Explicitly Solvable Continuous-Time Inference for Partially Observed Markov Processes.
IEEE Trans. Signal Process.(2022)
摘要
Many natural and engineered systems can be modeled as discrete state Markov processes. Often, only a subset of states are directly observable. Inferring the conditional probability that a system occupies a particular hidden state, given the partial observation, is a problem with broad application. In this paper, we introduce a continuous-time formulation of the sum-product algorithm, which is a well-known discrete-time method for finding the hidden states' conditional probabilities, given a set of finite, discrete-time observations. From our new formulation, we can explicitly solve for the conditional probability of occupying any state, given the transition rates and observations within a finite time window. We apply our algorithm to a realistic model of the cystic fibrosis transmembrane conductance regulator (CFTR) protein for exact inference of the conditional occupancy probability, given a finite time series of partial observations.
更多查看译文
关键词
Markov processes,Signal processing algorithms,Sum product algorithm,Inference algorithms,Hidden Markov models,Biological system modeling,Message passing,Biomedical signal processing,differential equations,hidden markov models,inference algorithms
AI 理解论文
溯源树
样例
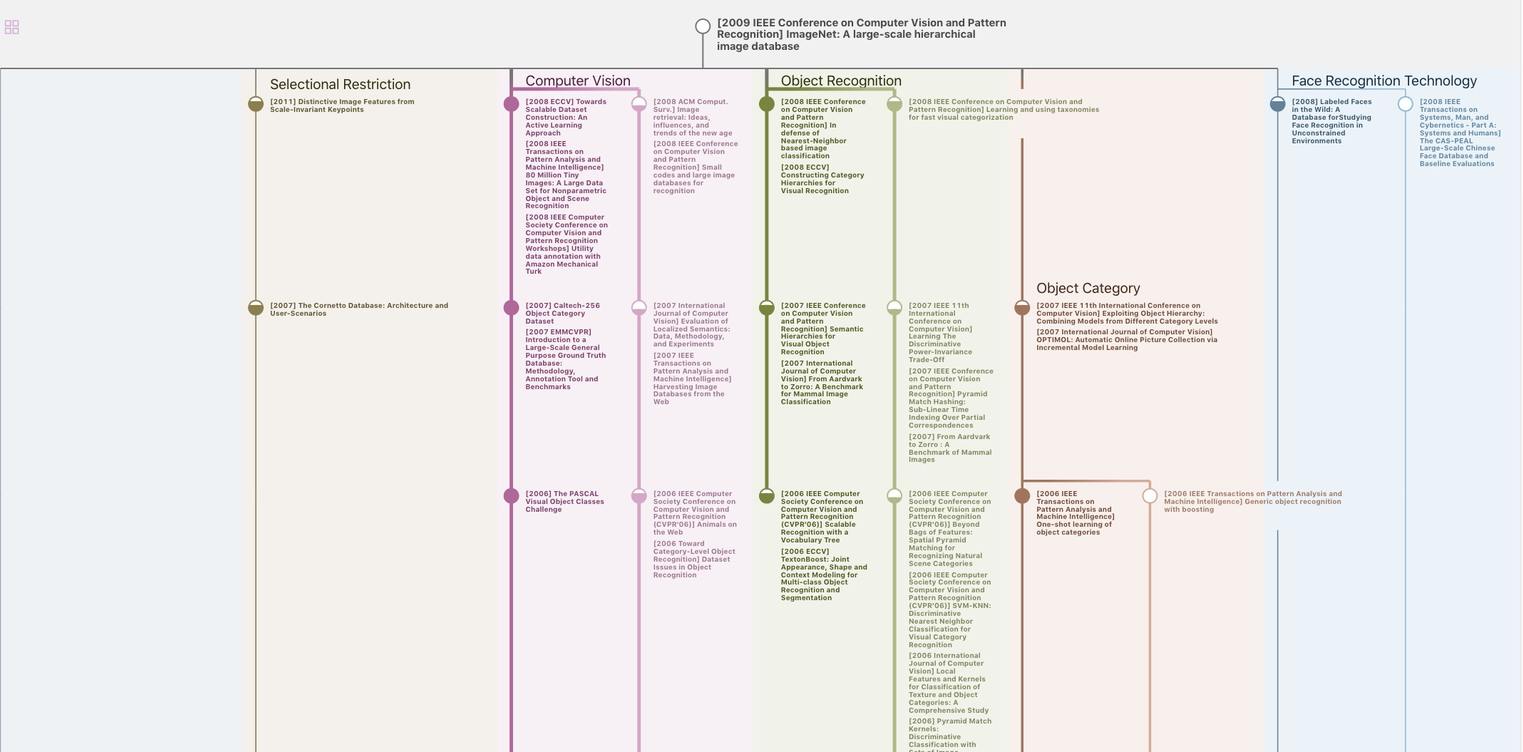
生成溯源树,研究论文发展脉络
Chat Paper
正在生成论文摘要