Spatial Graph Regularized Nonnegative Matrix Factorization for Hyperspectral Unmixing.
IEEE International Geoscience and Remote Sensing Symposium (IGARSS)(2022)
摘要
Hyperspectral unmixing is an important image interpretation technique that aims to estimate the pure constituent materials (endmembers) and their corresponding fractional abundances in each mixed pixel. Nonnegative matrix factorization (NMF) has attracted a lot of attention because of its ability to solve mixed pixel scenarios. The sparse NMF method achieves better unmixing results thanks to its full use of the sparse characteristics of the data. However, most existing sparse NMF unmixing techniques lack the consideration of spatial information. In fact, hyperspectral images contain intrinsic geometric information as well as rich spatial information. In this paper, a spatial graph regularized non-negative matrix factorization unmixing framework (SGNMF) is established. For the proposed SGNMF, on the one hand, the graph regularization is introduced to characterize the latent manifold structure of the data, and on the other hand, the spatial weighting factor is used to mine the spatial correlation between pixels. The optimization problem of the SGNMF model can be solved by a multiplicative iterative rule. Experimental results on synthetic data sets indicate that the newly proposed SGNMF method is able to produce better results than other advanced spectral unmixing algorithms.
更多查看译文
关键词
Hyperspectral Unmixing,NMF,graph regularization,spatially weighted unmixing
AI 理解论文
溯源树
样例
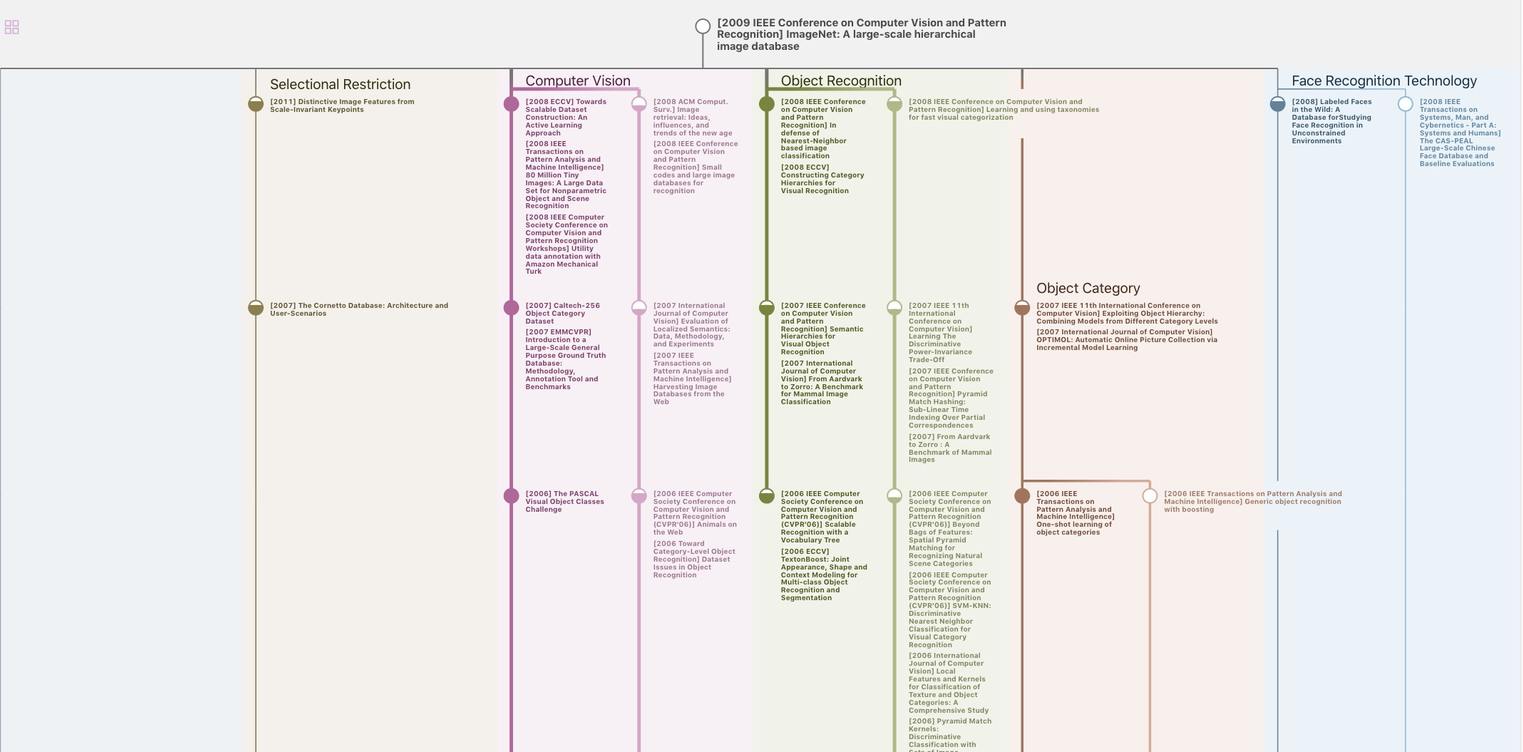
生成溯源树,研究论文发展脉络
Chat Paper
正在生成论文摘要