Evaluating protein binding interfaces with transformer networks
biorxiv(2023)
摘要
Computational protein-binding studies are widely used to investigate fundamental biological processes and facilitate the development of modern drugs, vaccines and therapeutics. Scoring functions aim to assess and rank the binding strength of the predicted protein complex. However, accurate scoring of protein binding interfaces remains a challenge. Here we show that our evaluating Protein binding Interfaces with Transformer Networks (PIsToN) approach can distinguish native-like protein complexes from incorrect conformations. Protein interfaces are transformed into a collection of two-dimensional images (interface maps), each corresponding to a geometric or biochemical property. Pixel intensities represent the feature values. A neural network was adapted from a popular vision transformer with several enhancements: a hybrid component to accept empirical-based energy terms, a multi-attention module to highlight essential features and binding sites, and the use of contrastive learning for better ranking performance. The resulting PIsToN model substantially outperforms state-of-the-art scoring functions on well-known datasets.
更多查看译文
关键词
Machine learning,Protein analysis,Engineering,general
AI 理解论文
溯源树
样例
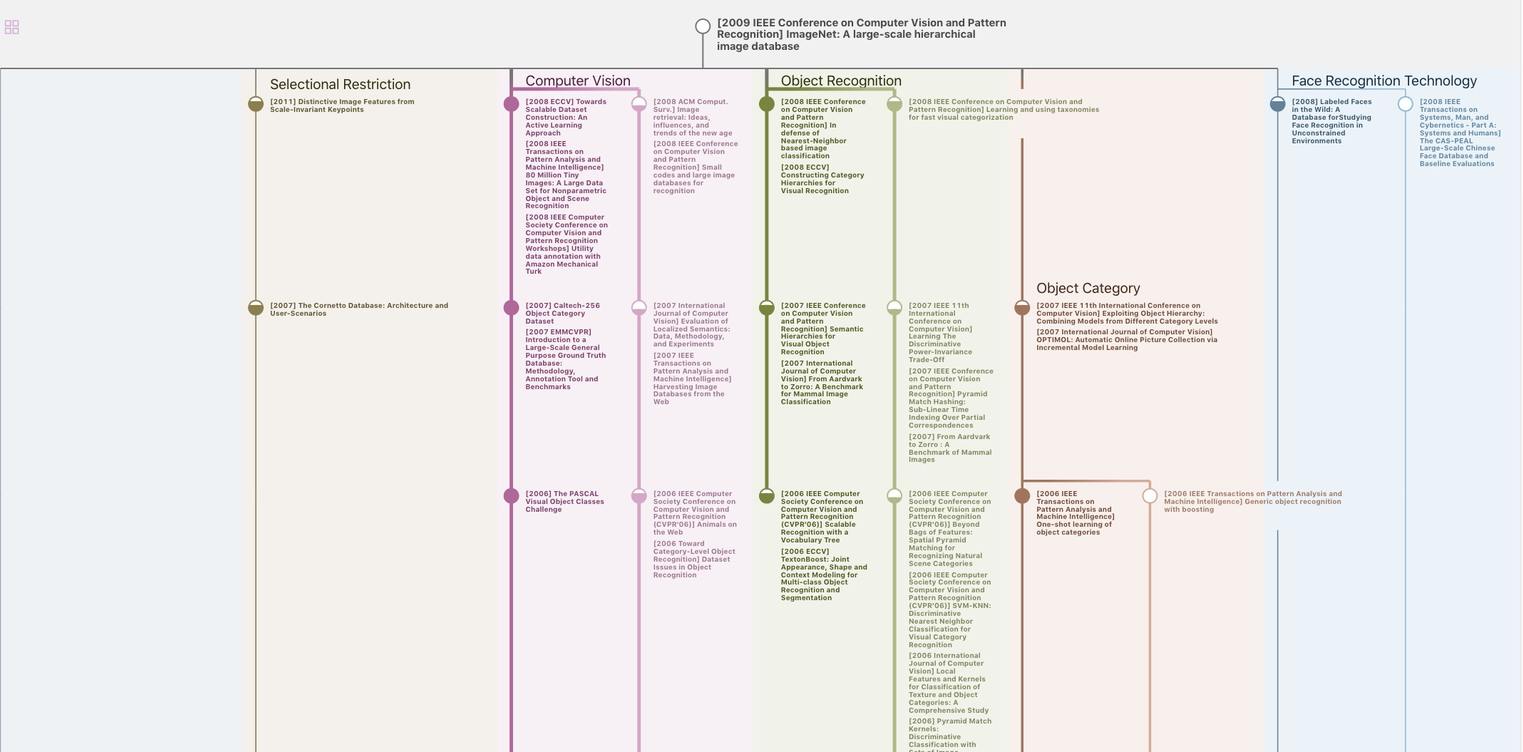
生成溯源树,研究论文发展脉络
Chat Paper
正在生成论文摘要