Interactive POI Recommendation: applying a Multi-Armed Bandit framework to characterise and create new models for this scenario.
Brazilian Symposium on Multimedia and the Web (WebMedia)(2022)
摘要
Nowadays, instead of the traditional batch paradigm where the system trains and predicts a model at scheduled times, new Recommender Systems (RSs) have become interactive models. In this case, the RS should continually recommend the most relevant item(s), receive the user feedback(s), and constantly update itself as a sequential decision model. Thus, the literature has modeled each recommender as a Multi-Armed Bandit (MAB) problem to select new arms (items) at each iteration. However, despite recent advances, MAB models have not yet been studied in some classical scenarios, such as the points-of-interest (POIs) recommendation. For this reason, this work intends to fill this scientific gap, adapting classical MAB algorithms for this context. This process is performed through an interactive recommendation framework called iRec. iRec provides three modules to prepare the dataset, create new recommendation agents, and simulate the interactive scenario. This framework contains several MAB state-of-the-art algorithms, a hyperparameter adjustment module, different evaluation metrics, different visual metaphors to present the results, and statistical validation. By instantiating and adapting iRec to our context, we can assess the quality of different interactive recommenders for the POI recommendation scenario.
更多查看译文
AI 理解论文
溯源树
样例
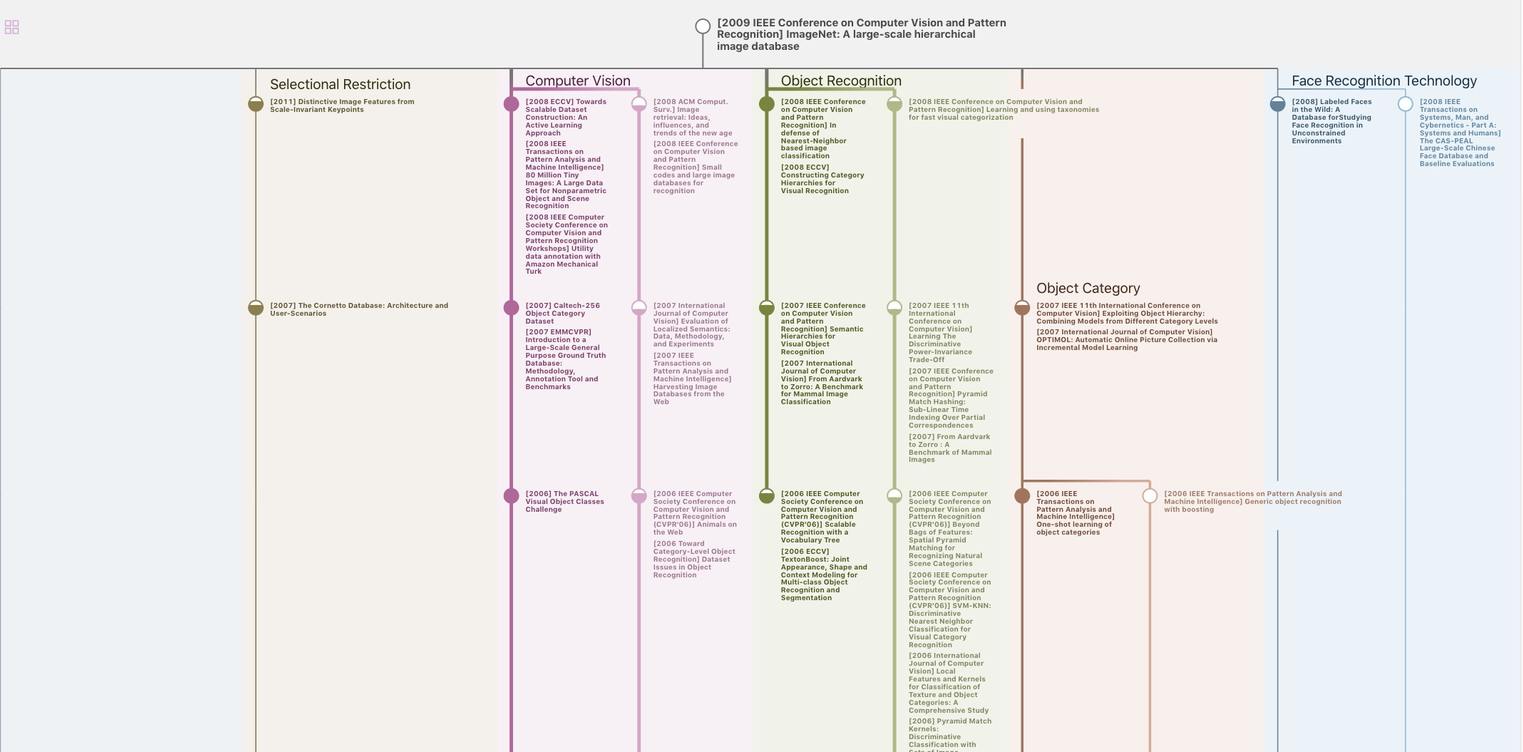
生成溯源树,研究论文发展脉络
Chat Paper
正在生成论文摘要