Generating Local Textual Explanations for CNNs: A Semantic Approach Based on Knowledge Graphs.
International Conference of the Italian Association for Artificial Intelligence (AI*IA)(2021)
摘要
Explainable Artificial Intelligence (XAI) has recently become an active research field due to the need for transparency and accountability when deploying AI models for high-stake decision making. In Computer Vision, despite state-of-the-art Convolutional Neural Networks (CNNs) have achieved great performance, understanding their decision processes, especially when a mistake occur, is still a known challenge. Current XAI methods for explaining CNNs mostly rely on visually highlighting parts of the image that contributed the most to the outcome. Although helpful, such visual clues do not provide a deeper understanding of the neural representation and need to be interpreted by humans. This limits scalability and possibly adds bias to the explainability process, in particular when the outcome is not the one expected. In this paper, we propose a method that provides textual explanations for CNNs in image classification tasks. The explanations generated by our approach can be easily understood by humans, which makes our method more scalable and less dependent on human interpretation. In addition, our approach gives the opportunity to link neural representations with knowledge. In the proposed approach we extend our notion of co-activation graph to include input data and we use such graph to connect neural representations from trained CNNs with external knowledge. Then, we use link prediction algorithms to predict semantic attributes of unseen input data. Finally, we use the results of these predictions to generate factual and counterfactual textual explanations of classification mistakes. Preliminary results show that when the link prediction accuracy is high, our method can generate good textual factual and counterfactual explanations that do not need human interpretation. Despite a more extensive evaluation is still ongoing, this indicates the potential of our approach in combining neural representations and knowledge graphs to generate explanations for mistakes in semantic terms.
更多查看译文
关键词
Explainable AI,Knowledge graphs,Deep representation learning,Computer vision
AI 理解论文
溯源树
样例
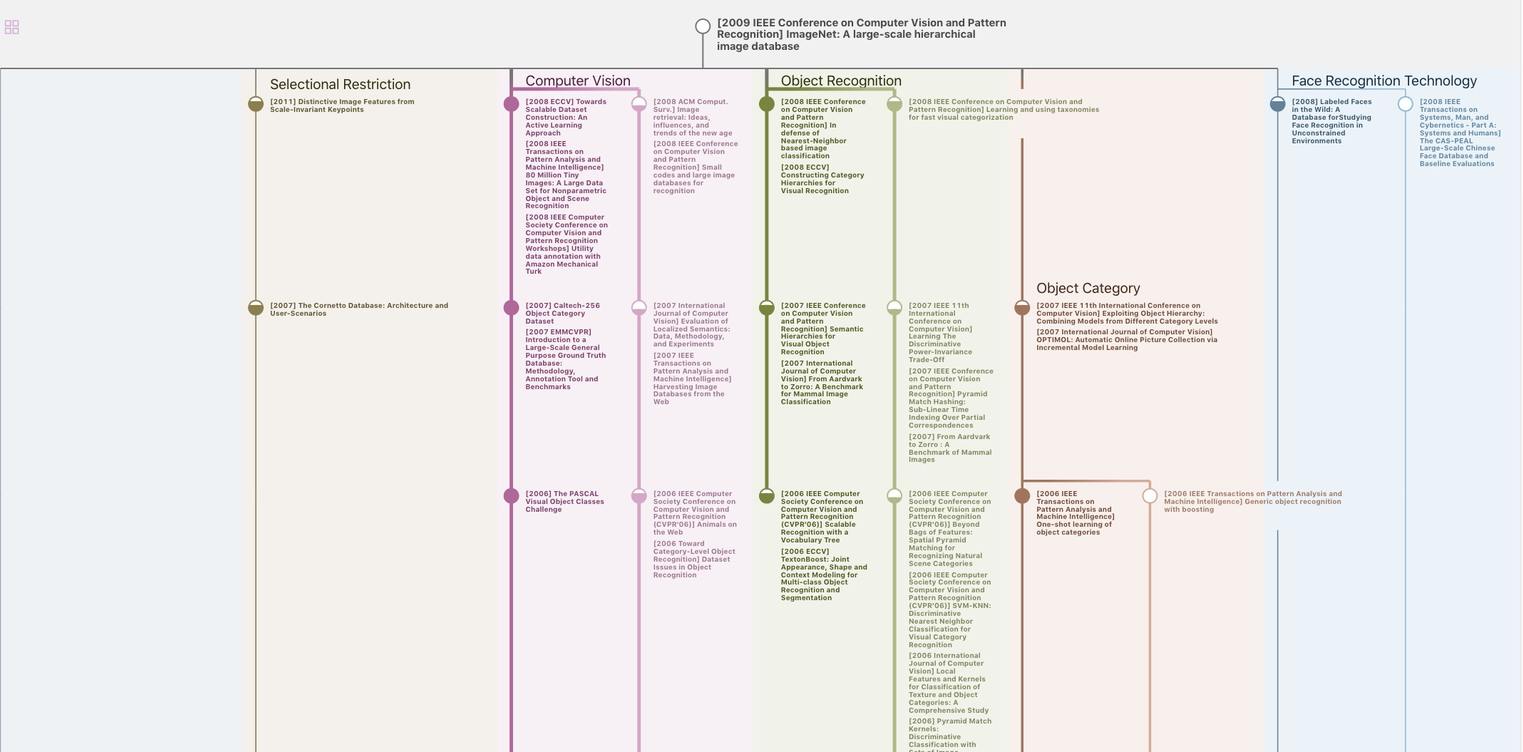
生成溯源树,研究论文发展脉络
Chat Paper
正在生成论文摘要