Policy Evaluation with Delayed, Aggregated Anonymous Feedback.
International Conference on Discovery Science (DS)(2022)
摘要
In reinforcement learning, an agent makes decisions to maximize rewards in an environment. Rewards are an integral part of the reinforcement learning as they guide the agent towards its learning objective. However, having consistent rewards can be infeasible in certain scenarios, due to either cost, the nature of the problem or other constraints. In this paper, we investigate the problem of delayed, aggregated, and anonymous rewards. We propose and analyze two strategies for conducting policy evaluation under cumulative periodic rewards, and study them by making use of simulation environments. Our findings indicate that both strategies can achieve similar sample efficiency as when we have consistent rewards.
更多查看译文
关键词
Reinforcement learning, Markov Decision Process (MDP), Reward estimation
AI 理解论文
溯源树
样例
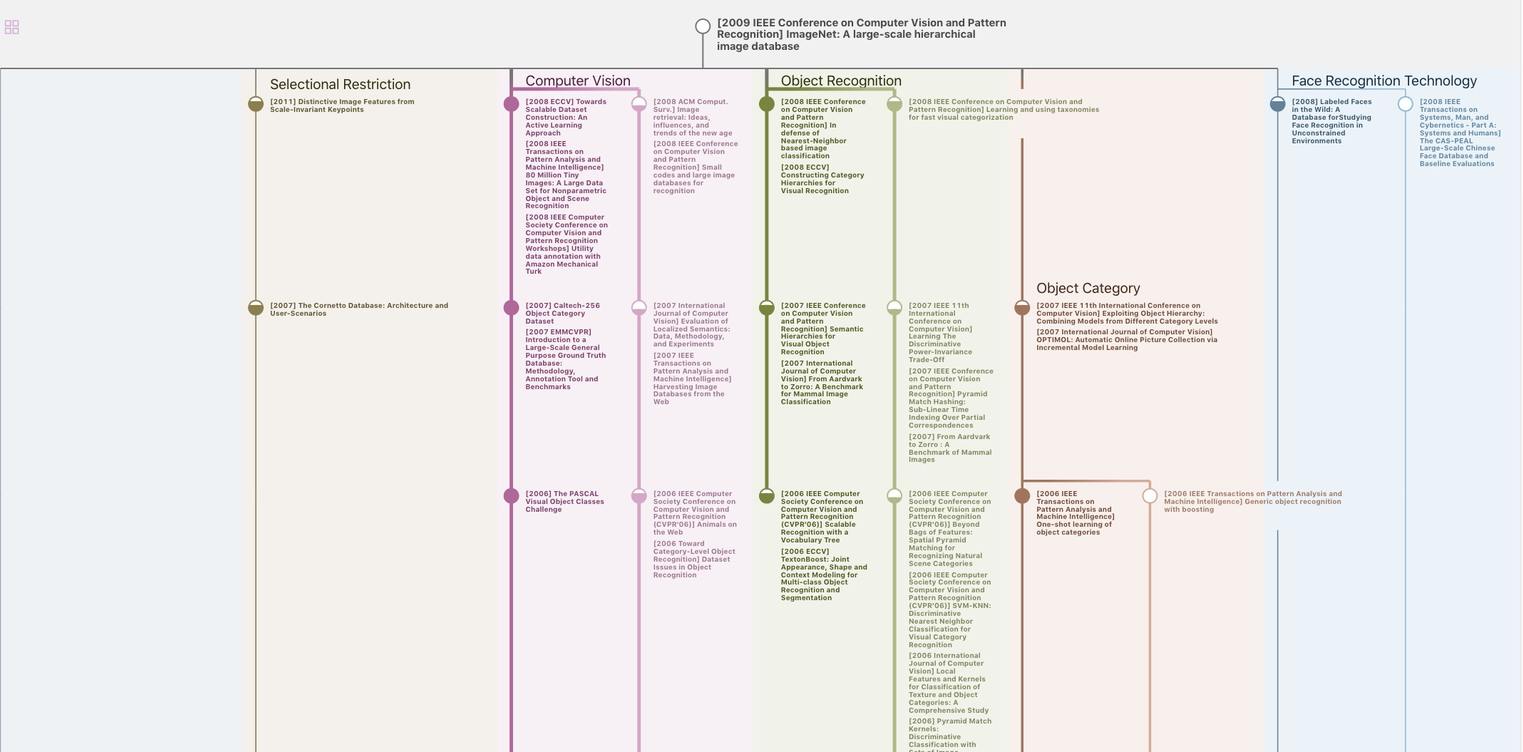
生成溯源树,研究论文发展脉络
Chat Paper
正在生成论文摘要