Time-varying Normalizing Flow for Generative Modeling of Dynamical Signals.
European Signal Processing Conference (EUSIPCO)(2022)
摘要
We develop a time-varying normalizing flow (TVNF) for explicit generative modeling of dynamical signals. Being explicit, it can generate samples of dynamical signals, and compute the likelihood of a (given) dynamical signal sample. In the proposed model, signal flow in the layers of the normalizing flow is a function of time, which is realized using an encoded representation that is the output of a recurrent neural network (RNN). Given a set of dynamical signals, the parameters of TVNF are learned according to maximum-likelihood approach in conjunction with gradient descent (backpropagation). Use of the proposed model is illustrated for a toy application scenario - maximum-likelihood based speech-phone classification task.
更多查看译文
关键词
normalizing flow,generative modeling,dynamical signals,time-varying
AI 理解论文
溯源树
样例
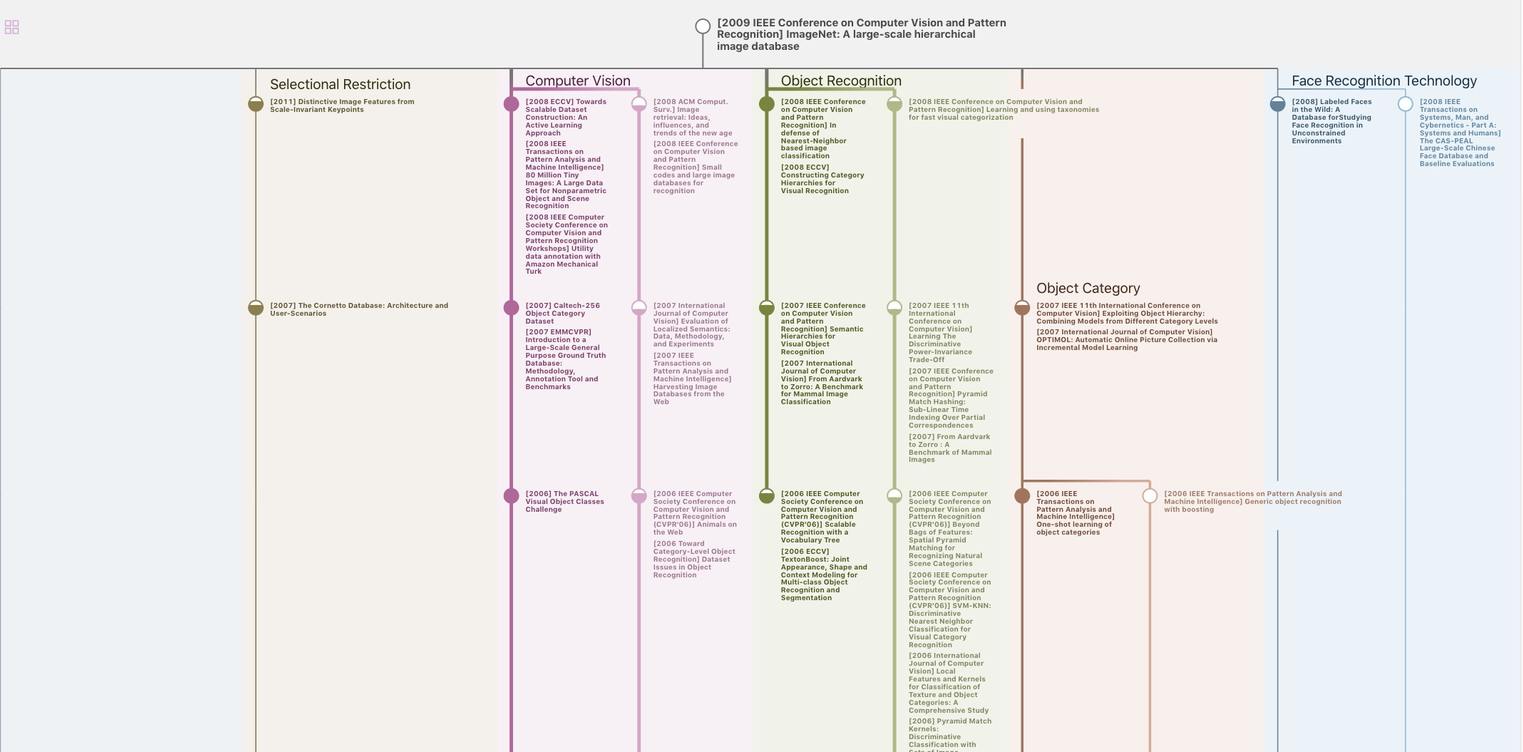
生成溯源树,研究论文发展脉络
Chat Paper
正在生成论文摘要