Learning-based Success Validation for Robotic Assembly Tasks
IEEE International Conference on Emerging Technologies and Factory Automation (ETFA)(2022)
摘要
The use of reinforcement learning for efficient robot programming has proven significant potential in research. Particularly in combination with advanced simulations, even complex assembly processes including variation and tolerances can be trained with little effort. However, reliable information about the system's current success state is needed to reward promising actions for training the reinforcement learning agent. While this success information is readily available in simulation or traditionally retrieved with rule-based approaches, a solution approach to infer the success state from available observation data would highly increase the robustness of the reward information and the subsequent transfer to reality. In this paper, we present a deep learning approach to learn the success criteria using the assembly benchmark process of peg-in-hole.
更多查看译文
关键词
Assembly Automation,Time Series Classification,Simulation
AI 理解论文
溯源树
样例
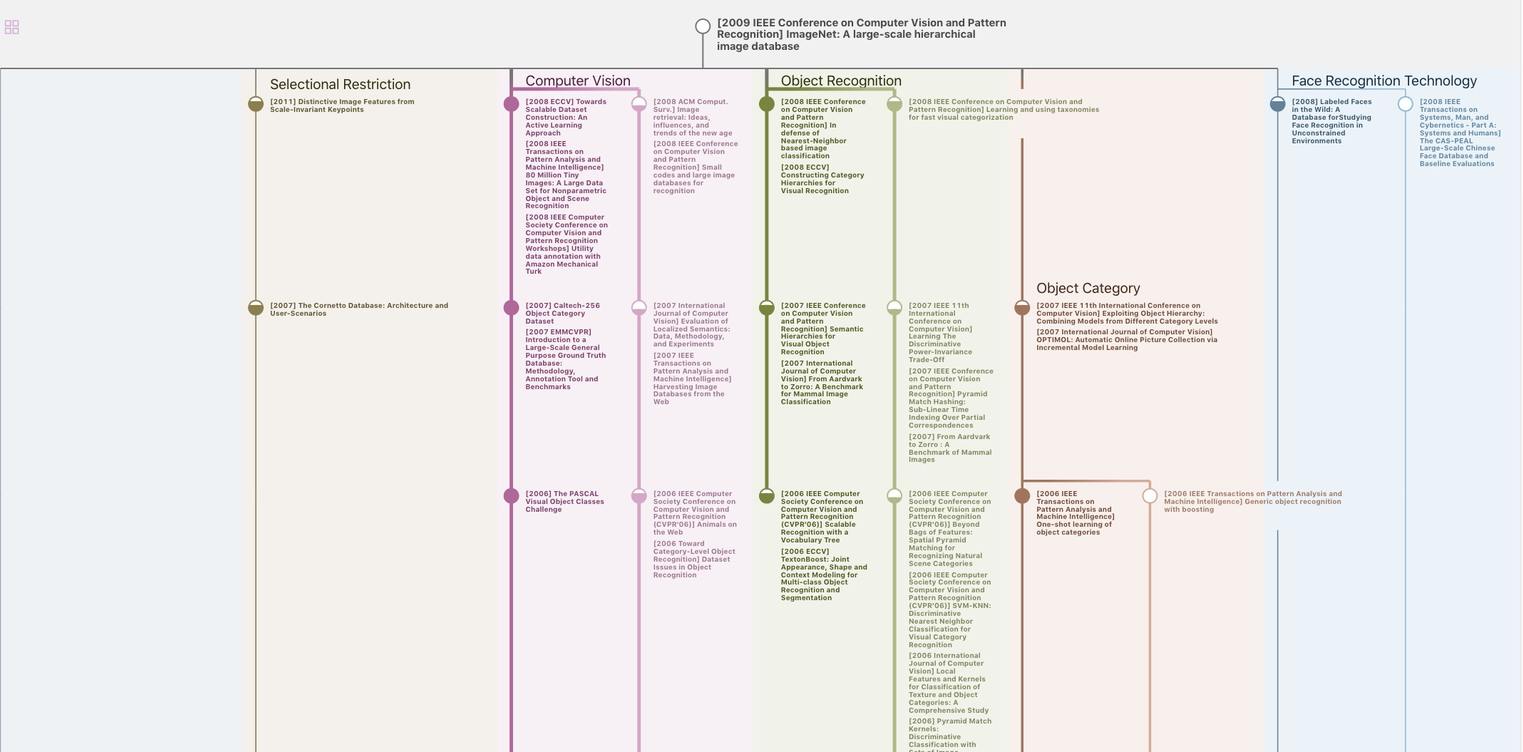
生成溯源树,研究论文发展脉络
Chat Paper
正在生成论文摘要