Heterogeneous domain adaptation and equipment matching: DANN-based Alignment with Cyclic Supervision (DBACS)
COMPUTERS & INDUSTRIAL ENGINEERING(2024)
摘要
Process monitoring and control are essential in modern industries for ensuring high quality standards and optimizing production performance. These technologies have a long history of application in production and have had numerous positive impacts, but also hold great potential when integrated with Industry 4.0 and advanced machine learning, particularly deep learning, solutions. However, in order to implement these solutions in production and enable widespread adoption, the scalability and transferability of deep learning methods have become a focus of research. While transfer learning has proven successful in many cases, particularly with computer vision and homogeneous data inputs, it can be challenging to apply to heterogeneous data. Motivated by the need to transfer and standardize established processes to different, non-identical environments and by the challenge of adapting to heterogeneous data representations, this work introduces the Domain Adaptation Neural Network with Cyclic Supervision (DBACS) approach. DBACS addresses the issue of model generalization through domain adaptation, specifically for heterogeneous data, and enables the transfer and scalability of deep learning-based statistical control methods in a general manner. Additionally, the cyclic interactions between the different parts of the model enable DBACS to not only adapt to the domains, but also match them. To the best of our knowledge, DBACS is the first deep learning approach to combine adaptation and matching for heterogeneous data settings. For comparison, this work also describes and analyzes subspace alignment and a multi-view learning method that deals with heterogeneous representations, called views, by mapping data into correlated latent feature spaces. Finally, the DBACS method, with its ability to adapt and match, is applied to a virtual metrology use case for an etching process run on different machine types in semiconductor manufacturing.
更多查看译文
关键词
Deep learning,Equipment matching,Heterogeneous domain adaptation,Multi-view learning,Semiconductor manufacturing,Virtual metrology
AI 理解论文
溯源树
样例
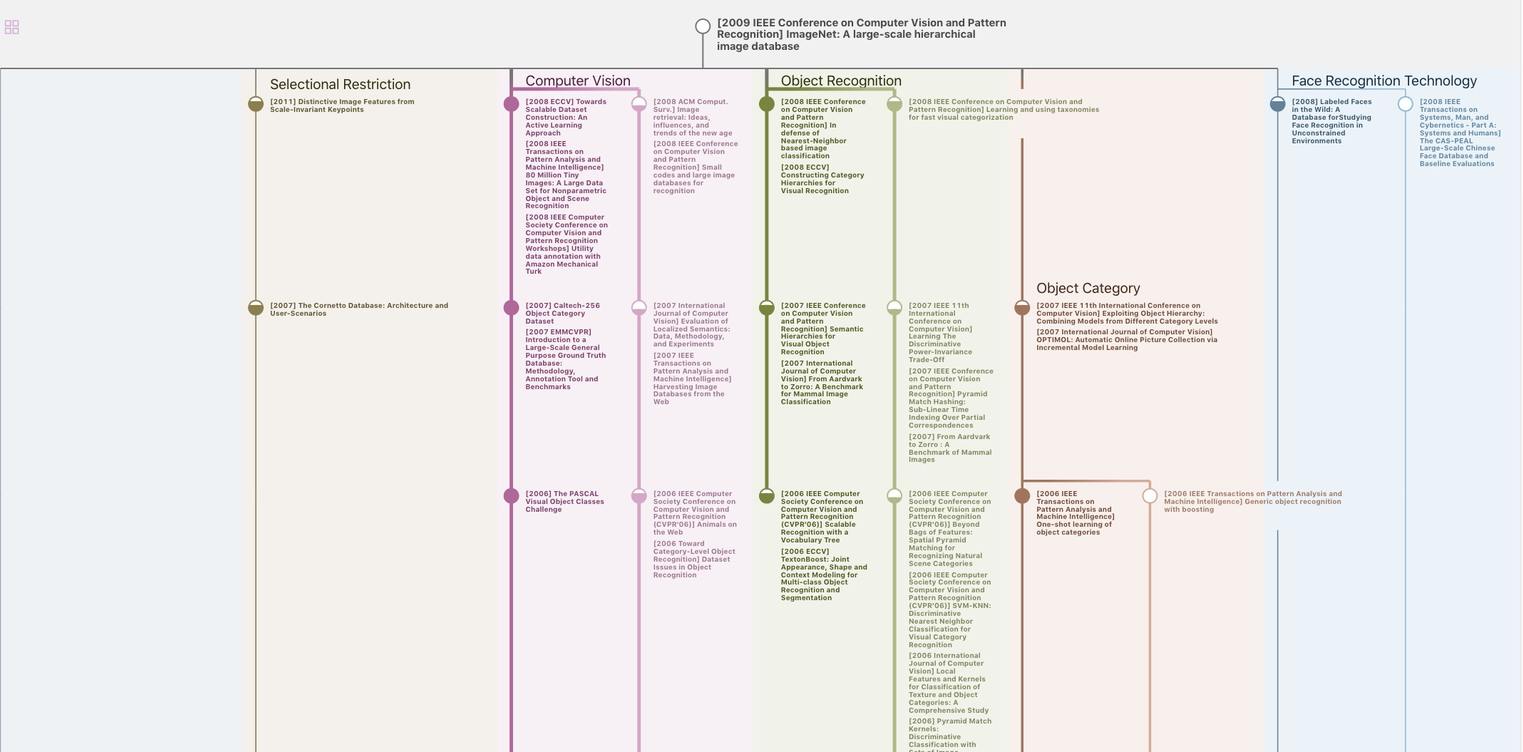
生成溯源树,研究论文发展脉络
Chat Paper
正在生成论文摘要