Predicting fire brigades' operations based on their type of interventions.
International Conference on Wireless Communications and Mobile Computing (IWCMC)(2022)
摘要
Forecasting the number of fire department deployments for different types of operations is important to size the need to the demand and hence, improve emergency response efficiency and reduce financial and material resources. Fire department operations are not considered hazardous because they are somewhat related to time and date. Fires are more likely to occur in the fall than in the winter, and floods are more risky in the winter than in the spring. Car accidents are also logically more likely to occur during the day than at night, when most people are resting at home. This work focuses on predicting the target value of fire calls by creating 14 different subsets of data for each type of possible category (childbirth, fire, suicide, traffic accident, drown, fire on public road, water-flood, heating, emergency aid to people, help for people, public road accident, brawl, witness, and wasp). The methodology was based on the Departmental Fire and Rescue Doubs (SDIS 25) in France, where two machine learning techniques were then implemented to verify the feasibility of the experiments. Although the results can be improved by adding additional explanatory variables, the results were promising.
更多查看译文
关键词
Prediction, XGBoost, LightGBM, firefighters' interventions
AI 理解论文
溯源树
样例
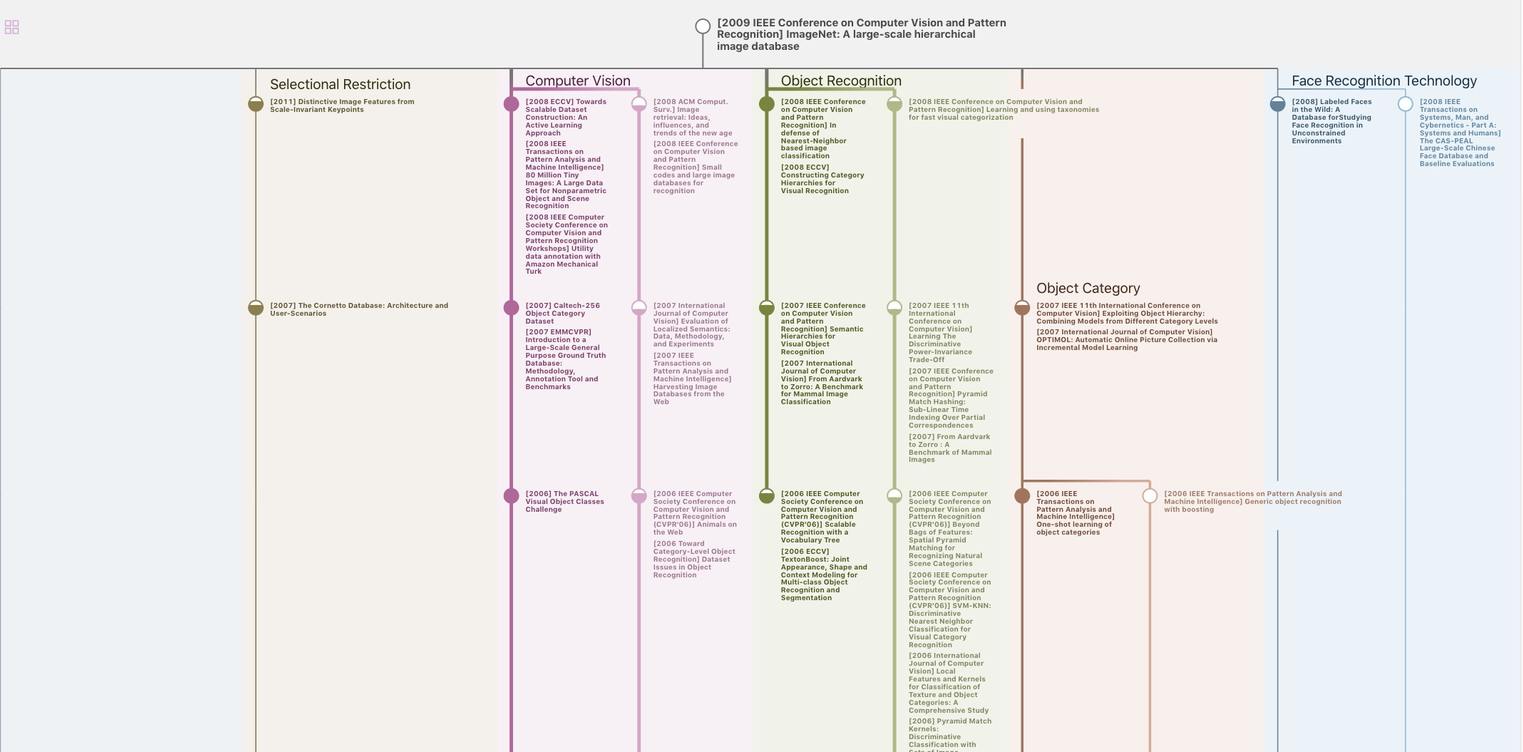
生成溯源树,研究论文发展脉络
Chat Paper
正在生成论文摘要