Traffic Volume Prediction with Automated Signal Performance Measures (ATSPM) Data.
International Symposium on Computers and Communications (ISCC)(2022)
摘要
Predicting short-term traffic volume is essential to improve transportation systems management and operations (TSMO) and the overall efficiency of traffic networks. The real-time data, collected from Internet of Things (IoT) devices, can be used to predict traffic volume. More specifically, the Automated Traffic Signal Performance Measures (ATSPM) data contain high-fidelity traffic data at multiple intersections and can reveal the spatio-temporal patterns of traffic volume for each signal. In this study, we have developed a machine learning-based approach using the data collected from ATSPM sensors of a corridor in Orlando, FL to predict future hourly traffic. The hourly predictions are calculated based on the previous six hours volume seen at the selected intersections. Additional factors that play an important role in traffic fluctuations include peak hours, day of the week, holidays, among others. Multiple machine learning models are applied to the dataset to determine the model with the best performance. Random Forest, XGBoost, and LSTM models show the best performance in predicting hourly traffic volumes.
更多查看译文
关键词
traffic volume prediction,automated signal performance measures,atspm
AI 理解论文
溯源树
样例
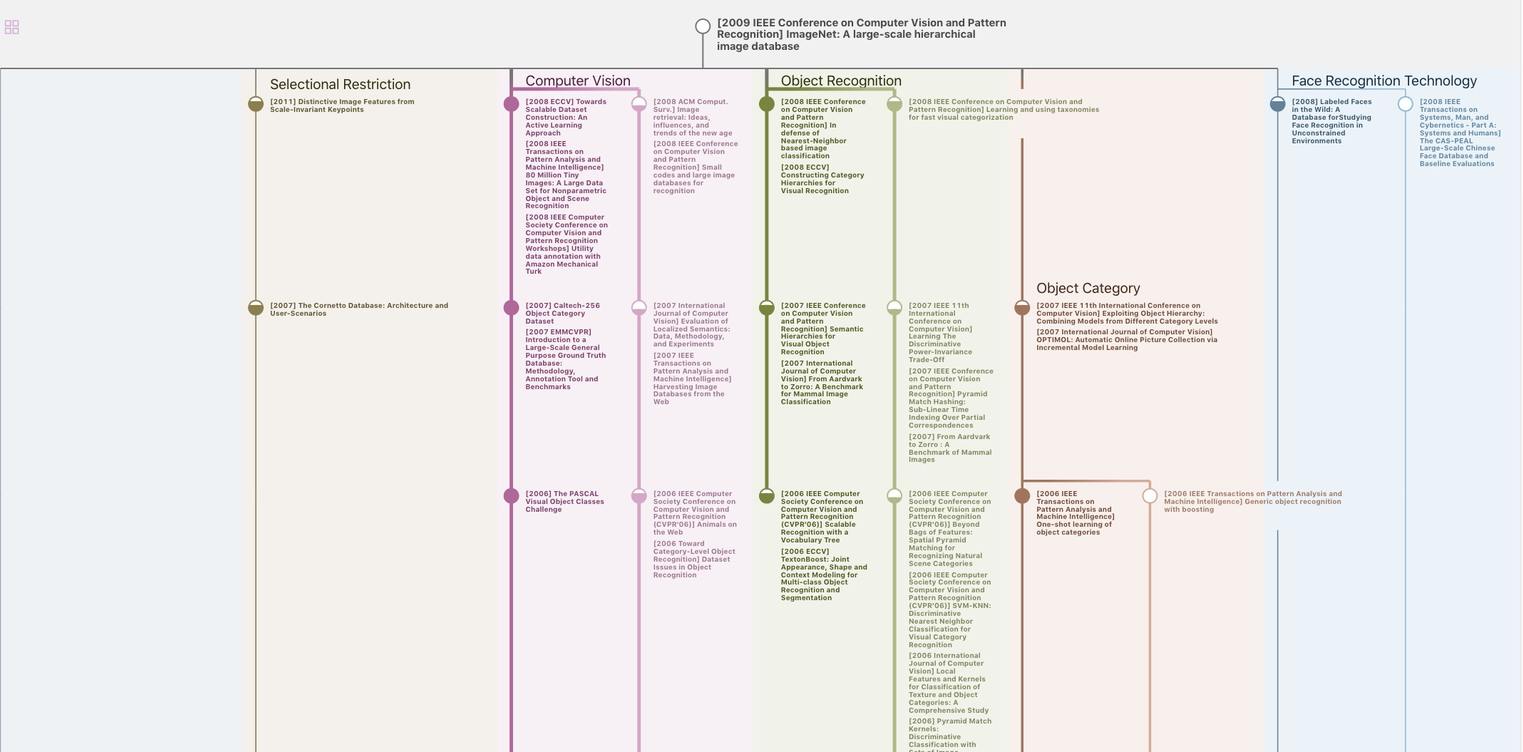
生成溯源树,研究论文发展脉络
Chat Paper
正在生成论文摘要