A Machine Learning-Driven Analysis of Phantom E911 Calls.
Wireless Network Security (WISEC)(2022)
摘要
Phantom Enhanced 911 (E911) calls are automatically generated 2 second calls, are a serious concern on cellular networks, and consume critical resources. As networks become increasingly complex, detecting and troubleshooting the causes of phantom E911 calls is becoming increasingly difficult. In this paper machine learning (ML) tools are used to analyze anonymized call detail record data collected by a major US telecom network service provider. The data is carefully pre-processed and encoded using an efficient encoding method. Classification algorithms K Nearest Neighbors (KNN) and Decision Trees (DTs) are then implemented to study correlations between device and network level features and a mobile device's ability to initiate phantom calls. Based on the results, this work also suggests certain policy changes for network operators that may decrease the high volume of phantom E911 calls or alleviate the pressure of phantom E911 calls on a cellular network.
更多查看译文
关键词
Data Encoding,Decision Tree,Diagnosis,Feature Engineering,KNearest Neighbors,Machine Learning,Transductive Learning
AI 理解论文
溯源树
样例
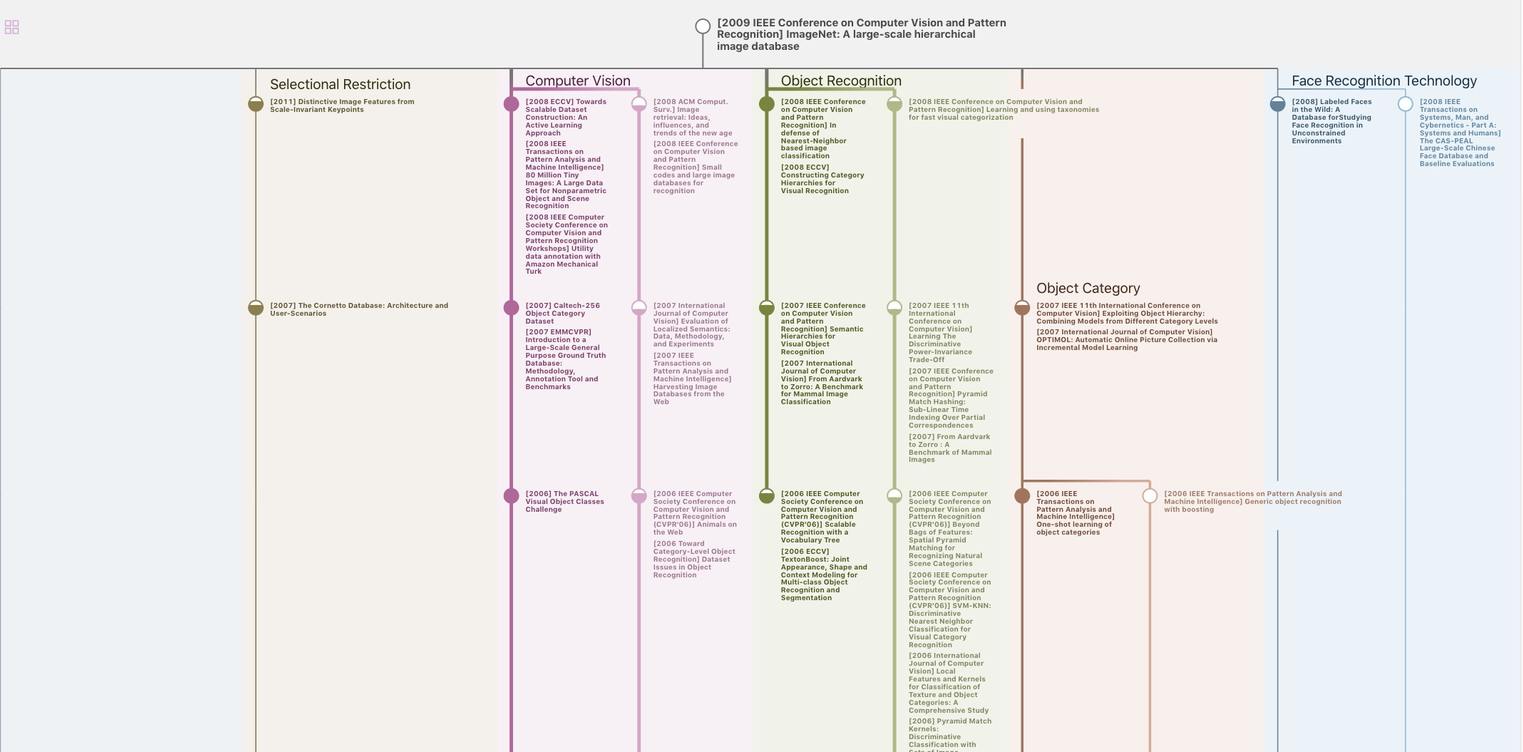
生成溯源树,研究论文发展脉络
Chat Paper
正在生成论文摘要