Granger Causal Inference for Interpretable Traffic Prediction.
International Conference on Intelligent Transportation Systems (ITSC)(2022)
摘要
Modeling spatial dependency is crucial to solving traffic prediction tasks; thus, spatial-temporal graph-based models have been widely used in this area in recent years. Existing approaches either rely on a fixed pre-defined graph (e.g., a road network) or learn the correlations between locations. However, most methods suffer from spurious correlation and do not sufficiently consider the traffic's causal relationships. This study proposes a Spatiotemporal Causal Graph Inference (ST-CGI) framework for traffic prediction tasks that learn both the causal graph and autoregressive processes. We decouple the spatiotemporal traffic prediction process into two steps; the causal graph inference step and the autoregressive step, where the latter relies on the former. Optimizing the entire framework on the autoregressive task approximates the Granger causality test and thus enables excellent interpretability of the prediction. Extensive experimentation using two real-world datasets demonstrates the outstanding performance of the proposed models.
更多查看译文
关键词
Granger Causal Inference,interpretable traffic prediction,modeling spatial dependency,traffic prediction tasks,spatial-temporal graph-based models,fixed pre-defined graph,spurious correlation,Spatiotemporal Causal Graph Inference framework,autoregressive processes,spatiotemporal traffic prediction process,autoregressive step,autoregressive task,Granger causality test
AI 理解论文
溯源树
样例
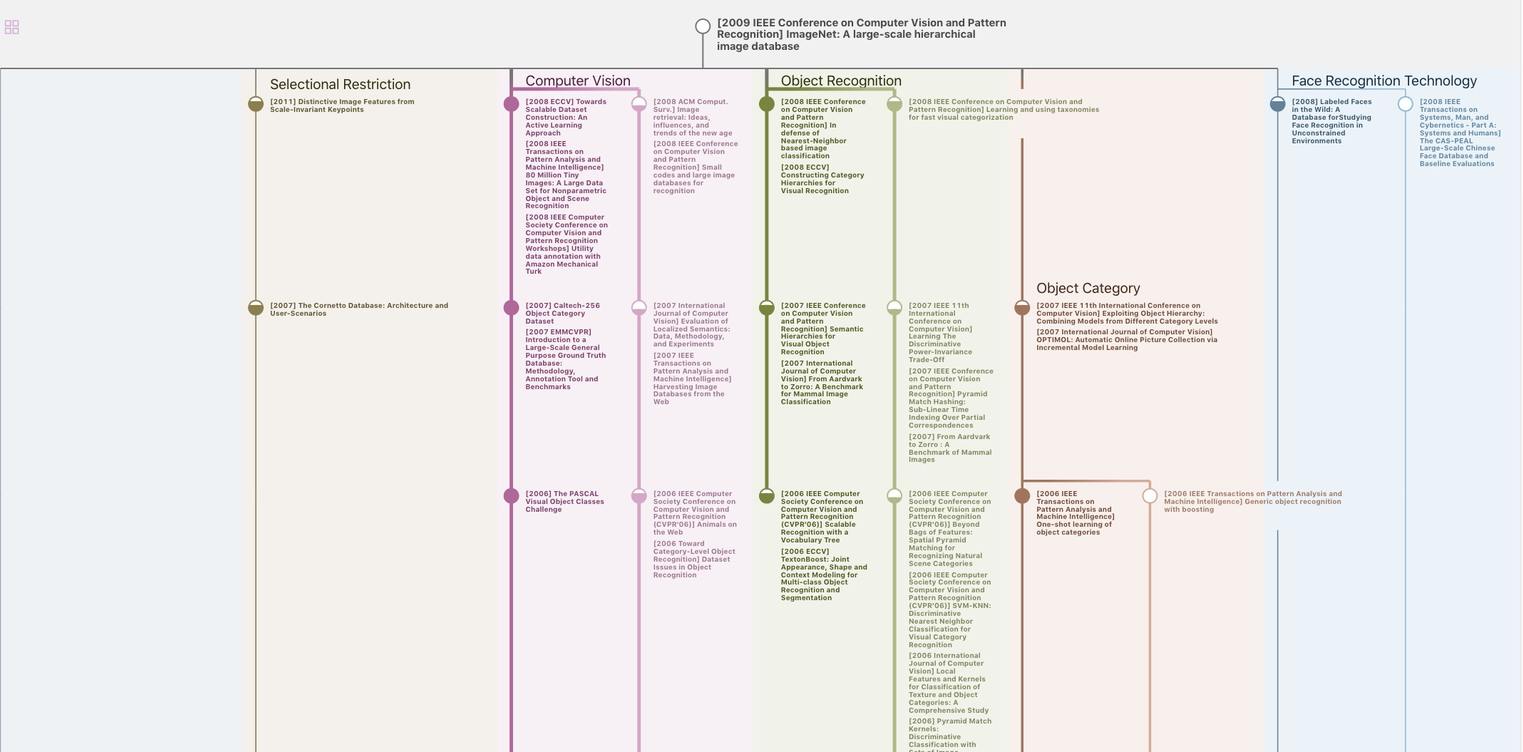
生成溯源树,研究论文发展脉络
Chat Paper
正在生成论文摘要