Multi-attribute Transformers for Sequence Prediction in Business Process Management.
International Conference on Discovery Science (DS)(2022)
摘要
Leveraging event logs to predict the evolution of an ongoing process is a challenging task in business process management (BPM). During the last years, sequence prediction models based on recurrent neural nets have demonstrated promise in this task attracting considerable interest from the community. Meanwhile, Transformer-based models and other architectures substituting recurrence with attention have become state-of-the-art in other sequence modeling tasks, especially in natural language processing. This paper investigates models based on the Transformer to predict operational business processes. In contrast to recent studies, we propose Multi-attribute Transformers, which exploit activities, resources, and time stamps for prediction, exploring different architectures to encode and integrate this information into the model. We also present multi-task variants of these models, which can predict the next activity of an ongoing process, when it will occur, and which resource it will trigger. Finally, we thoroughly evaluated these models in real datasets. In particular, we found that Multi-attribute Transformers can outperform Transformers that only use information about previous activities of the process. Moreover, our methods are competitive or better than existing multi-attribute recurrent models, allow significantly more parallelism during training and inference, and lead to more transparent/accountable predictions through the attention weights matrices.
更多查看译文
关键词
business process management,sequence prediction,multi-attribute
AI 理解论文
溯源树
样例
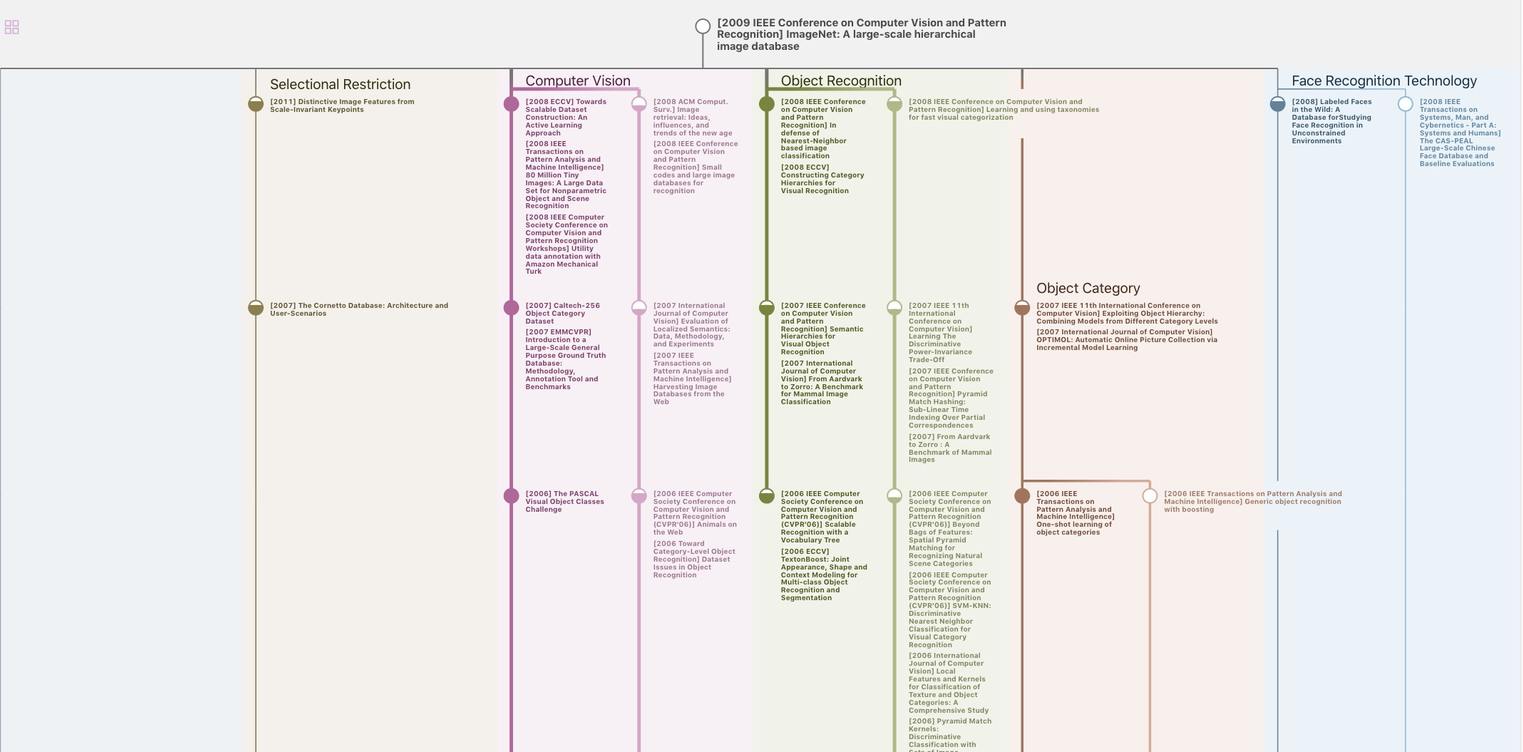
生成溯源树,研究论文发展脉络
Chat Paper
正在生成论文摘要