A practical extraction and spatial statistical pipeline for large 3D bioimages
biorxiv(2022)
摘要
A central tenet of biology and medicine is that there is a functional meaning underlying the cellular organisation of tissues and organs. Recent advances in histopathology and microscopy have achieved detailed visualisation of an increasing number of cell types in situ. Efficient methodologies to extract data from 3D images and draw detailed statistical inferences are, however, still lacking. Here we present a pipeline that can identify the location and classification of millions of cells contained in large 3D biological images using object detection neural networks that have been trained on more readily annotated 2D data alone. To draw meaning from the resulting data, we introduce a series of statistical techniques that are tailored to work with spatial data, resulting in a 3D statistical map of the tissue from which multi-cellular relationships can be clearly understood. As illustrations of the power of the approach, we apply these techniques to bone marrow images from intravital microscopy (IVM) and clarified 3D thick sections. These examples demonstrate that precise, large-scale data extraction is feasible, and that statistical techniques that are specifically designed for spatial data can distinctly reveal coherent, useful biological information.
### Competing Interest Statement
The authors have declared no competing interest.
更多查看译文
AI 理解论文
溯源树
样例
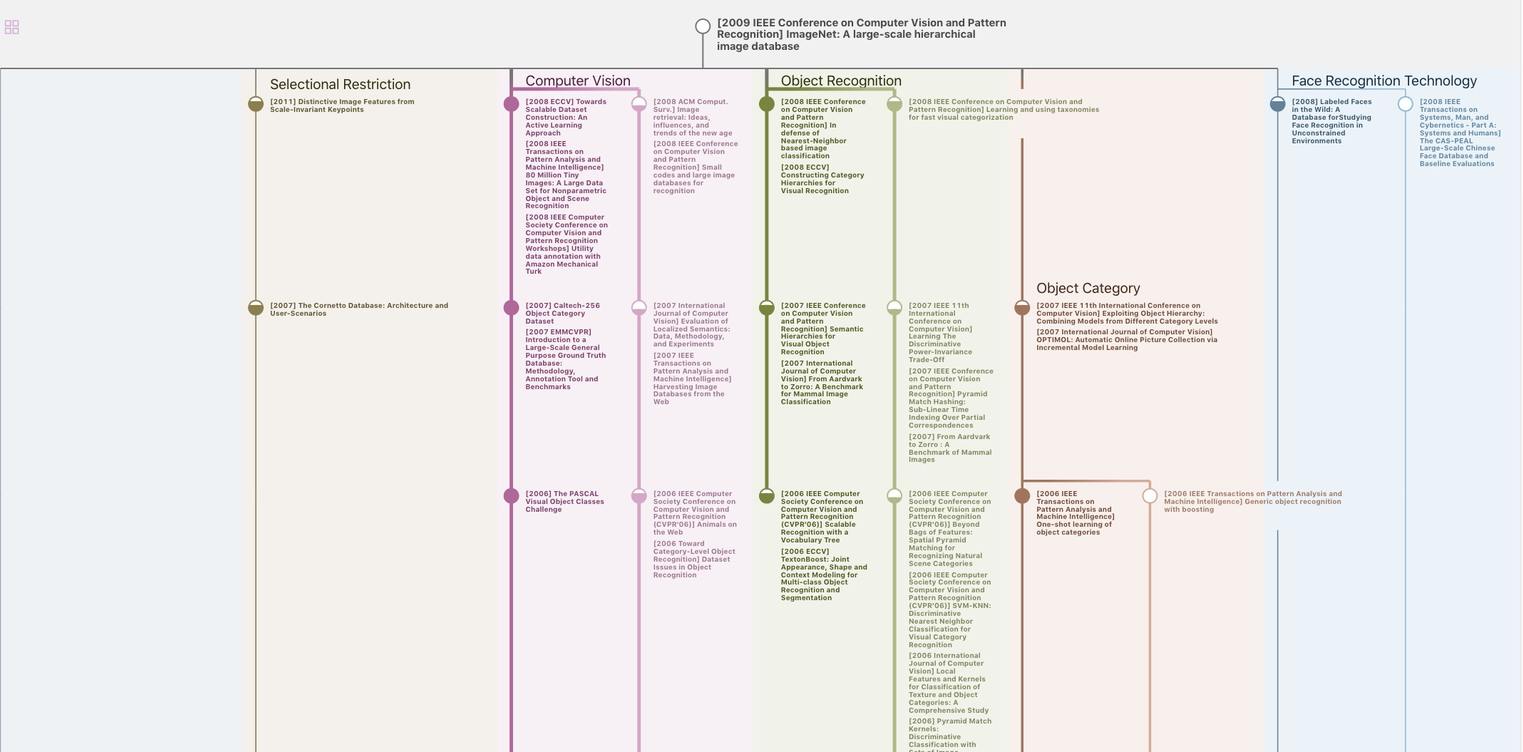
生成溯源树,研究论文发展脉络
Chat Paper
正在生成论文摘要