction, and uncustomizability of metrics. In response, we propose Contingency Space, a bounded semimetric space that provides a generic representation for any performance evaluation metric. Then we showcase how this space addresses the limitations. In this space, each metric forms a surface using which we visually compare different evaluation metrics. Taking advantage of the fact that a metric’s surface warps proportionally to the degree of which it is sensitive to the class-imbalance ratio of data, we introduce Imbalance Sensitivity that quantifies the skew-sensitivity. Since an arbitrary model is represented in this space by a single point, we introduce Learning Path for qualitative and quantitative analyses of the training process. Using the semimetric that contingency space is endowed with, we introduce Tau as a new cost sensitive and Imbalance Agnostic metric. Lastly, we show that contingency space addresses multi-class problems as well. Throughout this work, we define each concept through stipulated definitions and present every application with practical examples and visualizations.
更多查看译文
关键词
Machine learning,model validation and analysis,knowledge representation formalisms and methods
AI 理解论文
溯源树
样例
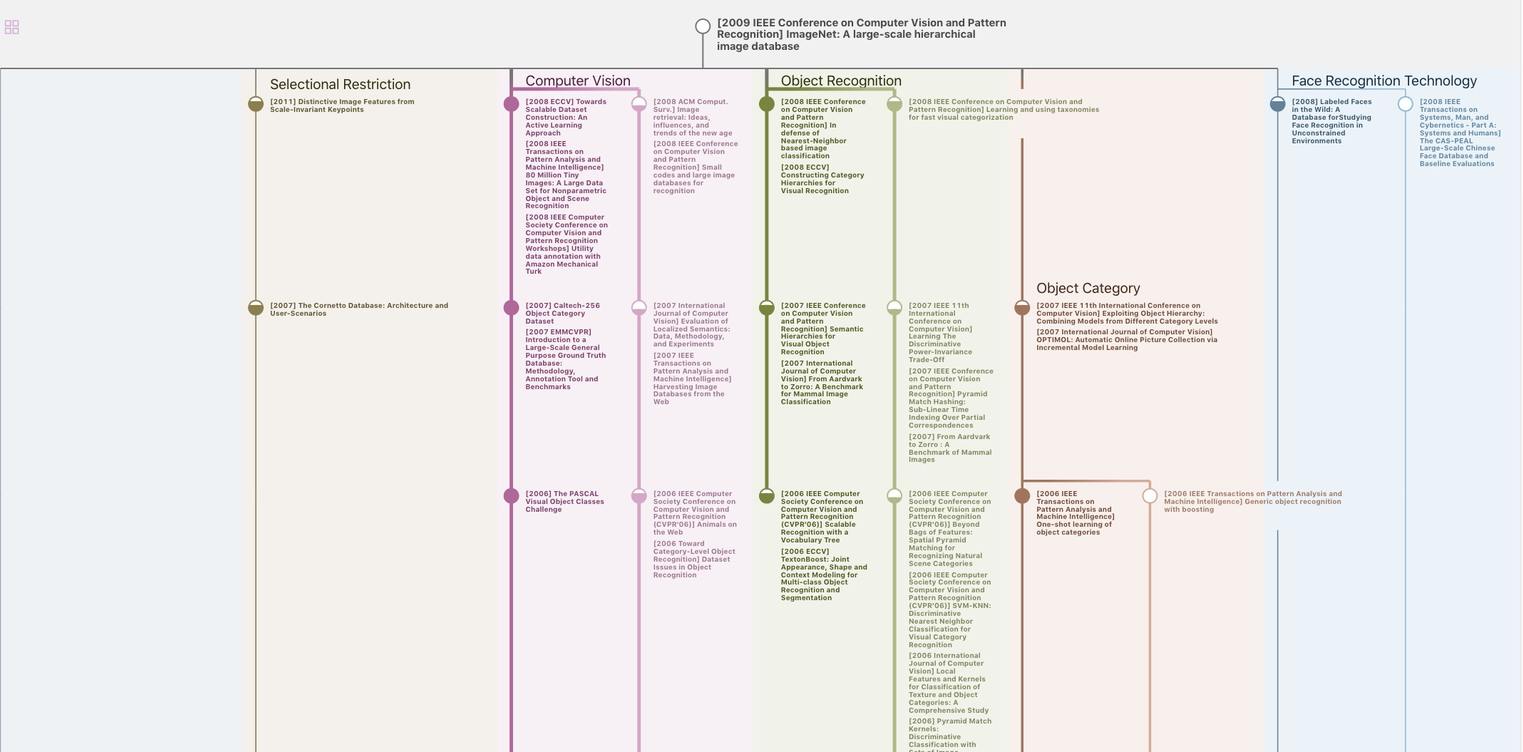
生成溯源树,研究论文发展脉络
Chat Paper
正在生成论文摘要