Global Patch Cross-Attention for Point Cloud Analysis.
Chinese Conference on Pattern Recognition and Computer Vision (PRCV)(2022)
摘要
Despite the great achievement on 3D point cloud analysis with deep learning methods, the insufficiency of contextual semantic description, and misidentification of confusing objects remain tricky problems. To address these challenges, we propose a novel approach, Global Patch Cross-Attention Network (GPCAN), to learn more discriminative point cloud features effectively. Specifically, a global patch construction module is developed to generate global patches which share holistic shape similarity but hold diversity in local structure. Then the local features are extracted from both the original point cloud and these global patches. Further, a transformer-style cross-attention module is designed to model cross-object relations, which are all point-pair attentions between the original point cloud and each global patch, for learning the context-dependent features of each global patch. In this way, our method can integrate the features of original point cloud with both the local features and global contexts in each global patch for enhancing the discriminative power of the model. Extensive experiments on challenging point cloud classification and part segmentation benchmarks verify that our GPCAN achieves the state-of-the-arts on both synthetic and real-world datasets.
更多查看译文
关键词
point cloud analysis,global,cross-attention
AI 理解论文
溯源树
样例
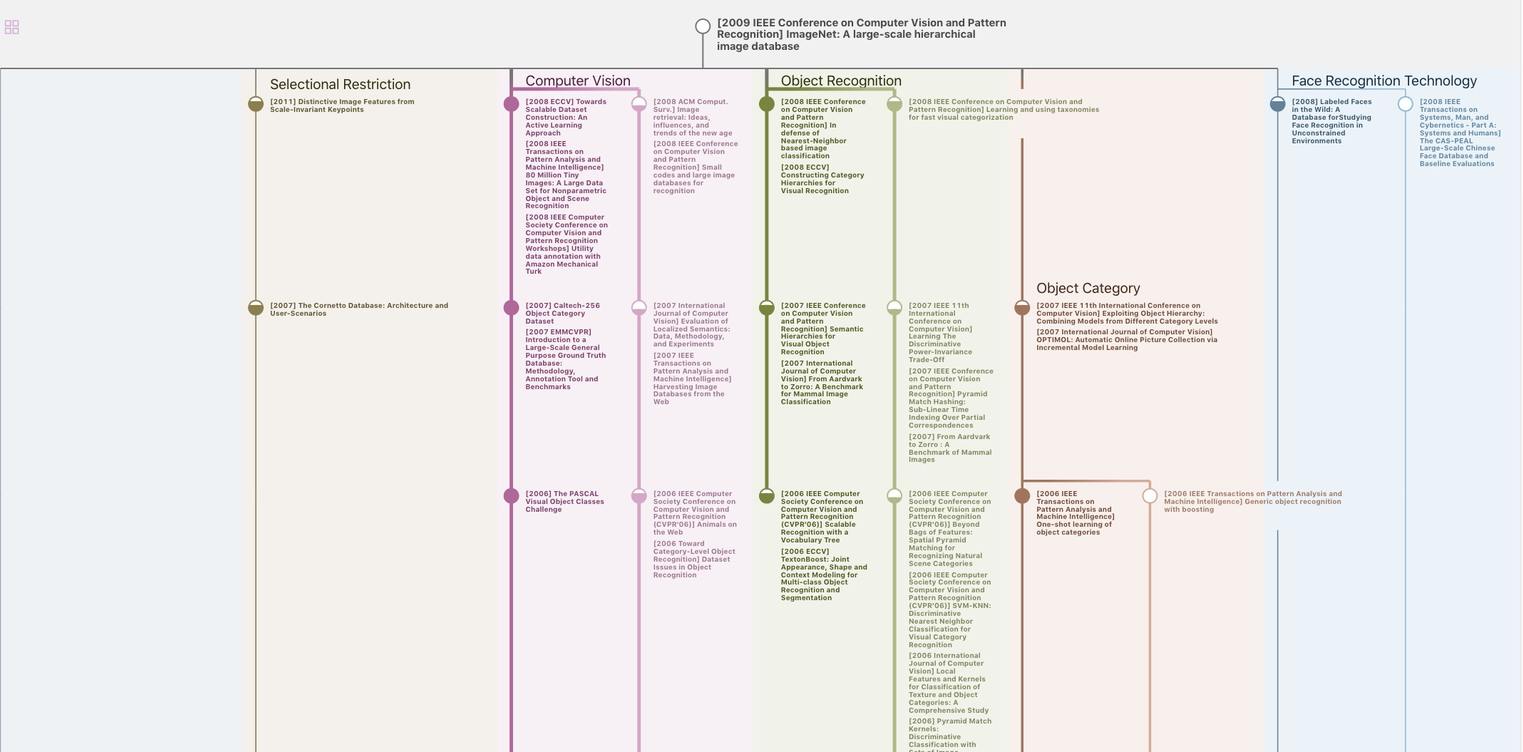
生成溯源树,研究论文发展脉络
Chat Paper
正在生成论文摘要