Towards missing traffic data imputation using attention-based temporal convolutional networks.
International Conference on Intelligent Transportation Systems (ITSC)(2022)
摘要
Measurements from traffic sensors usually go missing at unanticipated moments as a result of detector malfunctioning, communication error, or erratic sampling. These missing data may weaken or even imperil the validity and effectiveness of data-driven traffic applications. The paper proposes a novel deep learning-based missing traffic imputation framework using self-attention based temporal convolutional network (ATCN) to achieve fast extraction of spatio-temporal traffic patterns. To be specific, our proposed ATCNImp model uses an encoder-decoder architecture with one-dimensional convolutional (Conv1D) layer to obtain spatial representations. Additionally, the ATCN module is utilized between the encoder and decoder to capture long-range spatial-temporal dynamics, further enhancing feature presentations and highlighting degradation information under traffic missing settings. Finally, the performance of our ATCNImp is verified using the public PeMS-BAY dataset. Experimental results reveal that ATCNImp outperforms the other four imputation models and provides stable imputation performance.
更多查看译文
关键词
traffic data imputation,attention-based temporal convolutional networks,traffic sensors,unanticipated moments,detector malfunctioning,communication error,erratic sampling,missing data,data-driven traffic applications,deep learning-based,traffic imputation framework,self-attention based temporal convolutional network,fast extraction,spatio-temporal traffic patterns,ATCNImp model,encoder-decoder architecture,one-dimensional convolutional layer,spatial representations,ATCN module,long-range spatial-temporal dynamics,imputation models,stable imputation performance
AI 理解论文
溯源树
样例
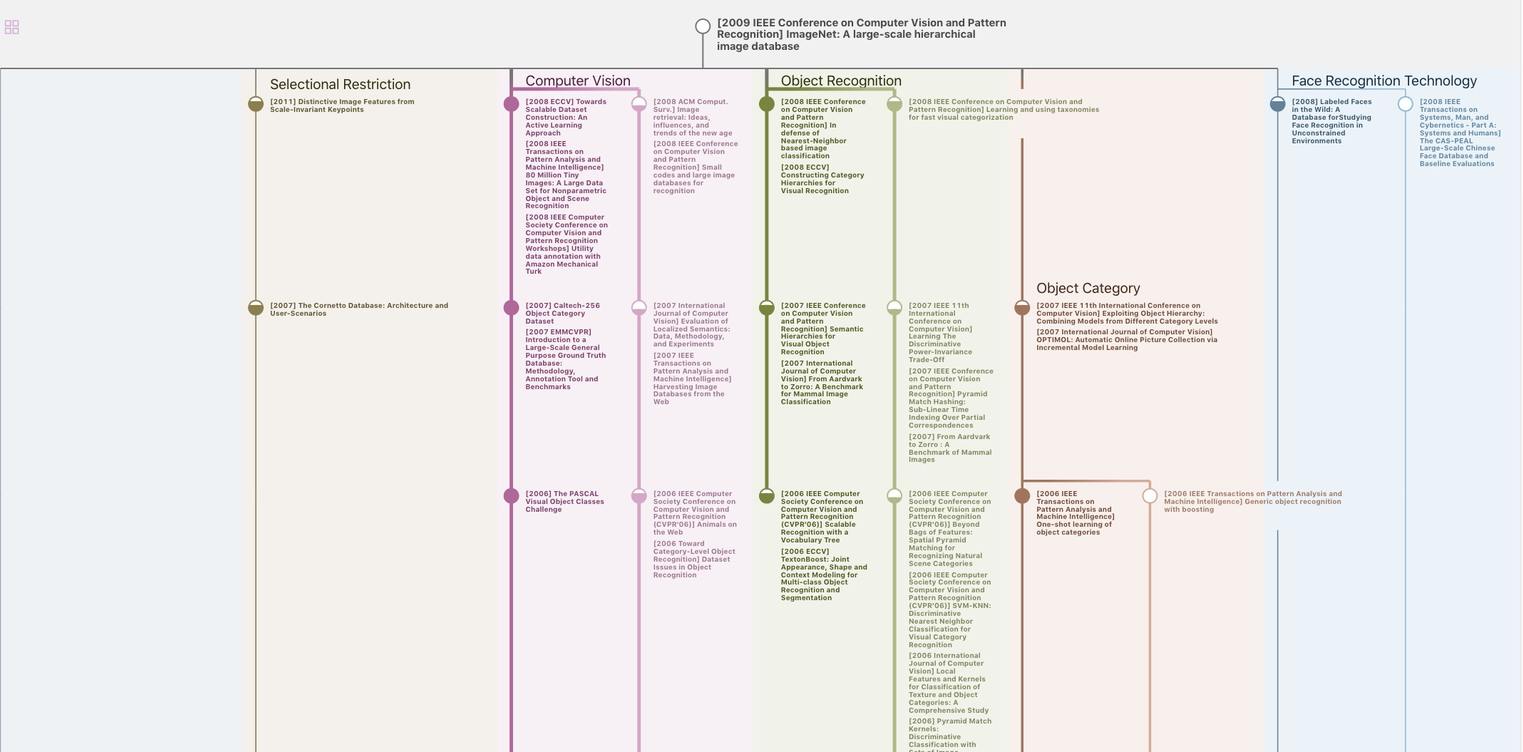
生成溯源树,研究论文发展脉络
Chat Paper
正在生成论文摘要