Machine Learning-Based Decision Making in Evolutionary Multiobjective Clustering.
Mexican International Conference on Artificial Intelligence (MICAI)(2022)
摘要
Clustering is an important optimization problem which is at the core of many data mining and machine learning applications. Evolutionary multiobjective clustering algorithms optimize multiple partition quality criteria simultaneously, producing a set of trade-off solutions in a single execution. How can a final solution be selected from this set of candidate trade-offs? Decision making is concerned with such a task, acknowledging that obtaining a single solution may represent the ultimate goal in practice. Given the limitations of current techniques, we explore a novel approach to decision making: tackling this task as a supervised learning problem. Our approach attempts to learn from the decision-making process in example (training) problems, with the aim of later applying the learned model to lead the identification of final solutions in new applications. In this paper, we present preliminary results on the evaluation of this proposal. Our experiments include comparisons with respect to a set of decision-making approaches from the literature and consider a collection of synthetic clustering problems with diverse characteristics. The results obtained are promising, highlighting the relevance of devising alternative decision-making strategies, the effectiveness of our proposal, and motivating further research on this matter.
更多查看译文
关键词
clustering,decision making,learning-based
AI 理解论文
溯源树
样例
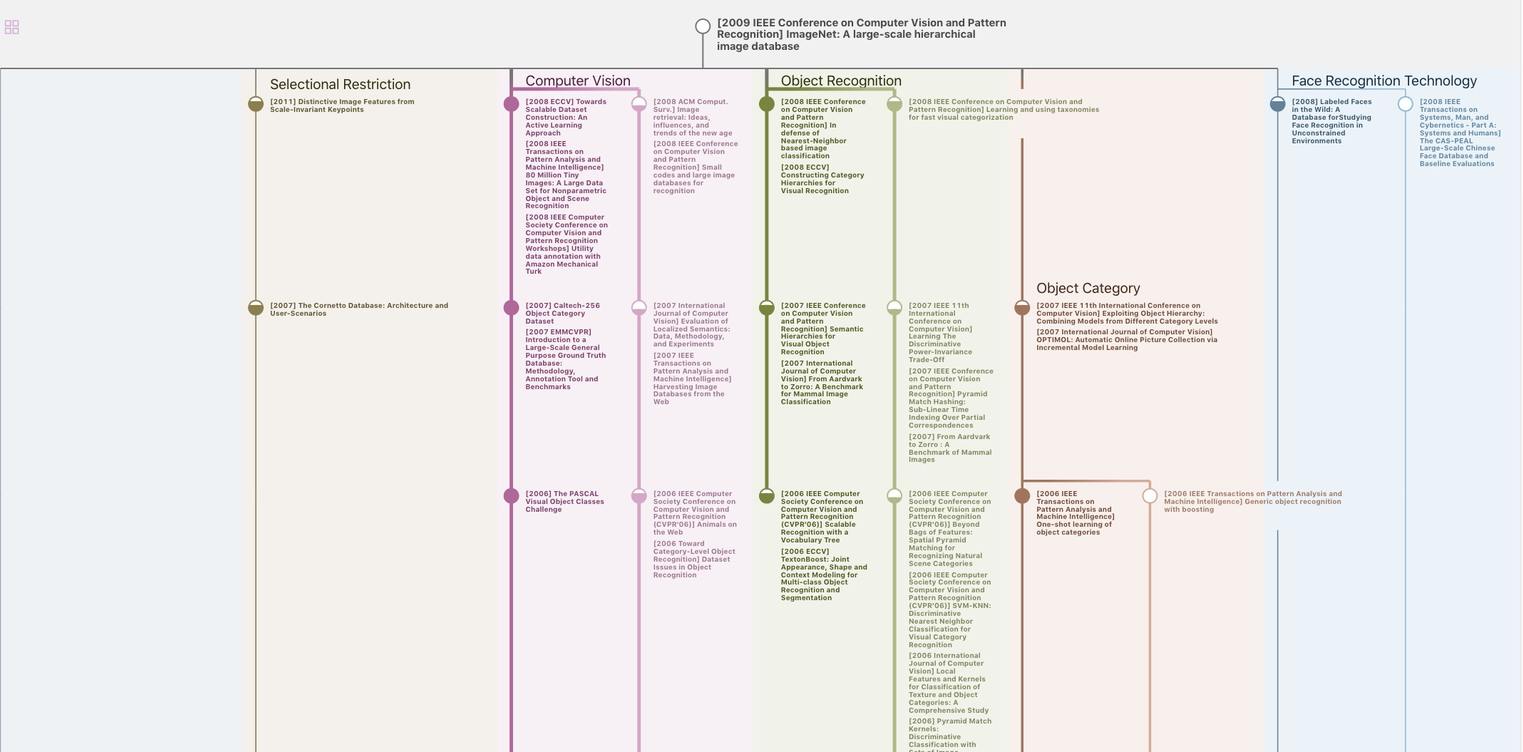
生成溯源树,研究论文发展脉络
Chat Paper
正在生成论文摘要