Bioactivity Predictors for the Inhibition of Staphylococcus Aureus Quinolone Resistance Protein
Applied Computer Sciences in Engineering(2022)
摘要
Antibiotic resistance is a problem that has been increasing in recent years due to the inappropriate use of antibiotics. However, more techniques to design new medicines are employed frequently nowadays. In addition, the application of artificial intelligence tools in discovering new drugs has proven to be a possible solution to this problem. This paper aims to show and analyze the results obtained from the use of machine learning techniques when two different sets of features: i) constructed from Lipinski’s rules of five, and ii) fingerprints from biomolecular sequences, were used. Six regressors were implemented to predict the minimum inhibitory concentration (MIC) valuer to generate models that allow the identification of possible drugs. A specific case for inhibition of the Staphylococcus Aureus and its protein NorA was studied in problems associated to Quinolone antibiotic resistance. A dataset of 187 sequences extracted from ChEmbl repository was used for this purpose. The results show that both Lipinski rules and fingerprints were favorable for generation models that fit actual MIC values of the molecules. The feature sets used and the regressors selected allowed generating models that can predict the bioactivity of a molecule, constituting a tool that could be valuable in the generation of new antibiotics to combat the problem addressed.
更多查看译文
关键词
Fingerprint, Lipinski rules, Machine learning, Protein, Regression, Sequence, Staphylococcus aureus
AI 理解论文
溯源树
样例
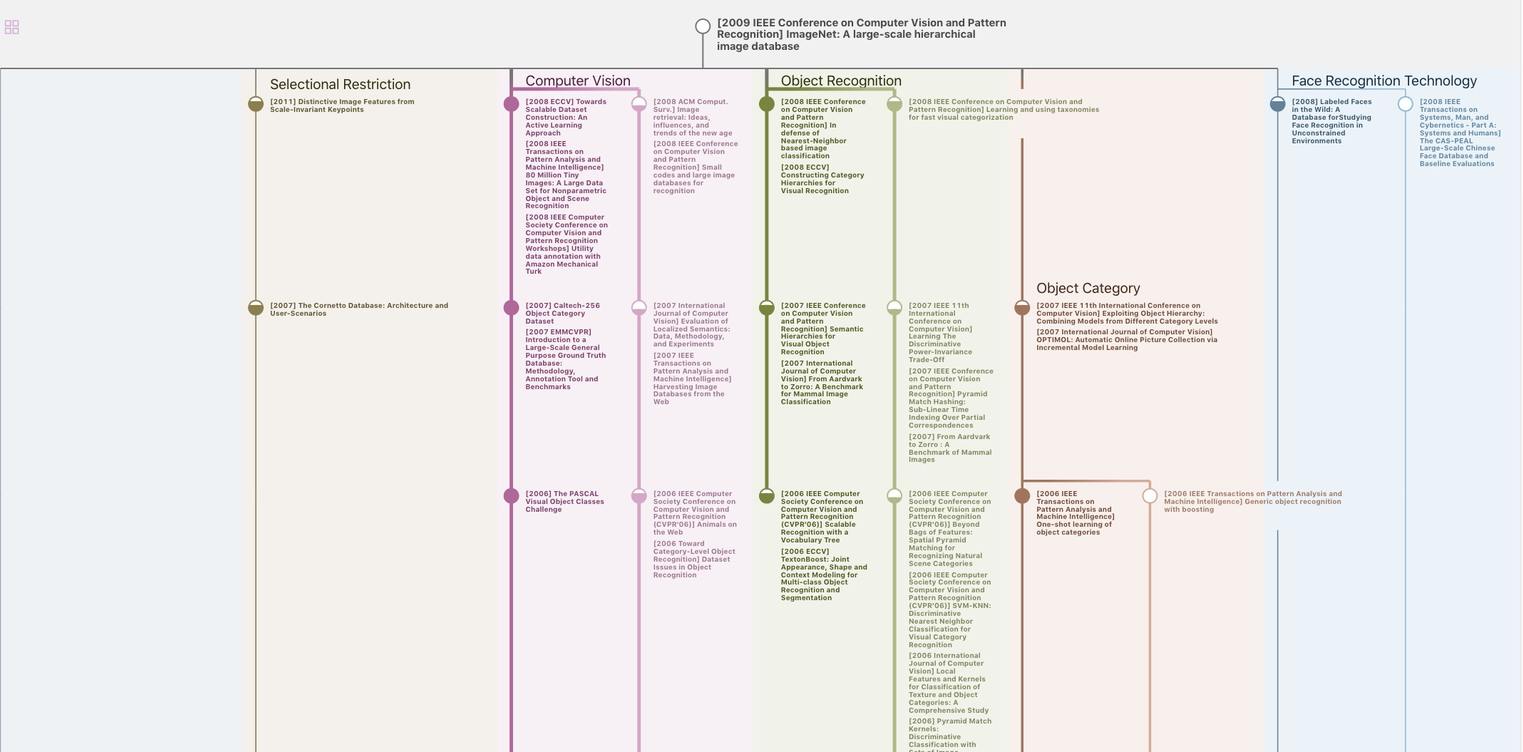
生成溯源树,研究论文发展脉络
Chat Paper
正在生成论文摘要