Surface Water Mapping in Sentinel-1 Images: A Probabilistic Approach Combining Classic Detection Methods.
IEEE International Geoscience and Remote Sensing Symposium (IGARSS)(2022)
摘要
Surface water mapping in satellite images enables flood monitoring, a task with increasing importance under changing climate conditions. Current segmentation methods based on Deep Learning require large, curated datasets for training, which are difficult to obtain. In this paper, we present a probabilistic approach to water segmentation based on established computer vision methods that requires little training and is easy to interpret. We use prior knowledge of typical backscatter intensity to locate seed pixels likely to be in water and land. A preliminary rough segmentation using thresholding guides the selection of two image patches that will be fully labeled using seeded region growing segmentation. Then, we sample small patches within the automatically labeled regions to train a fully connected neural network that, running in sliding windows, scores for the presence of water in the entire image. The approach is tested for mapping surface water during a large flood event in Aude, France. Outputs of the proposed approach are compared to the reference flood delineation map provided online by the Copernicus service. A F1 score of 0.67 suggests that performance of the proposed approach is similar or better than classic thresholding methods used as benchmark.
更多查看译文
关键词
Water mapping, floods, flood detection, image segmentation, computer vision
AI 理解论文
溯源树
样例
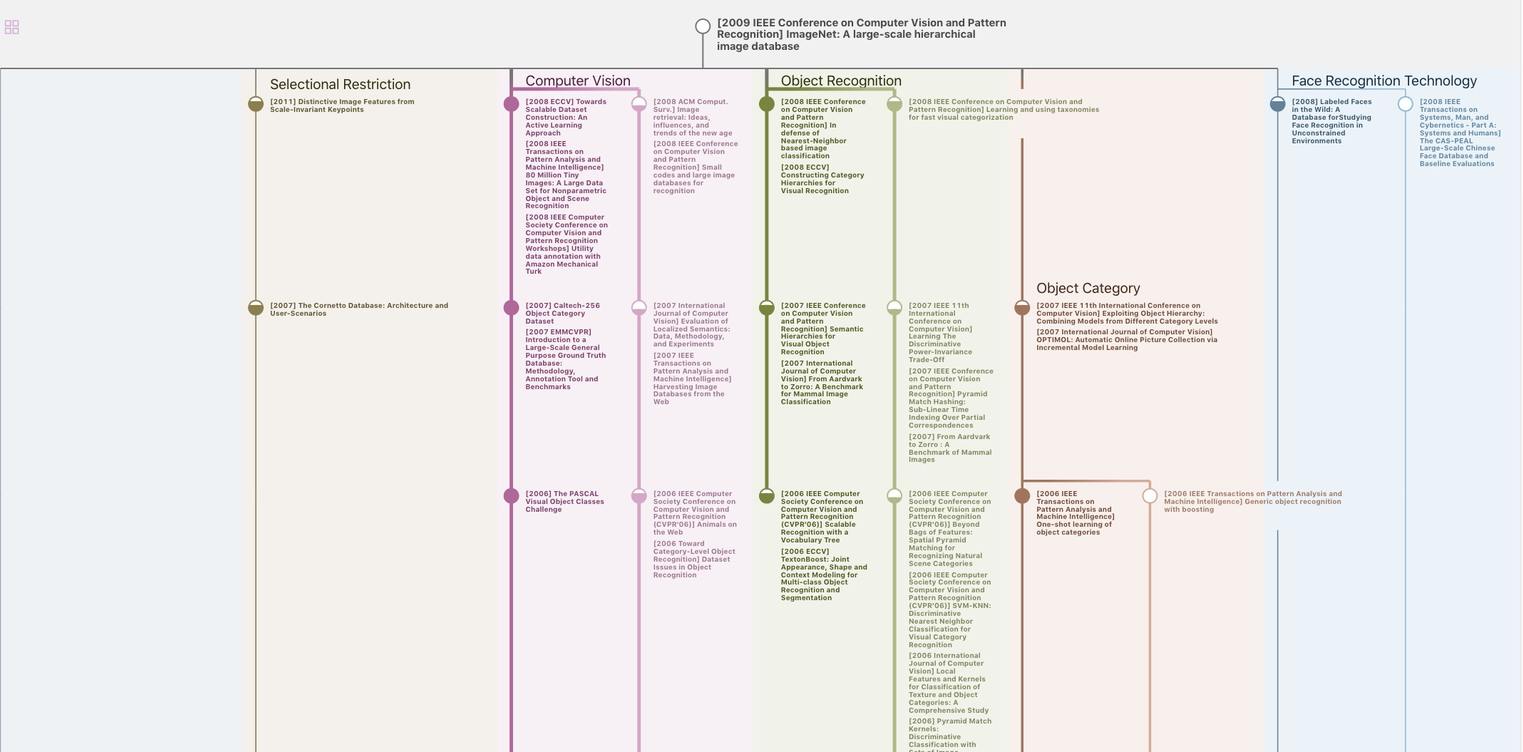
生成溯源树,研究论文发展脉络
Chat Paper
正在生成论文摘要