BACS: A comprehensive tool for deep learning-based anomaly detection in edge-fog-cloud systems.
European Signal Processing Conference (EUSIPCO)(2022)
摘要
The Internet of Things (IoT) technology has shown to offer promising benefits in various domains, including smart cities, smart factories, and smart manufacturing. However, the large number of heterogeneous devices deployed in such IoT systems increases the attack surface and hence poses additional security challenges. In this context, many of the relevant threat detection tasks can be formulated as deep learning (DL)-based detection of anomalies in the data acquired by the IoT system. There is hence a significant need for comprehensive anomaly detection (AD) tools in IoT applications. Indeed, as typical applications usually lack labeled data, multiple models need to be tried and compared one against another in order to get solid results. Moreover, the AD offerings should be adapted to the computational and storage capabilities of the underlying infrastructure for the given application. In this paper, we present BACS (Behavioral Analysis and Cognitive Security), a comprehensive DL-based tool for anomaly detection in IoT systems designed to be adaptable to edge-to-fog-to-cloud (E2F2C) environments. We provide an open source implementation of BACS, describe the internal architecture, explain how it is used by the developers, and illustrate its performance on real-world data provided by the Centro Ricerche Fiat (CRF) in the context of smart factory and smart logistics applications.
更多查看译文
关键词
Deep learning-based anomaly detection, edge-to-fog-to-cloud system, performance evaluation
AI 理解论文
溯源树
样例
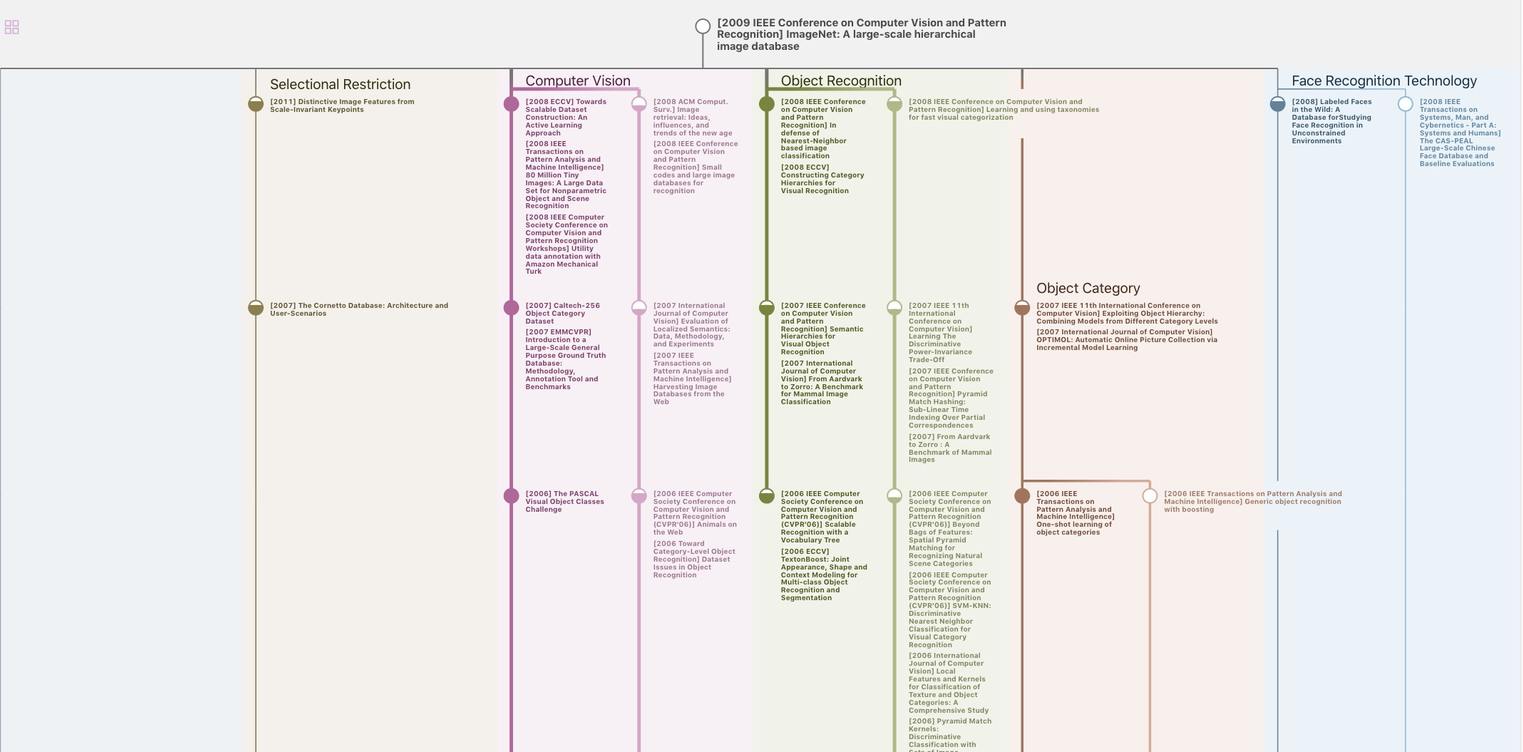
生成溯源树,研究论文发展脉络
Chat Paper
正在生成论文摘要