TIP: Task-Informed Motion Prediction for Intelligent Vehicles.
IEEE/RJS International Conference on Intelligent RObots and Systems (IROS)(2022)
摘要
When predicting trajectories of road agents, motion predictors often approximate the future distribution by a limited number of samples. This constraint requires the predictors to generate samples that best support the task given task specifications. However, existing predictors are often optimized and evaluated via task-agnostic measures without accounting for the use of predictions in downstream tasks, and thus could result in sub-optimal task performance. In this paper, we propose a task-informed motion prediction model that better supports the tasks through its predictions by jointly reasoning about prediction accuracy and the utility of the downstream tasks during training. The task utility function is commonly used to evaluate task performance. It does not require the full task information, but rather a specification of the utility of the task, resulting in predictors that are tailored to different downstream tasks. We demonstrate our approach on two use cases of common decision making tasks and their utility functions, in the context of autonomous driving and parallel autonomy. Experiment results show that our predictor produces accurate predictions that improve the task performance by a large margin in both tasks when compared to task-agnostic baselines on the Waymo Open Motion dataset.
更多查看译文
关键词
motion prediction,vehicles,task-informed
AI 理解论文
溯源树
样例
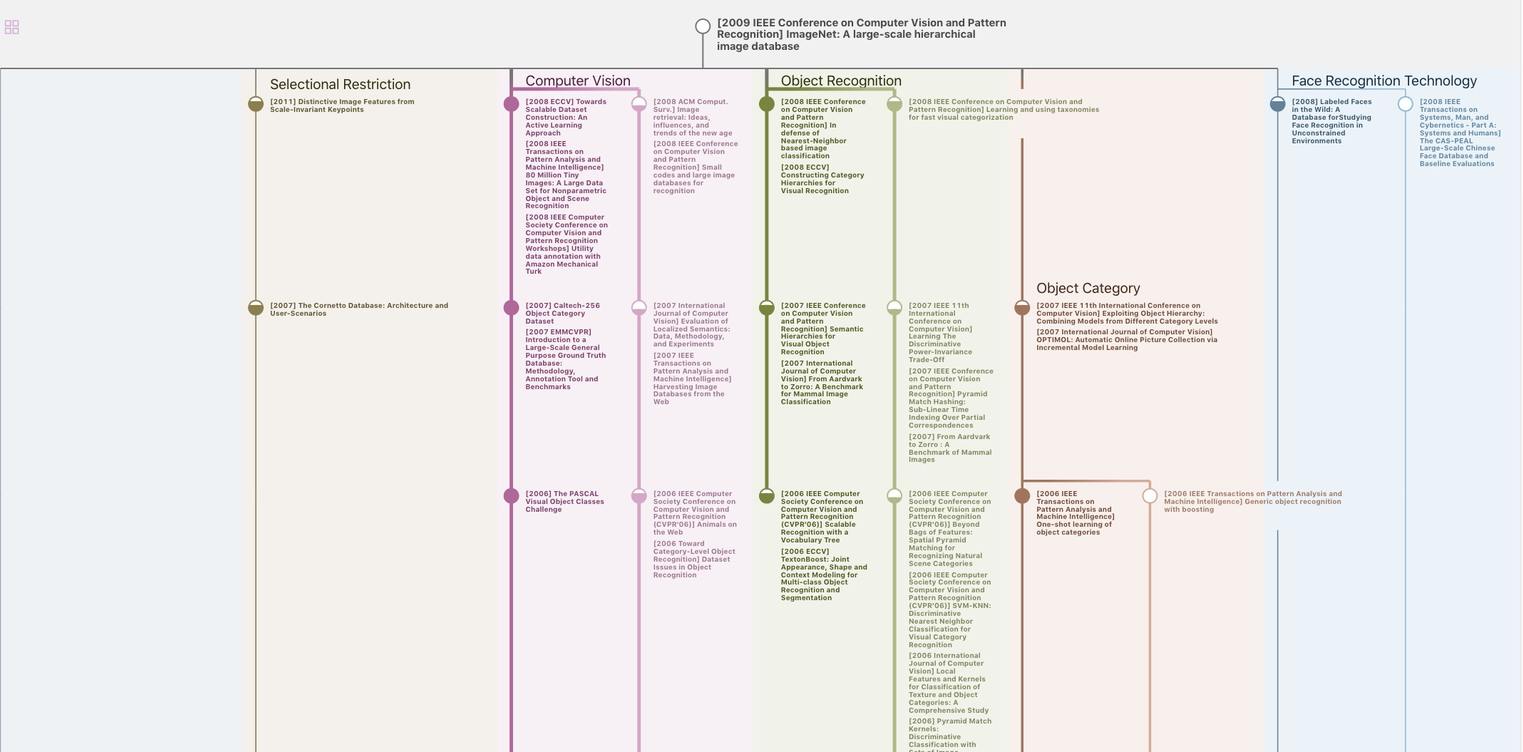
生成溯源树,研究论文发展脉络
Chat Paper
正在生成论文摘要