Label Aggregation with Clustering for Biased Crowdsourced Labeling.
International Conference on Machine Learning and Computing (ICMLC)(2022)
摘要
With the rapid development of crowdsourcing learning, amount of label aggregation methods are proposed to infer the true labels of instances from multiple noisy labels provided by inexpert crowd workers. Most of the label aggregation methods take the reliabilities of workers and the difficulties of instances into account and construct the probabilistic models, then infer the aggregated label and estimate the parameters simultaneously. However, to the best of our knowledge, label aggregation for biased crowdsourced labeling scenarios has not been sufficiently studied. Biased labeling is a critical factor that affects the performance of label aggregation and is hard to detect and model. To this end, this paper proposes a novel Label Aggregation with Clustering method for Biased Labeling (LACBL), to improve the quality of crowd labels by mitigating the labeling bias. LACBL detects the labeling bias of the dataset using clustering methods and then decreases the ratio of the biased class labels according to the bias. Finally, a label aggregation method is applied to the renewed label set. Experimental results on four real-world datasets show that LACBL outperforms other state-of-the-art label aggregation algorithms.
更多查看译文
AI 理解论文
溯源树
样例
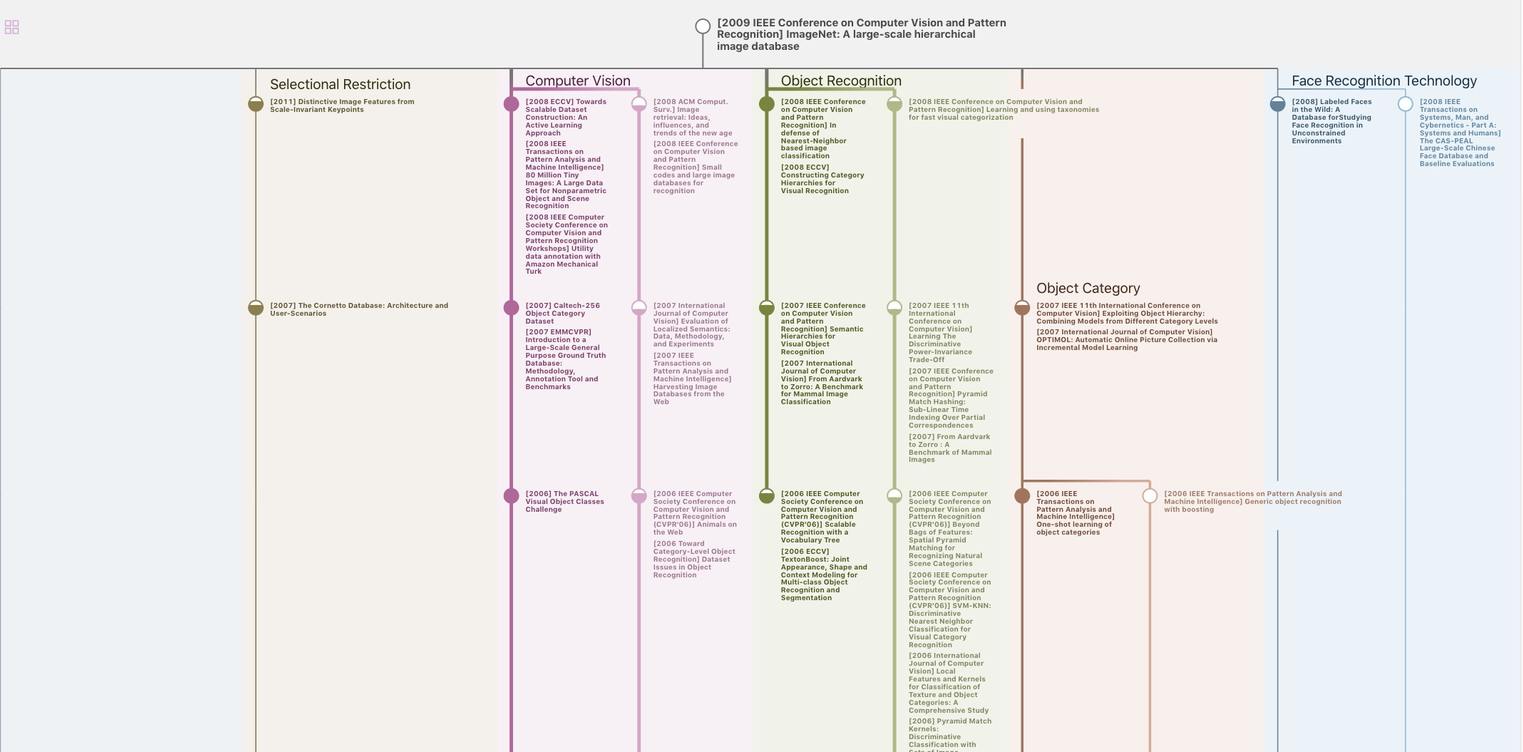
生成溯源树,研究论文发展脉络
Chat Paper
正在生成论文摘要