On the Effects of Properties of the Minibatch in Reinforcement Learning.
International Conference on Intelligent Technologies and Applications (INTAP)(2021)
摘要
Neural networks are typically trained on large amounts of data using a gradient descent optimization algorithm. With large quantities of data it is infeasible to calculate the gradient over the entire dataset, and the gradient is therefore estimated over smaller minibatches of data. Conventional wisdom in deep learning dictates that best performance is achieved when each minibatch is representative of the whole dataset, which is typically approximated by uniform random sampling from the dataset. In deep reinforcement learning the agent being optimized and the data are intimately linked, as the agent often chooses its own traversal of the problem space (and therefore data generation), and further the objective is not necessarily to perform optimally over the whole problem space but rather to identify the high rewarding regions and how to reach them. In this paper we hypothesize that one can train specifically for subregions of the problem space by constructing minibatches with data exclusively from this subregion, or conversely that one can avoid catastrophic forgetting by ensuring that each minibatch is representative of the whole dataset. We further investigate the effects of applying such a strategy throughout the training process in the offline reinforcement learning setting. We find that specific training in this sense is not possible with the suggested approach, and that simple random uniform sampling performs comparable or better than the suggested approach in all cases tested.
更多查看译文
关键词
minibatch,reinforcement learning,properties
AI 理解论文
溯源树
样例
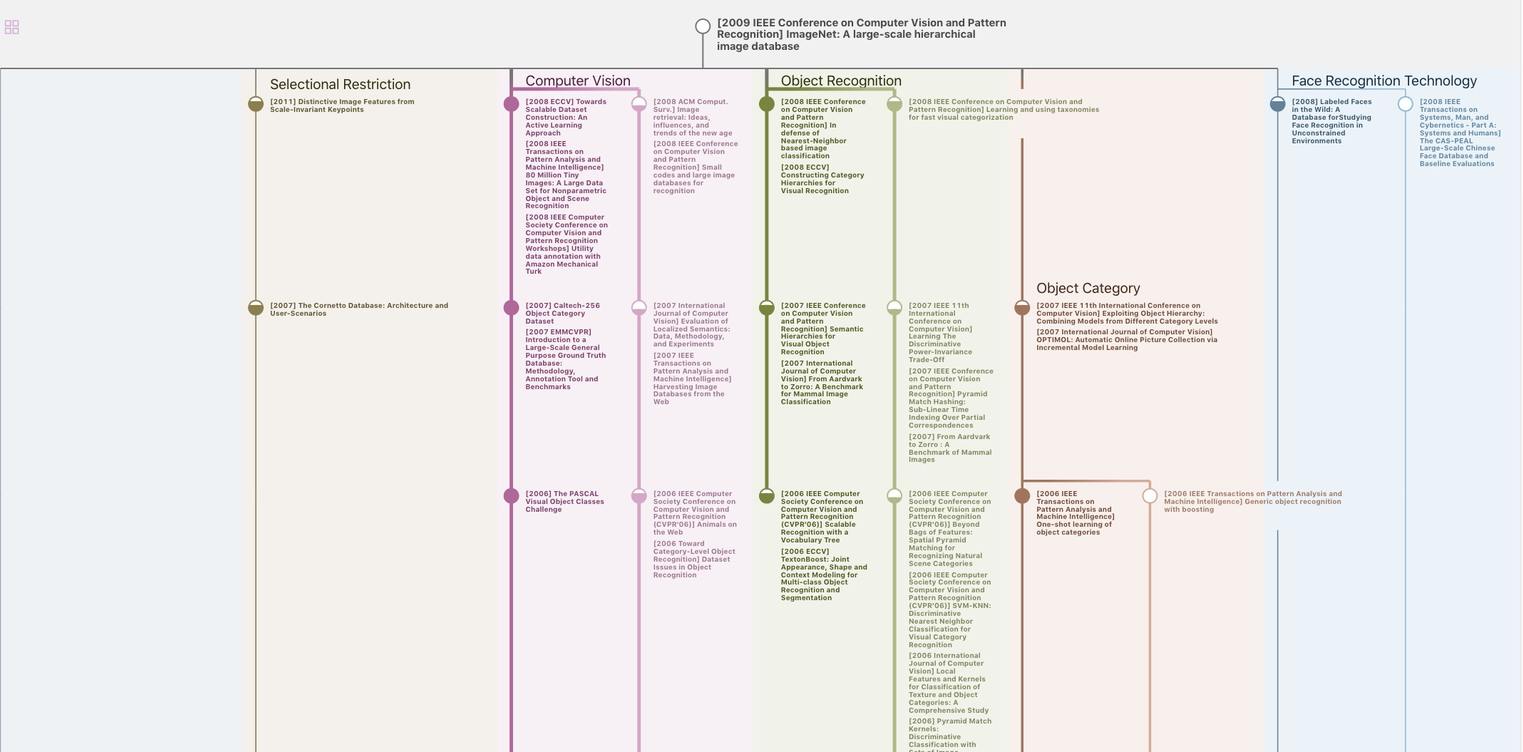
生成溯源树,研究论文发展脉络
Chat Paper
正在生成论文摘要