Generative adversarial feature learning for glomerulopathy histological classification
Biomedical Signal Processing and Control(2023)
摘要
Early diagnosis of glomerulopathy is significant for improving disease control and prognosis. Glomerulopathy is diagnosed by pathological images obtained from a renal biopsy, which has many complications and contraindications. In this work, we propose to utilize noninvasive renal ultrasound images to predict the pathological type of glomerulopathy. Due to the difficulty of pathological type recognition on renal ultrasound images and small amounts of annotated data, effective feature learning is more important. Therefore, we employ an adversarial learning approach to generate feature embeddings rather than images. AC-GAN, using conditional image generation and auxiliary classifier, is deployed to synthesize class-related image features. A basic residual network is applied to extract real renal features. After adversarial feature learning, an additional classifier is used for further optimizing and updating the feature extraction network. Through adversarial learning, the feature embeddings extracted by feature extraction network become more informative and discriminative. The t-SNE visualization of extracted features demonstrates that learned features by adversarial feature learning are more discriminative with image categories than others, and this adversarial feature learning architecture achieves 85% accuracy and 0.8542 AUC value, which is better than single classification network and image generation augmentation strategy.
更多查看译文
关键词
Renal ultrasound,Pathological types,Generative Adversarial Networks,Feature generation,Adversarial learning
AI 理解论文
溯源树
样例
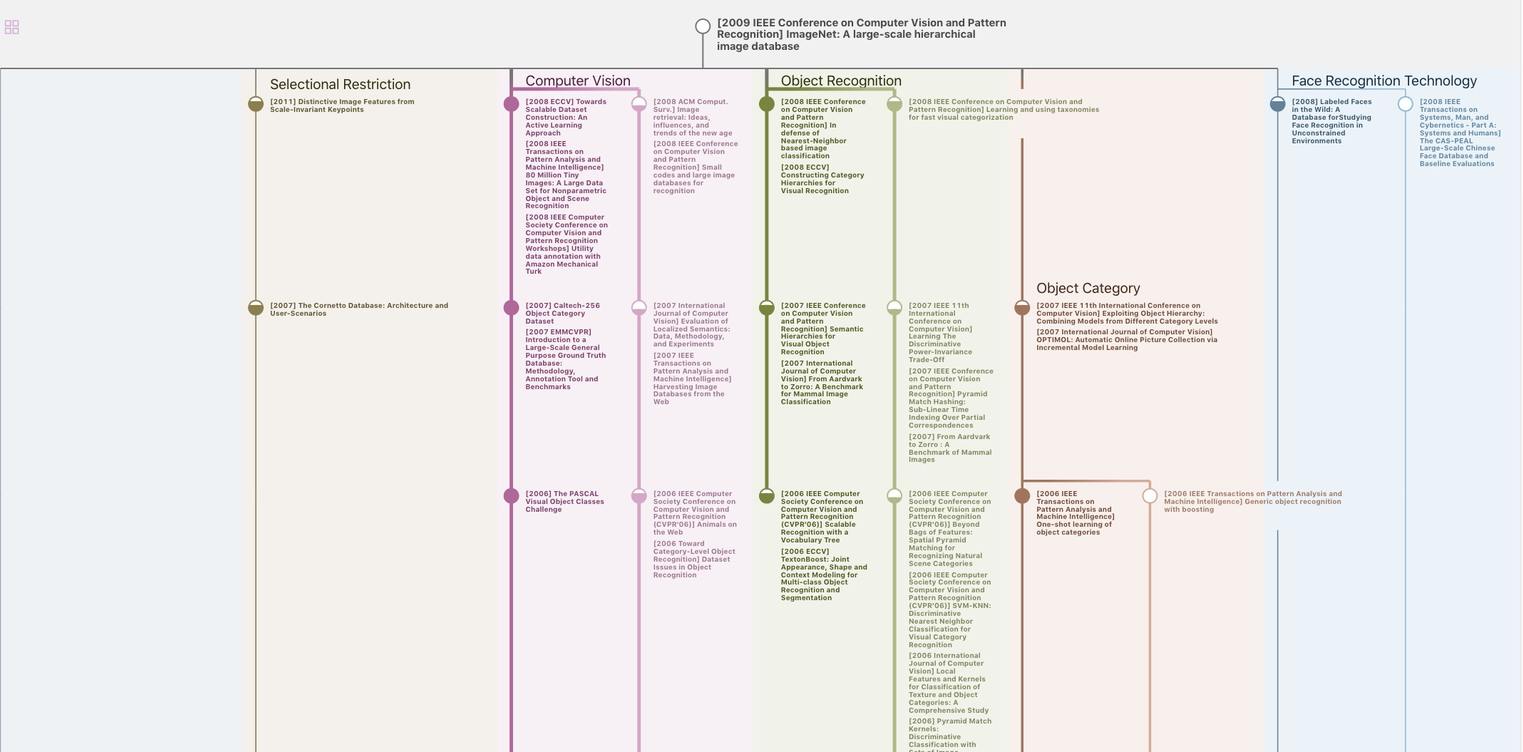
生成溯源树,研究论文发展脉络
Chat Paper
正在生成论文摘要