Deep Learning-Enhanced MHC-II Presentation Prediction and Peptidome Deconvolution
Bioinformatics Research and Applications(2023)
摘要
Antigen-presenting cells can elicit a CD4
$$^+$$
T cell response by displaying foreign peptides on the surface. Identifying such peptides requires robust prediction of the binding and presentation corresponding to peptides and major histocompatibility complexes class II (MHC-II) molecules. However, numerous experimental data suffer from inexact supervision, and the open conformation of MHC-II molecules leads to a complex peptide binding pattern. Though current prediction methods have significantly pushed the development of cancer vaccines and immunotherapies, an urgent desire for better approaches still exists. We practice the powerful multi-head self-attention technique for MHC-II-restricted peptidome deconvolution and antigen presentation prediction problems. According to binding motifs reflected by eluted ligands, the novel expert voting-based deconvolution strategy ensures a reliable MHC-II assignment. Driven by massive trusty annotated peptidome data, our method overwhelms the start-of-the-art MHC-II presentation prediction method, NetMHCIIpan4.0, on two independent single allelic datasets. All these results have demonstrated that our method can boost the performance of MHC-II presentation prediction and peptidome deconvolution.
更多查看译文
关键词
Antigen presentation,CD4 T cells,Deep learning,Deconvolution
AI 理解论文
溯源树
样例
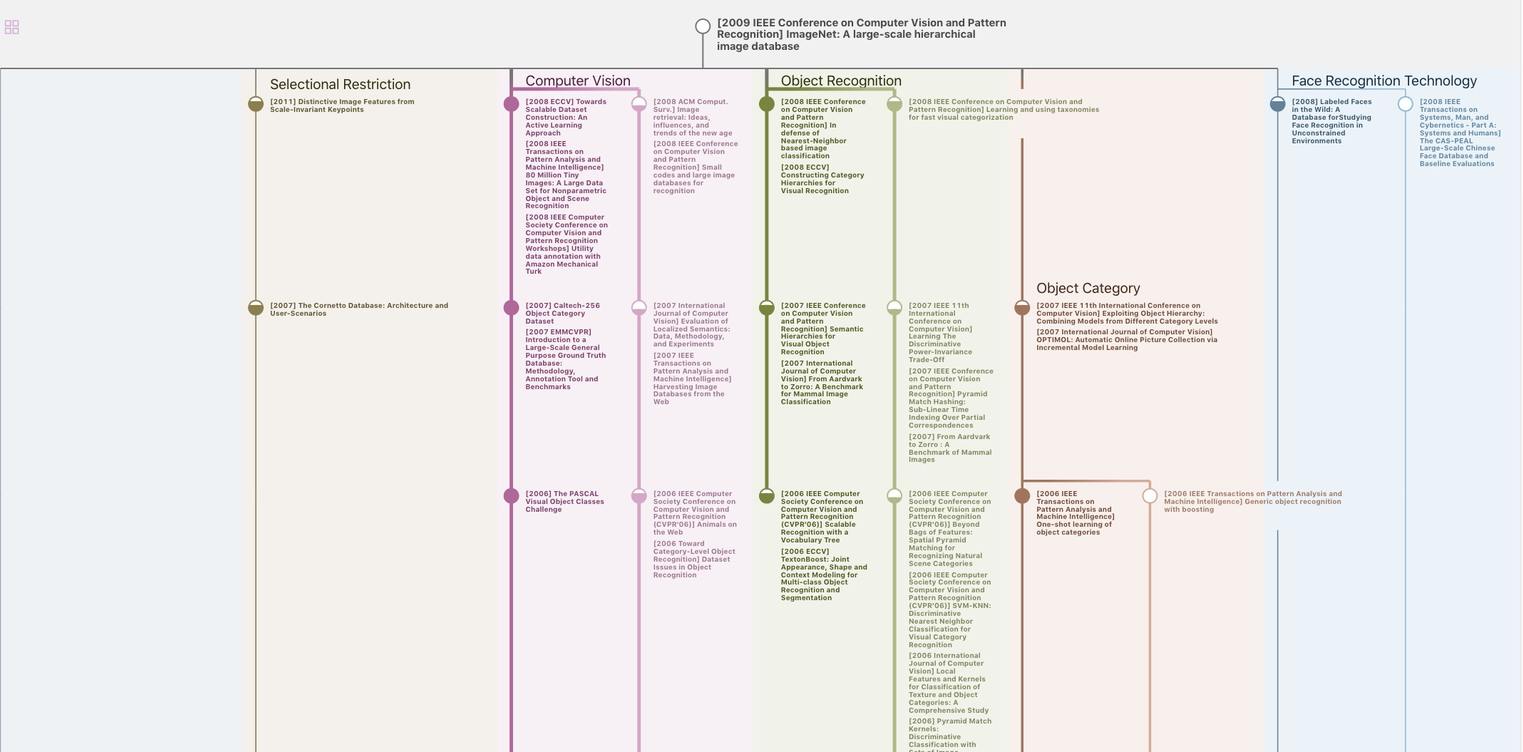
生成溯源树,研究论文发展脉络
Chat Paper
正在生成论文摘要