A Proposal of an Improved Maximum Bayes Boundary-Ness Training Method.
International Conference on Signal Processing and Machine Learning (SPML)(2022)
摘要
One ultimate objective of statistical pattern recognition is to achieve the optimal status that produces Bayes error and its corresponding Bayes boundary. To compute such parameters of pattern recognizers without requiring unrealistically long computation times and strict parameter assumptions, the Maximum Bayes Boundary-ness (MBB) training method was proposed, and its effectiveness was demonstrated. This method focuses on the fact that the posterior probabilities on the Bayes boundary are equal among the classes that comprise the boundary and aim to directly achieve it without estimating the Bayes error. In the MBB training method, a loss function is defined for each sample, its weighted average was defined as an empirical average loss, and its minimization was a training objective. However, the loss is defined slightly excessively using a convex function. In this paper, we demonstrate the high utility of a simplified MBB training that is defined by correcting or simplifying the formalization of conventional MBB training by removing the convex function in the loss. We experimentally compared five datasets and gained speed in most of them.
更多查看译文
AI 理解论文
溯源树
样例
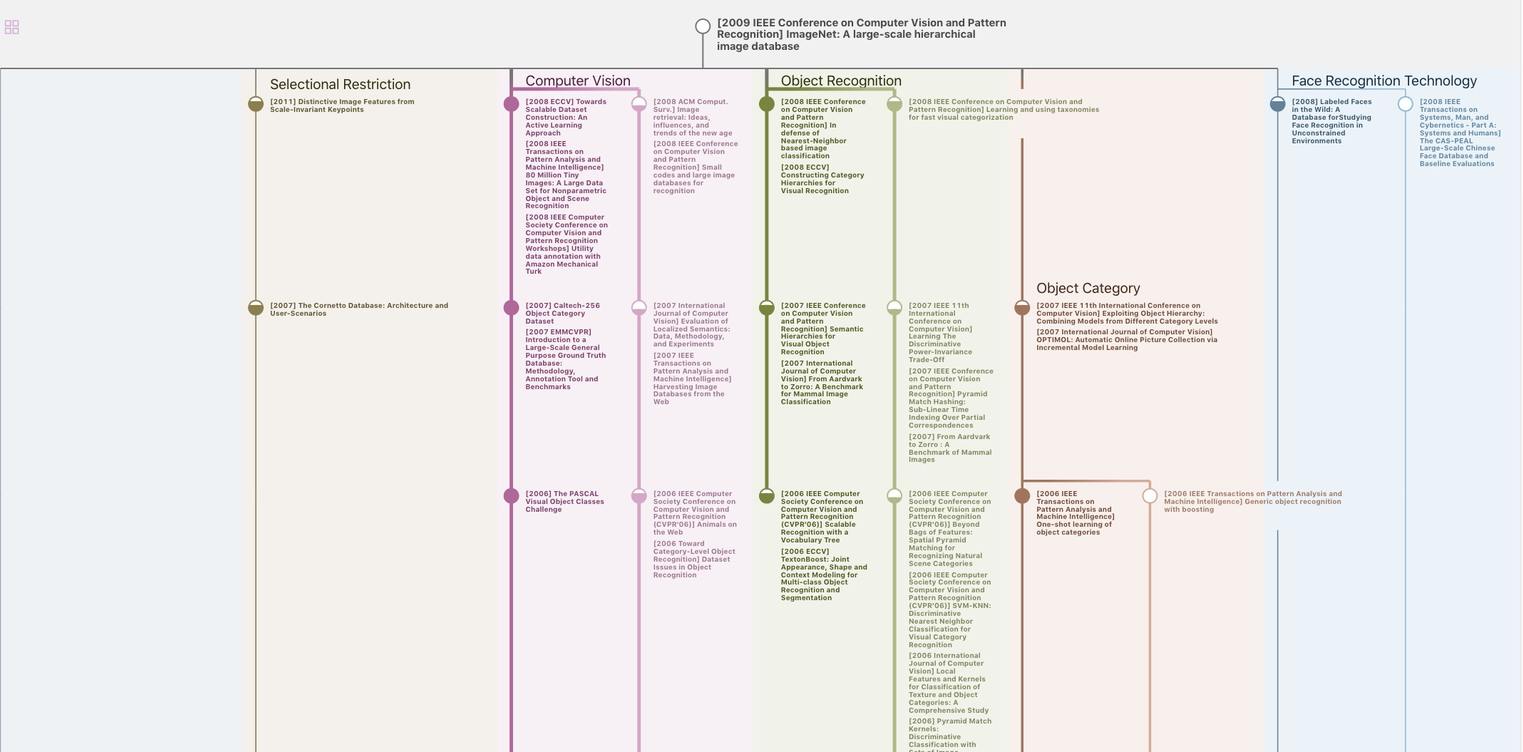
生成溯源树,研究论文发展脉络
Chat Paper
正在生成论文摘要