Weakly-supervised Salient Object Detection with Label Decoupling Siamese Network.
International Conference on Computing and Artificial Intelligence (ICCAI)(2022)
摘要
Weakly-supervised salient object detection (SOD) does not require a lot of manually annotated pixel-level labels. To further improve the detection accuracy of weakly-supervised SOD, we introduce a label decoupling siamese network (LDSN), which implements weakly-supervised SOD by learning from scribble labels. Scribble labels are novel weakly-supervised labels, which can reduce the cost of manual annotating and provide good interaction for users. In previous methods, the use of scribble labels mainly focuses on the salient object region. With the label decoupling siamese network, we make more adequately use of the scribble labels and the complementary relationship between salient object and background. We also propose a complementary self-supervised loss (CSS Loss) to realize a self-supervision. To improve the accuracy of the edge detection and interpretability of the network, we design a gradient priors weakly-supervised edge detection module (GWEM), which combines traditional image processing and deep learning methods. We compare the proposed network with 6 state-of-the-art SOD methods on 5 widely used datasets. The experiment results show that the performance of LDSN is superior to existing weakly-supervised SOD methods, and is compared with several pixel-level label supervised methods.
更多查看译文
AI 理解论文
溯源树
样例
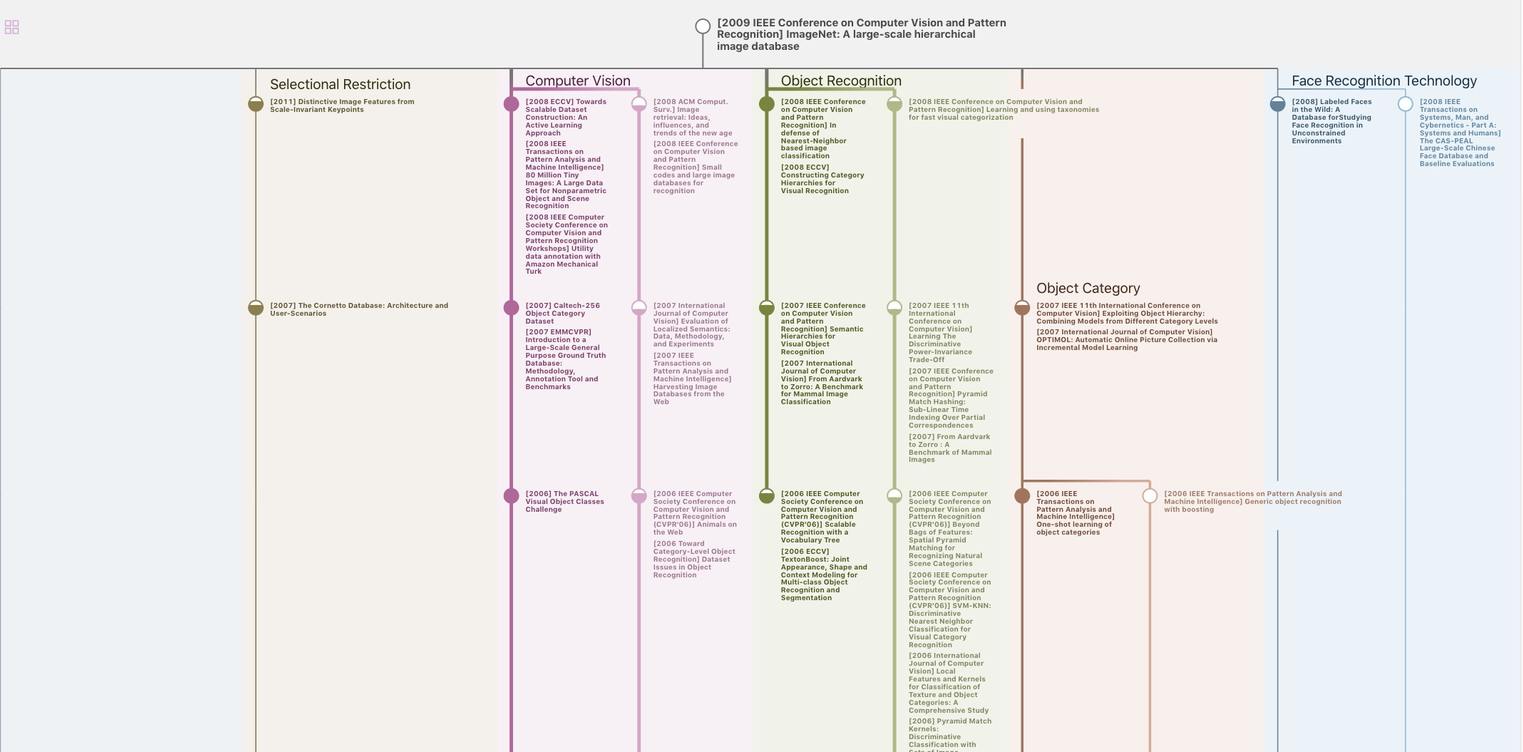
生成溯源树,研究论文发展脉络
Chat Paper
正在生成论文摘要