PointFP: A Feature-Preserving Point Cloud Sampling.
IEEE International Geoscience and Remote Sensing Symposium (IGARSS)(2022)
摘要
Many 3D perception applications (e.g., detection, classification, and segmentation) that directly process point cloud have made great progress with the development of 3D sensors in recent years. However, computational costs and storage demands of these applications grow significantly with the increase of point cloud size. Thus, it is necessary to sample the point cloud while preserving semantic features and uniform density. Deep learning-based sampling methods are task-specific and fail to generalize to different tasks. Hence, we propose a task agnostic point cloud simplification method, called point cloud feature-preserving. The proposed method preserves semantic features from the original point cloud by generating representative nodes. The sampling rate could be controlled by adjusting the number of representative nodes. Qualitative and quantitative experimental results on synthetic and real-world remote sensing point cloud datasets demonstrate the effectiveness of the proposed method.
更多查看译文
关键词
sampling,pointfp,pointfp,feature-preserving
AI 理解论文
溯源树
样例
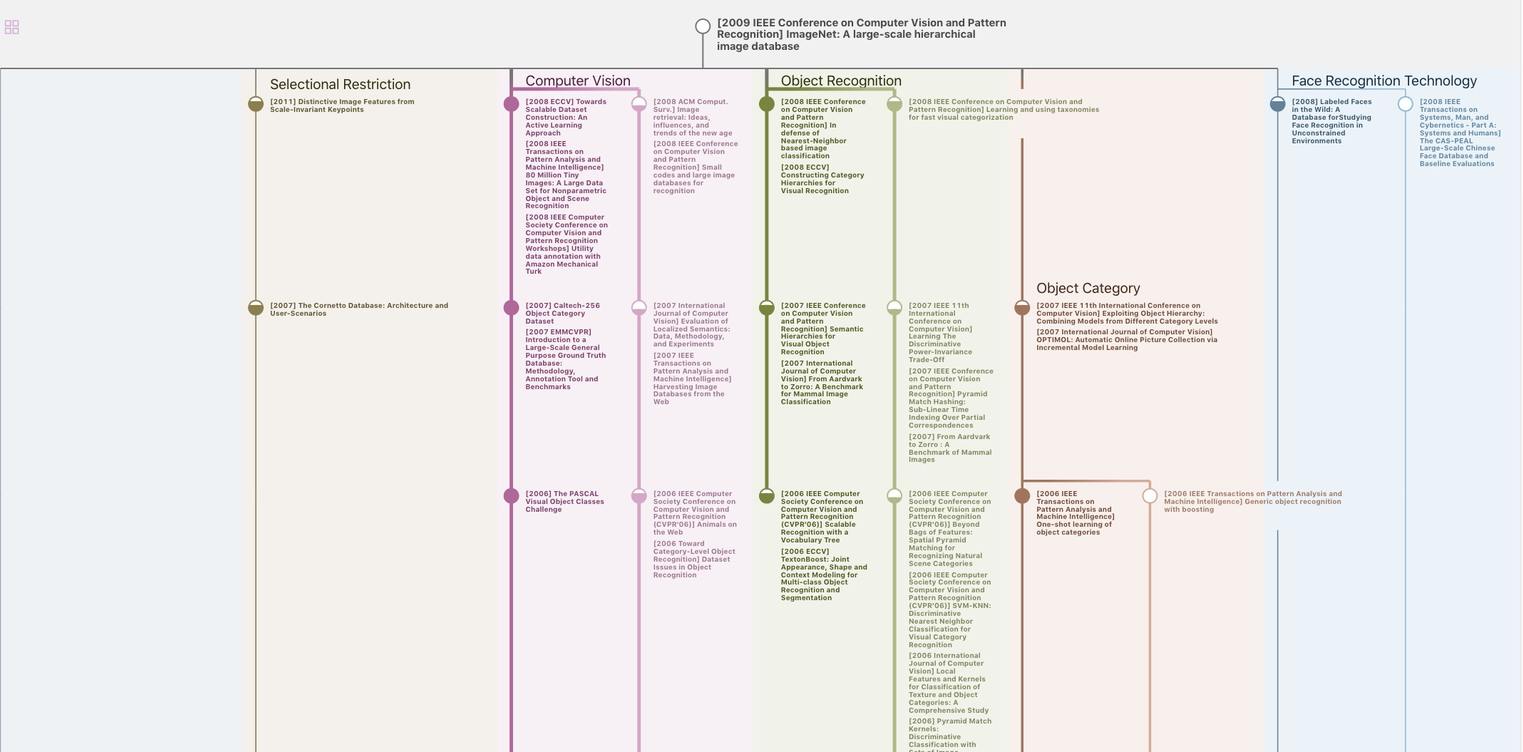
生成溯源树,研究论文发展脉络
Chat Paper
正在生成论文摘要