High-Dimensional Data Learning Based on Tensorial-Singular Space of Tensor Train Cores.
European Signal Processing Conference (EUSIPCO)(2022)
摘要
Tensors are multidimensional data structures used to represent many real world data. In the context of supervised learning, Support Vector Machines (SVMs) are known to be very efficient for different classification tasks. In this work, we propose a kernel metric for SVM to deal with non linear classification problems. First, we use the Tensor Train Decomposition (TTD) to decompose a tensor into TT-cores of order three and two matrices. In order to mitigate the problem of non-uniqueness of TTD, we propose a kernel based on the tensorial singular subspaces spanned by TT-cores. The TT-based kernel function proposed is based on the tools of t-Algebra of 3-rd order tensors. We also show that it is possible to use different kernel functions on each TT-core. Numerical experiments on real-world datasets show the competitivity of our approach compared to existing methods and the superiority of our method when dealing with few-sample of high-dimensional inputs.
更多查看译文
关键词
tensorial-singular,learning,high-dimensional,tensorial-singular
AI 理解论文
溯源树
样例
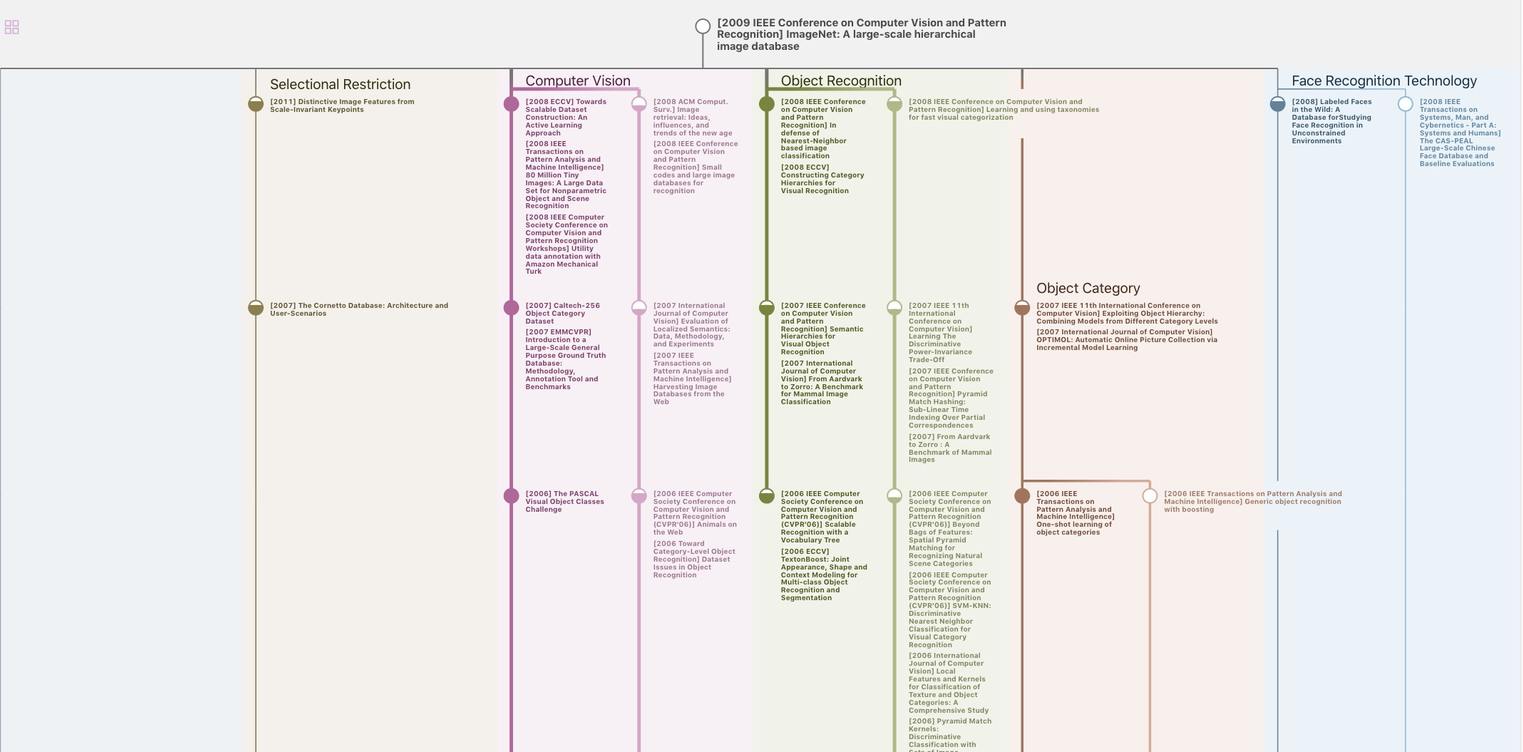
生成溯源树,研究论文发展脉络
Chat Paper
正在生成论文摘要