Context-Aware Completion Time Prediction for Business Process Monitoring.
WorldCIST(2022)
摘要
Time-based prediction problems are often modeled using machine learning. In business process monitoring, we associate time-based prediction tasks with predictive process monitoring goals. Solutions for prediction are based on typical pieces of information recorded in an event log related to business processes, such as timestamps and execution of activities. However, relevant characteristics about the business process are left out when we select only these attributes. In this context, we state the use of process contextual data should provide relevant information to improve predictions. In this paper, we discuss the completion time prediction problem by manually selecting and adding contextual process instance attributes into the description of process instances before input them to prediction models implemented using LSTM neural network and Annotated Transition Systems. Our approach focuses on how an attribute influences the completion time of existing cases, and how they affect the prediction performance. We evaluated our approach using real-world event logs and compared them with baseline predictions. The results showed predictions models trained using contextual attributes performed better, improving prediction response by up to 83%.
更多查看译文
关键词
Predictive process monitoring,Context-aware prediction,Completion time prediction,Process mining,Machine learning
AI 理解论文
溯源树
样例
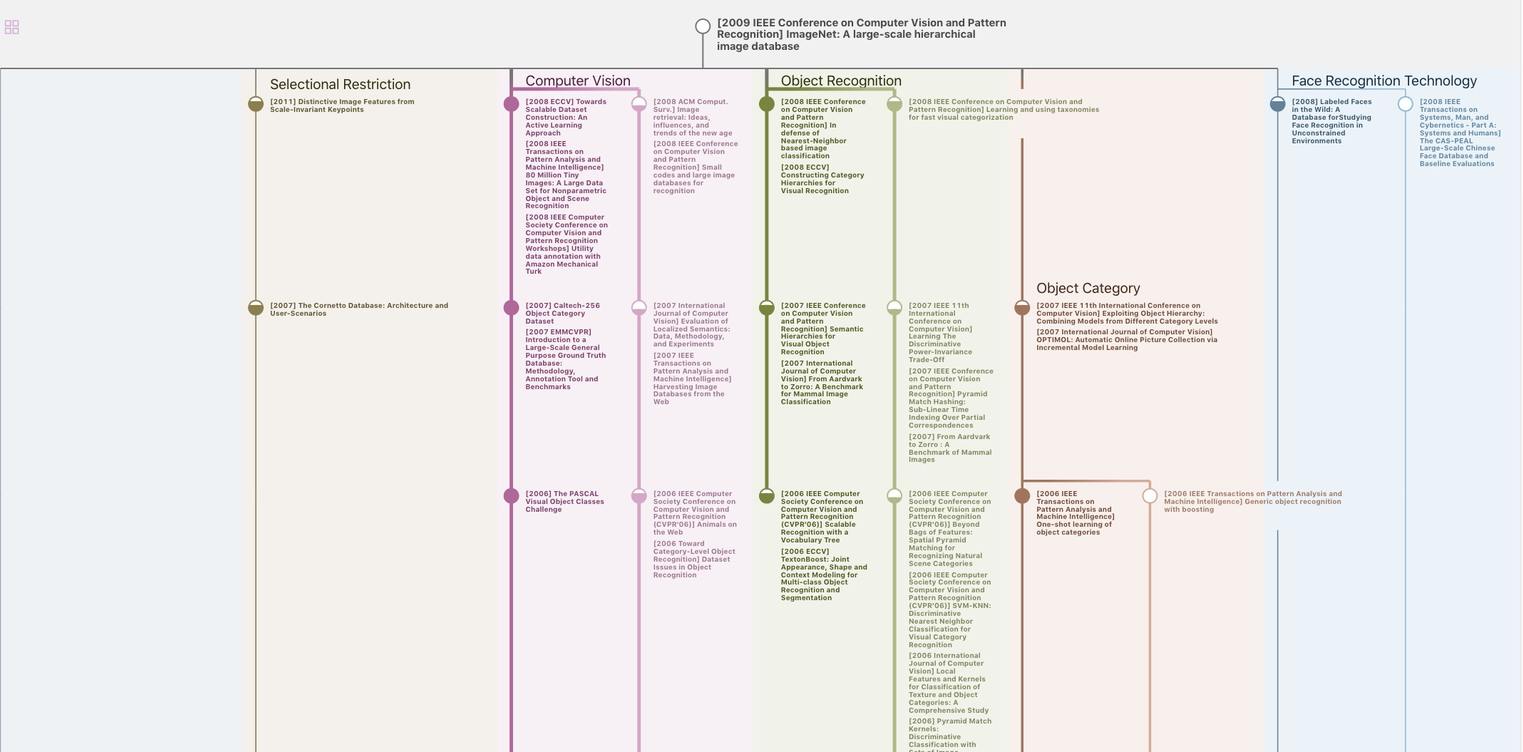
生成溯源树,研究论文发展脉络
Chat Paper
正在生成论文摘要