Time series-based deep learning model for personal thermal comfort prediction.
Energy-Efficient Computing and Networking (e-Energy)(2022)
摘要
Personal thermal comfort models are crucial for the future of human-in-the-loop HVAC control in energy-efficient buildings. Individual comfort models, compared to average population responses, can provide the personalization required for successful control. In this work, we frame the thermal preference prediction task as a multivariate, multi-class classification problem and use deep learning and time-series-based approach for thermal preference prediction. We combine l 1 regularization with a Regularized Long Short-Term Memory network (R-LSTM) to leverage the attentional mechanisms of such a model while counteracting overfitting. We run experiments on fourteen different subjects and find promising accuracy, F1 and AUC results, outperforming state-of-the-art machine learning approaches applied for the same task.
更多查看译文
AI 理解论文
溯源树
样例
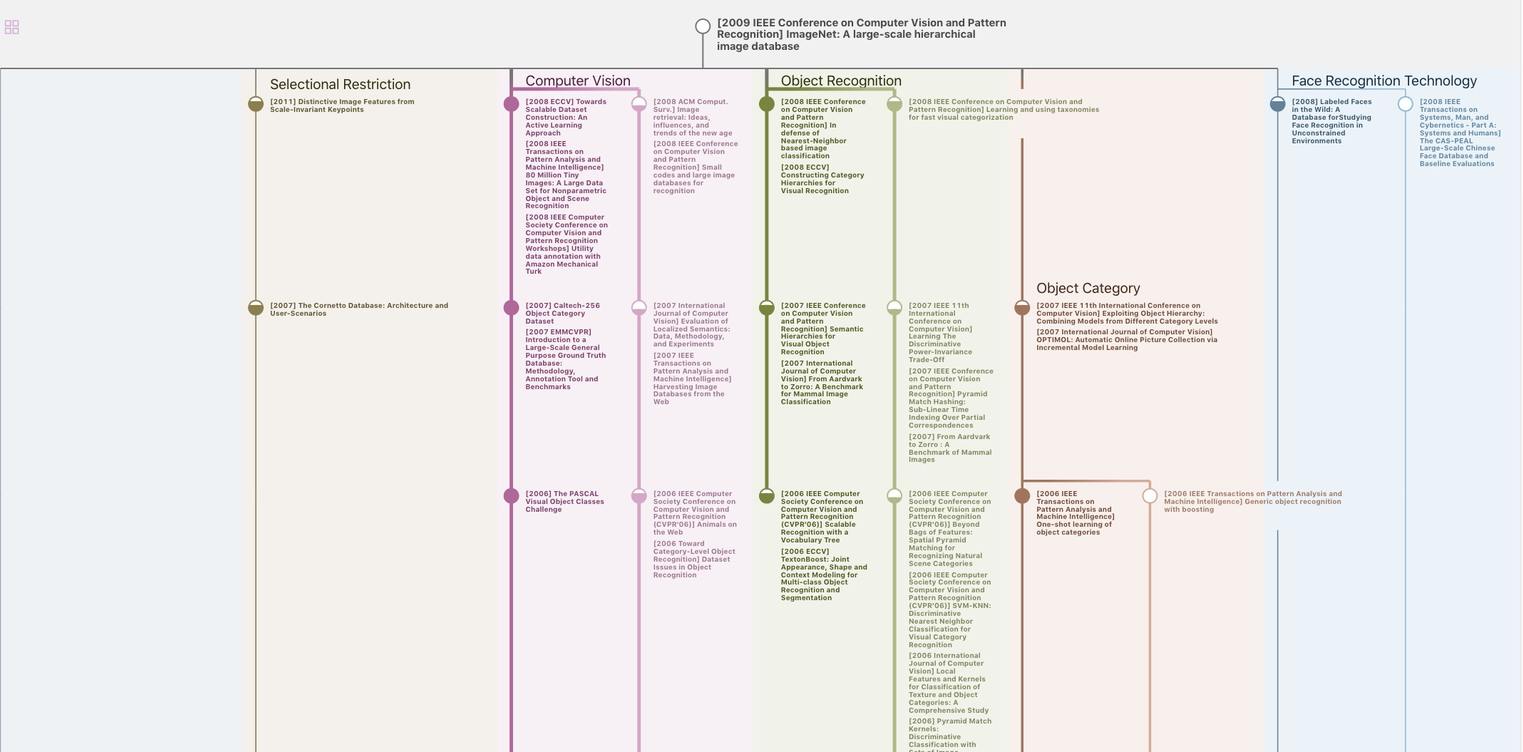
生成溯源树,研究论文发展脉络
Chat Paper
正在生成论文摘要