Neural Estimation of the Rate-Distortion Function for Massive Datasets.
International Symposium on Information Theory (ISIT)(2022)
摘要
A fundamental question in designing lossy data compression schemes is how well one can do in comparison with the rate-distortion function, which describes the known theoretical limits of lossy compression. Motivated by the empirical success of deep neural network (DNN) compressors on large, real-world data, we investigate methods to estimate the rate-distortion function on such data, which would allow comparison of DNN compressors with optimality. While one could use the empirical distribution of the data and apply the Blahut-Arimoto algorithm, this approach presents several computational challenges when the datasets are large and high-dimensional, such as the case of modern image datasets. Instead, we reformulate the rate-distortion objective, and solve the resulting functional optimization problem using neural networks. We provide experimental results on popular image datasets, and provide theoretical evidence why our method can accurately estimate the rate-distortion function. Additionally, we show that the rate-distortion achievable by DNN compressors are within several bits of the rate-distortion function. Lastly, we connect the rate-distortion objective and entropic optimal transport, and describe a method to implement an operational lossy compression scheme with guarantees on the achievable rate and distortion.
更多查看译文
关键词
Lossy compression,rate-distortion theory,neural networks,generative models,optimal transport
AI 理解论文
溯源树
样例
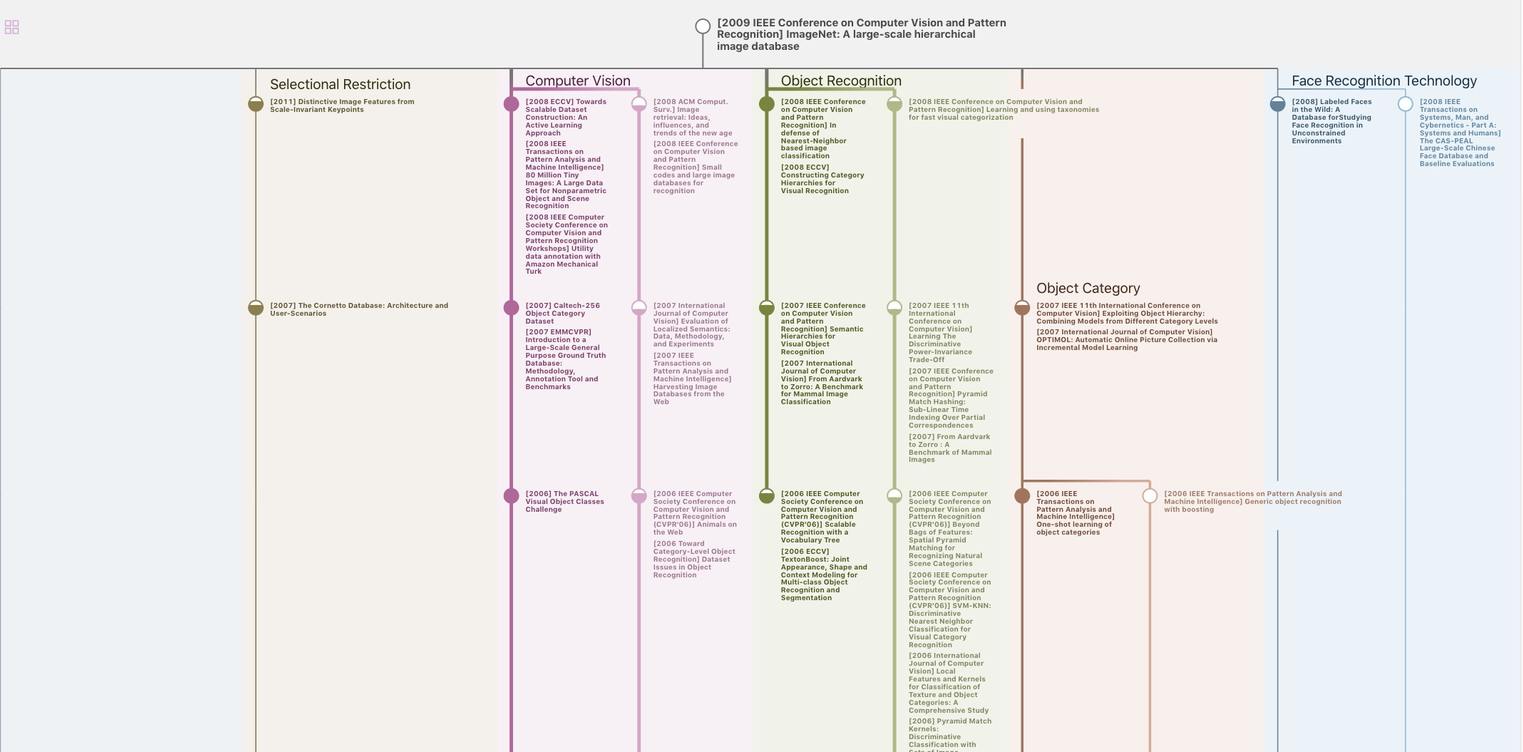
生成溯源树,研究论文发展脉络
Chat Paper
正在生成论文摘要