Towards Personalized Review Generation with Gated Multi-source Fusion Network.
International Conference on Database Systems for Advanced Applications (DASFAA)(2022)
摘要
Product review generation is an important task in recommender systems, which could provide explanation and persuasiveness for the recommendation. The key challenge of review generation is a lack of semantic information that can be used, resulting in more uncertainty in the generation processing. In this paper we propose a novel encoderdecoder personalized review generation model which aims to effectively and selectively fuse multiple resources including the user/product attributes and their textual information (e.g., historical reviews, short phrases). In the encoder part, we first design an attention layer to accordingly retrieve useful information from the users/product historical reviews since not all reviews are relevant to the target review generation. Note that the attention vector is derived from the semantic features of the short phrases (e.g., review summaries, product titles) which could indicate user preference and product characteristics. In the decoder part, we design a hierarchical gated multi-source network from both intra- and inter-gate perspectives to effectively fuse the multiple context features from encoders. Experimental results on the real-world dataset show that our proposed model could achieve better review generation performance than the baselines, in terms of fluency, readability, and personalization.
更多查看译文
关键词
Review generation,Recommender system,Multi-source gate
AI 理解论文
溯源树
样例
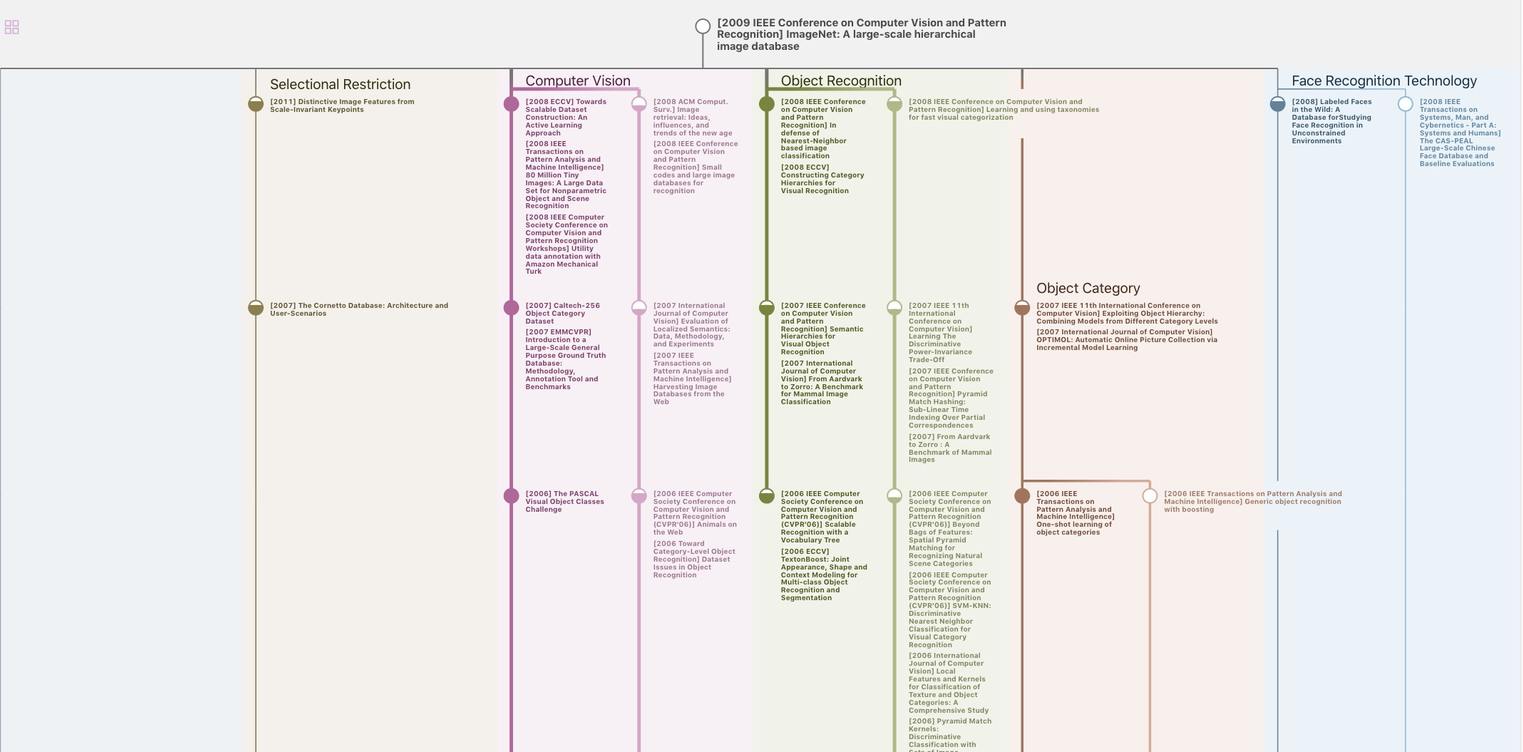
生成溯源树,研究论文发展脉络
Chat Paper
正在生成论文摘要