Improving Deepfake Detection by Mixing Top Solutions of the DFDC
European Signal Processing Conference (EUSIPCO)(2022)
摘要
The falsification of faces in videos is a growing phenomenon over the years. One of the most popular ways to tamper a face in a video is known as “deepfake”, Today, many tools exist to allow anyone to create a deepfake to discredit an individual or usurp an identity. Fortunately, the detection of deepfakes is an increasing topic of interest for the scientific community. As a result, many efforts have been made to develop mechanisms to automatically identify deepfake videos. In addition, several public deepfakes datasets have been built to help researchers to develop more effective detection methods. The most recent and also the most complete of these datasets is the one built by Facebook as part of the international DeepFake Detection Challenge (DFDC). Thousands of different frameworks, mainly based on deep learning, have been proposed during this challenge. The best solution that has been proposed obtains the accuracy of 82% on the DFDC dataset. However, the accuracy of this method is only 65% on unseen videos from the Internet. In this paper we analyse the five best methods of the DFDC and their complementarity. In addition, we experimented different assembly strategies (boosting, bagging and stacking) among these solutions. We show that we can achieve a large improvement
$(+ 41\%$
on log loss and
$+2.26\%$
on accuracy) when we carefully choose the models to be assembled with the most appropriate right merging method to use.
更多查看译文
关键词
deepfake detection, deepfake detection challenge, ensembling
AI 理解论文
溯源树
样例
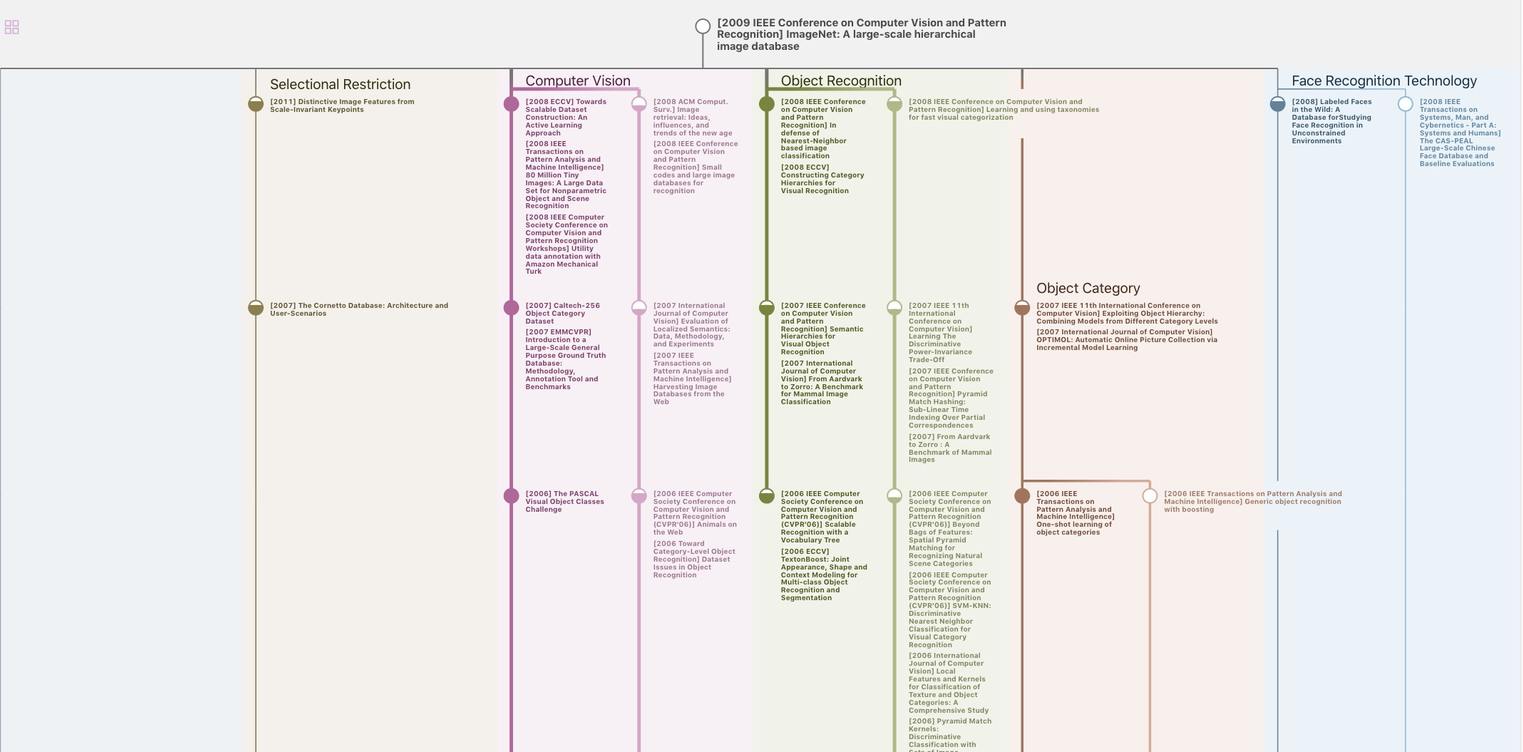
生成溯源树,研究论文发展脉络
Chat Paper
正在生成论文摘要