Linear chain conditional random fields, hidden Markov models, and related classifiers
arxiv(2023)
摘要
Practitioners use Hidden Markov Models (HMMs) in different problems for about sixty years. Besides, Conditional Random Fields (CRFs) are an alternative to HMMs and appear in the literature as different and somewhat concurrent models. We propose two contributions. First, we show that basic Linear-Chain CRFs (LC-CRFs), considered as different from the HMMs, are in fact equivalent to them in the sense that for each LC-CRF there exists a HMM - that we specify - whom posterior distribution is identical to the given LC-CRF. Second, we show that it is possible to reformulate the generative Bayesian classifiers Maximum Posterior Mode (MPM) and Maximum a Posteriori (MAP) used in HMMs, as discriminative ones. The last point is of importance in many fields, especially in Natural Language Processing (NLP), as it shows that in some situations dropping HMMs in favor of CRFs was not necessary.
更多查看译文
关键词
conditional random fields,classifiers,markov models
AI 理解论文
溯源树
样例
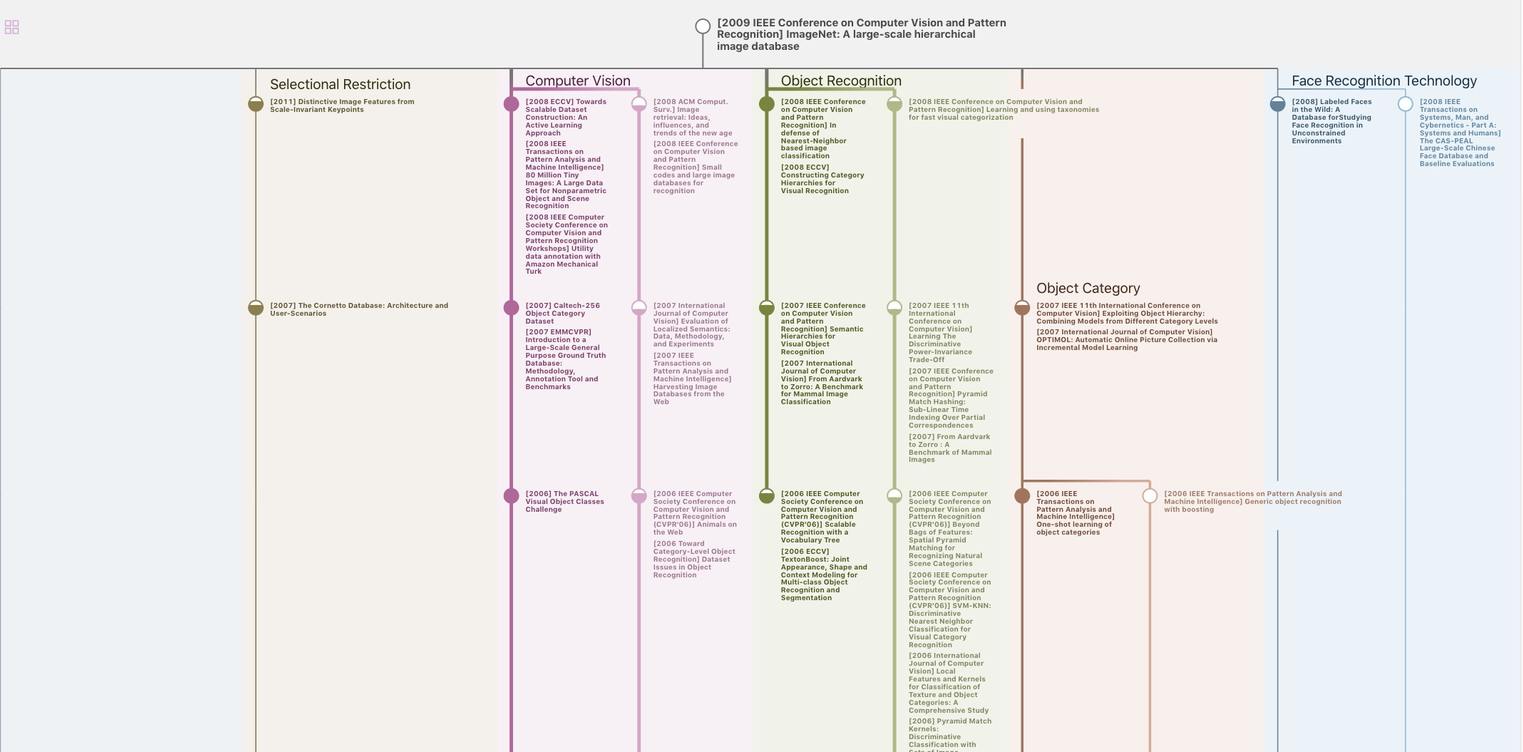
生成溯源树,研究论文发展脉络
Chat Paper
正在生成论文摘要